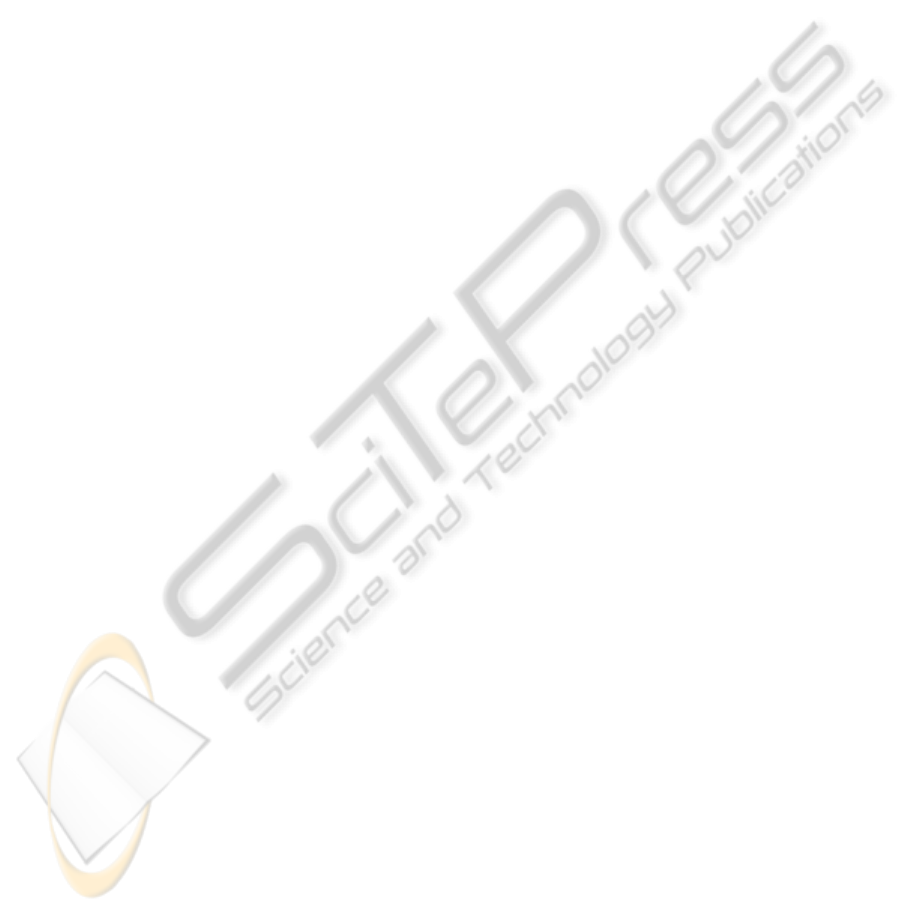
well. The strategy holds positions based upon a com-
parison of the returns of two ETFs, one scaled by
an estimate of β
rsp
,t
. Apparently small variation in
the estimates of the regression parameter are not of
large consequence. Given the trading rule is based
on the sign of the error ε
t
, it appears that on many
days, slight variation in the estimate of θ
t
across
DLMs does not result in a change to
sign
(ε
t
). Fig-
ure 8 shows that over the interval studied, the mixture
model provided a higher return per unit of risk, if only
to a modest extent. What is worth mentioning is that
the comparison we make is the on-line mixture model
against the ex post best performance of all constant
parameter models. Acknowledging this distinction,
the mixture model’s performance is more impressive.
6 CONCLUSIONS
Mixtures of dynamic linear models are a useful tech-
nology for modeling time series data. We show the
ability of DLMs parameterized with time varying val-
ues to generate observations for complex dynamic
processes. Using a mixture of DLMs, we extract time
varying parameter estimates that offered insight to the
returns process of the S&P 500 ETF during the finan-
cial crisis of 2008. Our on-line mixture model demon-
strated superior performance compared to the ex post
optimal component DLM in a statistical arbitrage ap-
plication.
The contributions of this paper include the pro-
posal of a method, trailing interval likelihood, for
constructing component model prior probabilities.
This technique facilitated successful modeling of time
varying observational and evolution variance parame-
ters, and captured model evidence not adequately con-
veyed in the one-step forecast distribution due to scal-
ing issues. We proposed the use of two widely avail-
able time-series to facilitate easier replication and
extension of the statistical arbitrage application pro-
posed by (Montana et al., 2009). Our addition of
a hedge to the statistical arbitrage application from
(Montana et al., 2009) resulted in dramatically im-
proved Sharpe ratios.
We have only scratched the surface of the mod-
eling possibilities with DLMs. The mixture model
technique eliminates the burden of a priori specifica-
tion of process parameters. We look forward to evalu-
ating models with higher dimension state vectors and
parameterized evolution matrices. Due to the inher-
ently parallel nature of DLM mixtures, we also look
forward to exploring the ability of current hardware
to tackle additional challenging modeling problems.
REFERENCES
Bar-Shalom, Y., Li, X., Kirubarajan, T., and Wiley, J.
(2001). Estimation with applications to tracking and
navigation. John Wiley & Sons, Inc.
Bishop, C. (2006). Pattern Recognition and Ma-
chine Learning (Information Science and Statistics).
Springer Science+Business Media, LLC. New York,
NY, USA.
Chen, R. and Liu, J. (2000). Mixture Kalman filters. Jour-
nal of the Royal Statistical Society: Series B (Statisti-
cal Methodology), 62(3):493–508.
Crassidis, J. and Cheng, Y. (2007). Generalized Multiple-
Model Adaptive Estimation Using an Autocorrelation
Approach. In Information Fusion, 2006 9th Interna-
tional Conference on, pages 1–8. IEEE.
Ghahramani, Z. and Hinton, G. (1996). Parameter estima-
tion for linear dynamical systems. Technical Report
CRG-TR-96-2, University of Toronto.
Hamilton, J. (1994). Time series analysis. Princeton Uni-
versity Press: Princeton, NJ, USA.
Johnson, R. and Wichern, D. (2002). Applied Multivari-
ate Statistical Analysis. Prentice Hall: Upper Saddle
River, NJ, USA.
Kalaba, R. and Tesfatsion, L. (1996). A multicriteria ap-
proach to model specification and estimation. Com-
putational Statistics & Data Analysis, 21(2):193–214.
Kalman, R. et al. (1960). A new approach to linear filtering
and prediction problems. Journal of Basic Engineer-
ing, 82(1):35–45.
Minka, T. (1999). From hidden Markov models to linear
dynamical systems. Technical Report 531, Vision and
Modeling Group of Media Lab, MIT.
Minka, T.P. (2007). Bayesian inference in dynamic models:
an overview. http://research.microsoft.com.
Montana, G., Triantafyllopoulos, K., and Tsagaris, T.
(2009). Flexible least squares for temporal data min-
ing and statistical arbitrage. Expert Systems with Ap-
plications, 36(2):2819–2830.
PDR Services LLC (2010). Prospectus. SPDR S&P 500
ETF. https://www.spdrs.com.
Rydex Distributors, LLC (2010). Prospectus. Rydex S&P
Equal Weight ETF. http://www.rydex-sgi.com/.
Sarkka, S. and Nummenmaa, A. (2009). Recursive noise
adaptive Kalman filtering by variational Bayesian ap-
proximations. Automatic Control, IEEE Transactions
on, 54(3):596–600.
Valpola, H., Harva, M., and Karhunen, J. (2004). Hierar-
chical models of variance sources. Signal Processing,
84(2):267–282.
West, M. and Harrison, J. (1997). Bayesian Forecasting
and Dynamic Models. Springer-Verlag New York, Inc.
New York, NY, USA.
Yelland, P. and Lee, E. (2003). Forecasting product sales
with dynamic linear mixture models. Technical Re-
port SMLI TR-2003-122, Sun Microsystems, Inc.
ICPRAM 2012 - International Conference on Pattern Recognition Applications and Methods
302