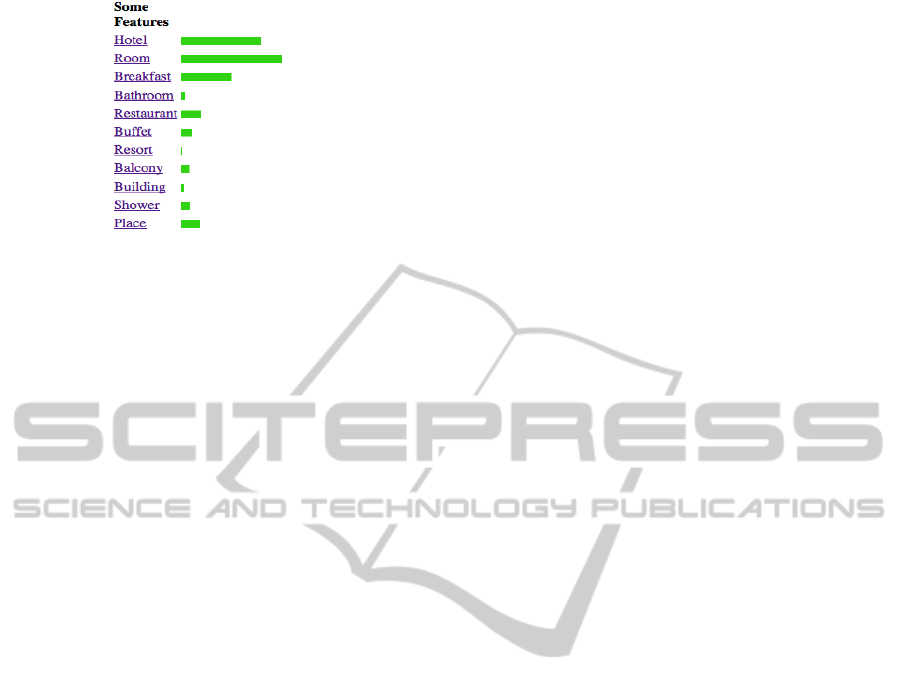
Figure 2: A sample of opinion summarization.
sample of result of the opinion summarization task.
The figure illustrates a simple polarity evaluation
comparing the preferences about the represented
features. In the phase of data aggregation, it is
important to notice relations between features, e.g.
breakfast and buffet, as showed in the first picture in
order to give a correct interpretation of data. The
system currently does not analyse this kind of
relations between data, but is able to deduce only
that users like the breakfast of the hotel but less the
buffet organization.
5 FUTURE WORKS
Several Opinion Mining methods and techniques
have been developed in order to analyse contents
and reviews. In this paper an automatic approach to
the extraction of feature terms has been proposed.
With the introduction of the synsets and the
semantic categorization, we aim to define a method
of extraction of more accurate meanings and features
from textual resources. We propose the
identification of the context of features by means of
the semantic net of WordNet in order to reach a
more complete list of features and attributes related
to an object. Future works will provide the
development of the opinion summarization task, the
definition of a tool for the navigation of features and
related opinions and the generalization of the
approach in order to apply it to general contexts.
The tool will perform opinion monitoring activities,
an essential task in listening to and taking advantage
from consumer preferences and opinions. A
validation to support the value of the expressed ideas
will be one of the goals of the above mentioned
approach and experimental results will be product.
REFERENCES
Akkaya, C., Wiebe, J., Mihalcea, R., 2009. Subjectivity
word sense disambiguation. In Conference on Empirical
Methods in Natural Language Processing, Singapore,
The Association for Computational Linguistics.
Benamara, F., Cesarano, C., Picariello, A., Reforgiato, D.,
Subrahmanian, V. S., 2007. Sentiment Analysis:
Adjectives and Adverbs are better than Adjectives
Alone. In Proceedings of ICWSM 07 International
Conference on Weblogs and Social Media.
Crimson Hexagon, 2009. Listen, Understand, Act. How a
listening platform provides actionable insight. http://
www.crimsonhexagon.com/PDFs/Crimson_Hexagon_
Listen_Understand_Feb_2009.pdf
Ding, X., Liu, B., Yu, P. S., 2008. A Holistic Lexicon-
Based Approach to Opinion Mining. WSDM '08 Pro-
ceedings of the international conference on Web
search and web data mining, ACM, New York, USA.
Esuli, A. Sebastiani, F., 2007. PageRanking WordNet
synsets: An application to Opinion Mining.
Proceedings of the 45th Annual Meeting of the
Association of Computational Linguistics Volume: 45,
Issue: June, Publisher: Association for Computational
Linguistics, Pages: 424-431
Gartner, 2011. Gartner's 2011 Hype Cycle Special Report
Evaluates the Maturity of 1,900 Technologies. http://
www.gartner.com/it/page.jsp?id=1763814
Leacock C. and Chodorow M. 1998. Combining local
context and WordNet similarity for word sense
identification. In Fellbaum 1998, pp. 265-283.
Lee, D., Jeong, O. R., Lee, S., 2008. Opinion Mining of
customer feedback data on the web. In ICUIMC '08
Proceedings of the 2nd international conference on
Ubiquitous information management communication
Magnini, B., Strapparava, C., Pezzulo, G., Gliozzo, A.,
2002. The Role of Domain Information in Word Sense
Disambiguation. Natural Language Engineering,
special issue on Word Sense Disambiguation, 8(4), pp.
359-373, Cambridge University Press
Miller, G., 1998. WordNet: An Electronic Lexical
Database, Bradford Books
Popescu, A. M., Etzioni, O, 2005. Extracting Product
Features and Opinions from Reviews. Proceedings of
the 2005 Conference on Empirical Methods in Natural
Language Processing.(EMNLP’05).
Rentoumi, V., Giannakopoulos, G., 2009. Sentiment
analysis of figurative language using a word sense
disambiguation approach. In International Conference
on Recent Advances in Natural Language Processing
(RANLP 2009), Borovets, Bulgaria, The Association
for Computational Linguistics
Yi, J., Nasukawa, T., Bunescu, R., Niblack, W., 2003.
Sentiment analyzer: Extracting sentiments about a
given topic using natural language processing
techniques. In Proceedings of the IEEE International
Conference on Data Mining.
Zhai, Z., Liu, B., Xu, H., Jia, P., 2010. Grouping Product
Features Using Semi-Supervised Learning with Soft-
Constraints. In Proceedings of the 23rd International
Conference on Computational Linguistics (COLING-
2010), Beijing, China.
ICAART 2012 - International Conference on Agents and Artificial Intelligence
476