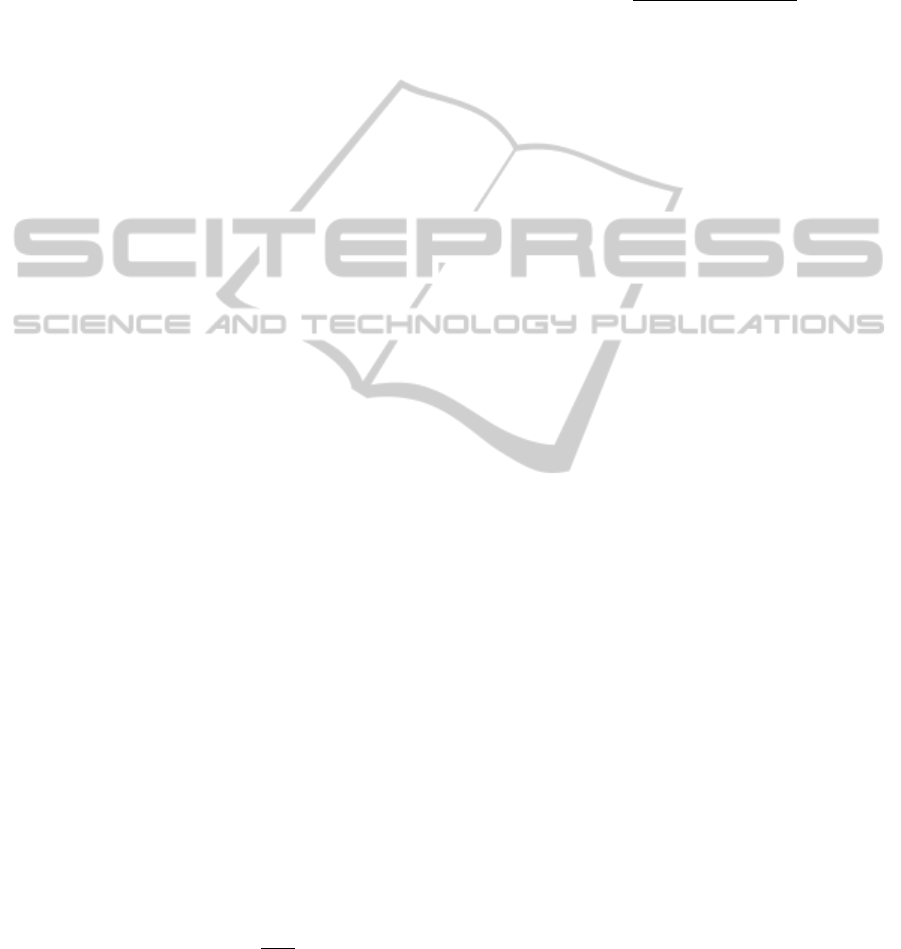
t ∈ T = (0, 1, 2,.. .). The winning decision of MG
(resp. MJ) is determined by the minority (resp. ma-
jority) group of −1 or 1. Each strategy R
i,a
(µ) ∈ R
i,a
is given a score U
i,a
(t) so that the best strategy can
make a winning decision. For the last m winning de-
cisions, denoted by µ = h
m
(t − 1) ⊆ H, agent i’s strat-
egy R
i,a
(µ) ∈ R
i,a
determines −1 or 1 by (1). Among
them, each agent i selects his highest scored strategy
R
∗
i
(µ) ∈ R
i,a
and makes a decision a
i
(t) = R
∗
i
(µ) at
time t ∈ T. The highest scored strategy is represented
by
R
∗
i
(µ) = arg max
a∈{1,...,s}
U
i,a
(t), (2)
which is randomly selected if there are many ones. An
aggregate value A(t) =
∑
N
i=1
a
i
(t) is called an excess
demand. If A(t) > 0, agents with a
i
(t) = −1 win, and
otherwise, agents with a
i
(t) = 1 win in MG, and vice
versa in MJ. Hence the payoffs g
MG
i
and g
MJ
i
of agent
i are represented by
g
MG
i
(t + 1) = −a
i
(t)A(t) and (3)
g
MJ
i
(t + 1) = a
i
(t)A(t), respectively. (4)
The winning decision h(t) = −1 or 1 is added to the
end of the history H, i.e., h
m+1
(t) = [h
m
(t − 1),h(t)],
and then it will be reflected in the next step. After the
winning decision has been turned out, every score is
updated by
U
i,a
(t + 1) = U
i,a
(t) ⊕ R
i,a
(µ) · sgn(A(t)), (5)
where ⊕ means subtraction for MG (addition for MJ)
and sgn(x) = 1 (x ≥ 0), = −1 (x < 0). In other
words, the scores of winning strategies are increased
by 1, while those of losing strategies are decreased
by 1. We simply say that an agent increases selling
(resp. buying) strategies if the scores of selling (resp.
buying) strategies are increased by 1. Likewise the
decrement of scores. Notice that the score is an ac-
cumulated value from an initial state in the original
MG. In contrast, we define it as a value from the last
H
p
steps according to (Liu et al., 2004). That is, we
use
U
i,a
(t+1) = U
i,a
(t)⊕R
i,a
(µ)·sgn(A(t))−U
i,a
(t−H
p
).
(6)
The constant H
p
is not relevant to m, but is only used
for selecting the highest score. Analogous to a fi-
nancial market, the decision a
i
(t) = 1 (respectively,
−1) represents buying (respectively, selling) an asset.
Usually, the price of an asset is defined as
p(t + 1) = p(t) · exp
A(t)
N
. (7)
2.2 Asset Value Game
The difference between MG and our asset value game
is the payoff function. Let v
i
(t) be agent i’s mean
asset value at time t, and u
i
(t) the number of units of
his asset. The payoff function in AG is defined as
g
AG
i
(t + 1) = −a
i
(t)F
i
(t), (8)
where F
i
(t) = p(t) − v
i
(t). The mean asset value v
i
(t)
and the number of asset units u
i
(t) are updated by
v
i
(t + 1) =
v
i
(t)u
i
(t) + p(t)a
i
(t)
u
i
(t) + a
i
(t)
(9)
and
u
i
(t + 1) = u
i
(t) + a
i
(t), (10)
respectively. That is, the payoff function (3) in MG is
replaced by (8) in AG. Without loss of generality, we
assume that v
i
(t), u
i
(t) > 0 for any t ∈ T.
The basic idea behind the payoff function is that
each agent wants to decrease his acquisition cost in
order to make his appraisal gain. Figure 2(a) shows
the relationship between the price and the mean asset
values of N = 3 agents, where the price is represented
by the solid, heavy line. Notice that if the population
size N is small, the price change becomes drastic.
The most important feature of the AG is to ap-
preciate the past gains and losses. Even though an
agent has bought a high-priced asset during the asset-
inflated term (see Figure 1), the mean asset value of
the agent reflects the fact and an appropriate action
compared with the current price is recommended.
2.3 Extended Asset Value Game
Here we consider the drawbacks of AG, and present
an extended AG, denoted by ExAG, to improve them.
Though the AG captures a good feature of an agent’s
behavior, the payoff function indirectly appreciates
desirable strategies. If the adopted strategy is not de-
sirable, the agent has to wait until the desirable one
gains the highest score. So, there is a time lag be-
tween the rapid change of a price and the adjustment
of an agent’s behavior.
More precisely, the movement of price is followed
by the asset values (see arrows in Figure 2(a)). This
behavior can be explained by the following reasons. If
the price rapidly rises, it exceeds almost all the mean
asset values. Then, F
i
(t) = p(t) − v
i
(t) becomes plus
and the a
i
(t) = −1 (i.e., sell) action is recommended.
So, some agents change from trend-followers to con-
trarians in a few steps. During the steps, such agents
remain trend-followers, that is, buy assets at the high
price. Thus, their mean asset values follow the move-
ment of price.
Our solution is to provide another option of the
agent. That is, the agent who has much higher/lower
asset value than the current price can directly act as
the payoff function, called a direct action. However,
A NEW VARIANT OF THE MINORITY GAME - Asset Value Game and Its Extension
17