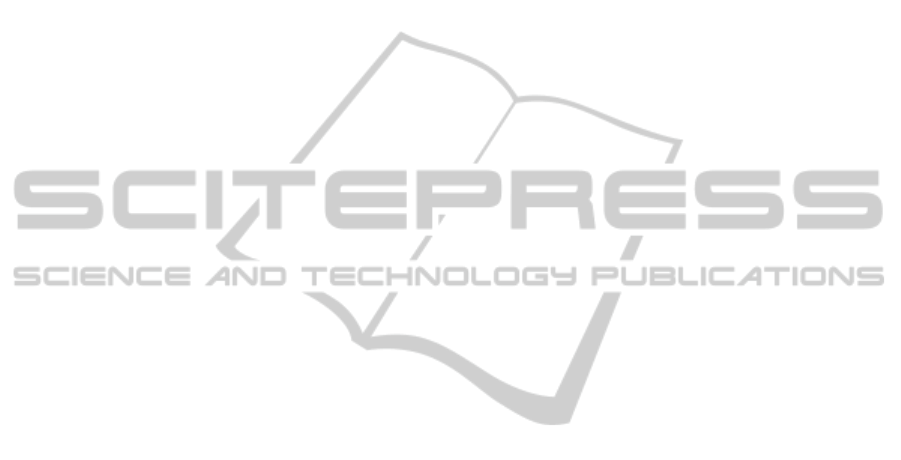
patients metabolic response like weight, medication
and information about the current training state.
The available weather data only had a minor
influence and lowers the precision of the model.
This may reflect the fact that weather has no direct
influence on the patient when he trains in a tempered
environment. However, that does not mean that the
direct environment has no influence at all. We want
to examine this by the measurement of the
conditions inside the training area. Furthermore we
are going to examine if the weather indirectly affects
the Borg value, another very important value to
control the intensity of the rehabilitation training.
The influence of the resting HR at the beginning
of a training in S3 leads to a good precision of the
model during the training itself. This predictor is
probably influenced by many other, hard to measure
variables like medical treatment, stress, dehydration
and coffee consumption, which might have a strong
impact on the metabolic system. This leads to the
unexpected observation that the given blood
pressure values show only a very small effect on the
HR. Blood pressure kinetics are in close relationship
to HR, but not to absolute values, due to
antihypertensive treatment in most patient’s.
The prediction can be used to estimate the
patient’s physical state on the day of testing and
thereby help to define an appropriate training
intensity before the training starts.
The phase-wise prediction in S4 during the
runtime of the training shows a relative error below
5%. This should be precise enough to robustly detect
abnormal HR developments and calculate the
optimal load for the next phase. In future we will
focus on the analysis of other time dynamic
predictors that might increase the model accuracy
and also facilitate high refresh rates without the
abstract distinction between training phases.
5 CONCLUSIONS
We created a statistical model to predict HR as an
important vital parameter for the rehabilitation
training of cardiopulmonary patients. We considered
demographic data, training plan information, other
vital parameters and weather information as
potential predictors and classified them into five
aim-specific scenarios. The validation of the model
revealed that weather and the measured blood
pressure have nearly no direct influence on HR. Age
and previously measured HR based variables like the
resting HR strongly influence the responding HR.
The model exhibits an overall low error of ≈11
bpm in median, when used for the creation of a
training schedule (scenario 1). The error is reduced
by about 50%, when the model is used for prediction
at the beginning of a training session. The error
decreases below a significance level when the model
is used during a training to predict HR at the
beginning of each of the four training phases. This
makes it potentially suitable to detect critical
situations before they appear.
The precision of the prediction might be
improved by additionally including expert
knowledge and further statistical methods, but it
already serves as a good basis for the integration of
HR predictive mechanisms into training related
systems and might potentially increase the safety
and efficiency during the rehabilitation training of
cardiopulmonary patients.
ACKNOWLEDGEMENTS
This work was funded in part by the Ministry for
Science and Culture of Lower Saxony within the
Research Network “Design of Environments for
Ageing” (grant VWZN 2420/2524).
REFERENCES
Achten, J., Jeukendrup, A. E., 2003. Heart rate
monitoring: applications and limitations. Sports Med,
33(7):517–538.
Borg, G., 1970. Perceived exertion as an indicator of
somatic stress. Scandinavian journal of Rehabilitation
Medicine, 2(2):92–98.
Cheng, T. M., Savkin, A. V., Celler, B. G., Su, S. W.,
Wang, L., 2008. Nonlinear modeling and control of
human heart rate response during exercise with
various work load intensities. IEEE J BME,
55(11):2499–2508.
Hair, J. F., Black, W. C., Babin, B. J., Anderson, R. E.,
Tatham, R. L., 2006. Multivariate data analysis.
Pearson/Prentice Hall, Upper Saddle River, NJ, 6. ed.
edition. ISBN 0-13-032929-0.
Helmer, A., Song, B., Ludwig, W., Schulze, M.,
Eichelberg, M., Hein, A., Tegtbur, U., Kayser, R.,
Haux, R., Marschollek, M., 2010. A sensor-enhanced
health information system to support automatically
controlled exercise training of COPD patients. In
Pervasive Computing Technologies for Healthcare
(Pervasive Health), 2010 4th International Conference
on, pages 1 – 6.
Lipprandt, M., Eichelberg, M., Thronicke, W., Kruger, J.,
Druke, I., Willemsen, D., Busch, C., Fiehe, C., Zeeb,
E., Hein, A., 2009. OSAMI-D: An open service
A HEART RATE PREDICTION MODEL FOR THE TELEREHABILITATION TRAINING OF CARDIOPULMONARY
PATIENTS
35