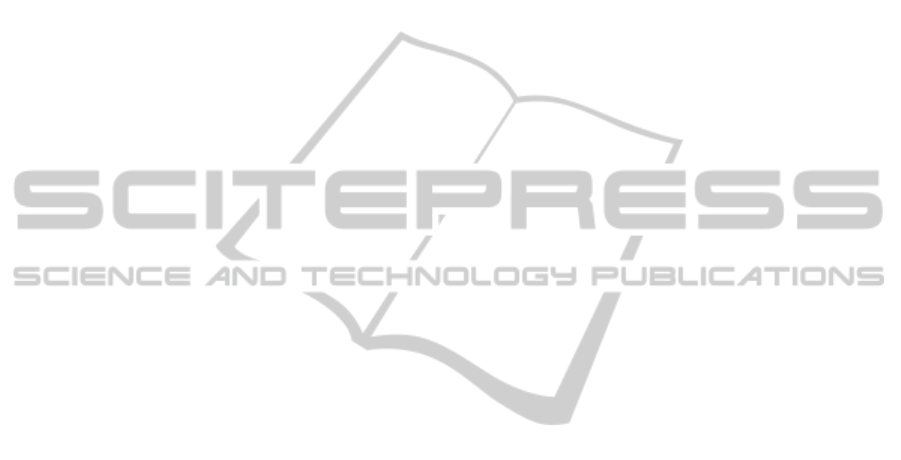
4.3 Application in Portfolio
Optimization
In principle, the concept of PFVaR can also be used
in a portfolio optimization context. Technically, it is
quite simple to generalize traditional risk-return op-
timization approaches to the newly suggested PFVaR
measures. However, although the replacement of or-
dinary risk measures like VaR by PFVaR measures
looks quite simple on first glance, it has to be noted
that the interpretation changes quite significantly: In
traditional approaches, the expected return from time
t
R
to t
R
+ h, i.e. E[V
t
R
+h
− V
t
R
], is compared against
a risk measure applied to the profit-and-loss distribu-
tion of the net asset valuesV
t
R
+h
−V
t
R
(here, h denotes
the time horizon of the risk measure). If the risk mea-
sure is now replaced by a potential future risk mea-
sure, then the risk of the profit-and-loss distribution of
V
T+h
−V
T
for some future time T is compared against
the return from time t
R
to T (or T +h), which does not
represent a meaningful setup.
Instead, it is much more meaningful to introduce
additional constraints based on PFVaR measures in
traditional portfolio optimization. For example, one
might consider the situation that the expected re-
turn from time t
R
to T should be maximized (usually
T − t
R
represents one year), while risk capital – rep-
resented by the VaR of the profit-and-loss distribution
of V
T
−V
t
R
– is limited by the available capital of the
bank. In such a case, it would be meaningful to intro-
duce additional PFVaR constraints for smaller time
horizons, which would guarantee that a short term
VaR on a time horizon of h does not exceed a certain
much smaller limit throughout the whole time period
from t
R
to T.
5 RESUMEE
We have shown that VaR figures cannot be expectedto
be constant, but may vary overtime due to severalrea-
sons, such as changes in covariance parameters and
sensitivities. We have further observed that all effects
might be similarly important and need to be taken into
account. As a remedy against model risk arising from
these changes, the new concept of potential future
market risk has been introduced and motivated and
it has been argued how this can be incorporated into
a bank’s planning cycle. Although PFVaR numbers
themselves depend on the simulated risk factors, i.e. a
certain dependence on models and assumptions is al-
ways inherent, we have illustrated that this concept
has several powerful applications, especially in new
business planning and economic capital buffer plan-
ning. Still, a careful selection of the simulation as-
sumptions is key to a successful application of the PF-
VaR concept. Eventually, we believe that the PFVaR
concept can also be successfully applied in a portfo-
lio optimization context, but we leave this for future
research, as this needs much more detailed technical
considerations.
REFERENCES
Alexander, C. (2001). Market Models – A Guide to Finan-
cial Data Analysis. John Wiley & Sons, Chicago.
Alexander, C. and Sarabia, J. M. (2011). Value-at-Risk
Model Risk. SSRN eLibrary.
Bams, D. and Wielhouwer, J. L. (2001). Empirical Issues
in Value-At-Risk. Astin Bulletin, 31(2):299–315.
Berkowitz, J., Christoffersen, P., and Pelletier, D. (2011).
Evaluating Value-at-Risk models with desk-level data.
Management Science.
Berkowitz, J. and OBrien, J. (2002). How accurate are
Value-at-Risk models at commercial banks? Journal
of Finance, (55):1093–1111.
Christoffersen, P. and Goncalves, S. (2005). Estimation
Risk in Financial Risk Management. Journal of Risk,
7(3):1–28.
Christoffersen, P., Hahn, J., and Inoue, A. (2001). Testing
and comparing Value-at-Risk measures. Journal of
Empirical Finance, (8):325–342.
Christoffersen, P. and Pelletier, D. (2004). Backtesting
Value-at-Risk: a duration-based approach. Journal of
Financial Econometrics, 1(2):84–108.
Danielsson, J. (2002). The Emperor has no Clothes: Limits
to Risk Modelling. Journal of Banking and Finance,
26:1273–1296.
Davidson, C. (2008). The best scenario. Risk Magazine,
June.
Figlewski, S. (2003). Estimation Error in the Assessment of
Financial Risk Exposure. Working Paper.
Hendricks, D. (1996). Evaluation of Value-at-Risk Mod-
els Using Historical Data. Economic Policy Review,
Federal Reserve Bank of New York, April:39–69.
Jorion, P. (1996). Risk
2
: Measuring the Risk in Value at
Risk. Financial Analysts Journal, Nov/Dec:47–56.
Jorion, P. (2007). Value At Risk: The New Benchmark for
Managing Financial Risk. McGraw-Hill, New York,
3rd edition.
Kamdem, J. S. (2005). Value-At-Risk And Expected Short-
fall For Linear Portfolios With Elliptically Distributed
Risk Factors. International Journal of Theoretical and
Applied Finance, 8(5):537–551.
Kerkhof, J., Melenberg, B., and Schumacher, H. (2010).
Model risk and capital reserves. Journal of Banking
and Finance, (34):267–279.
Kupiec, P. (1995). Techniques for Verifying the Accuracy
of Risk Measurement Models. Journal of Derivatives,
3:73–84.
Pykhtin, M. (2005). Counterparty Credit Risk Modelling.
Risk Books, London.
COPING WITH LONG TERM MODEL RISK IN MARKET RISK MODELS
245