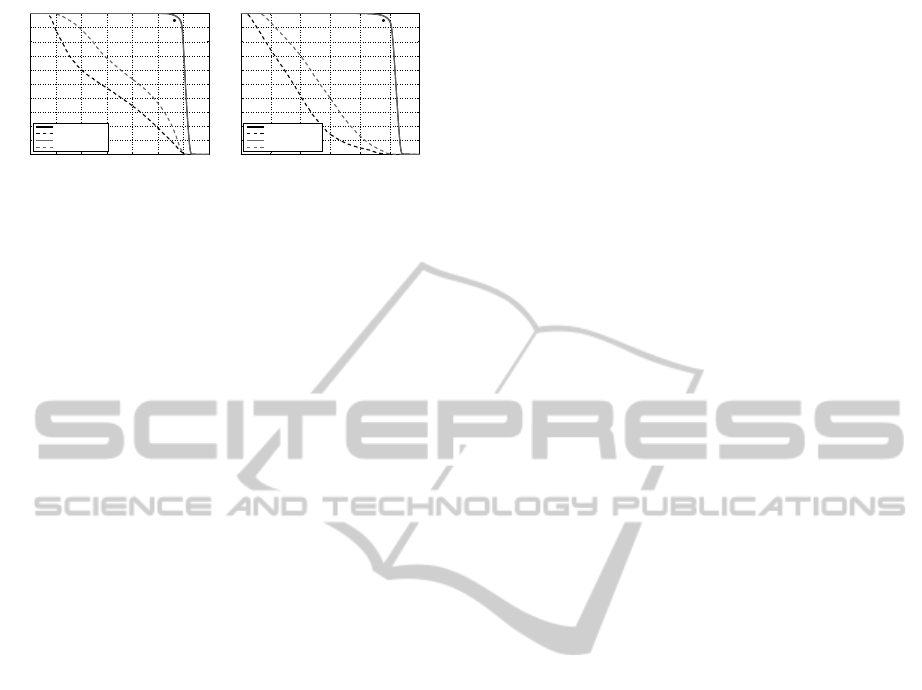
0 10 20 30 40 50 60 70
0
10
20
30
40
50
60
70
80
90
100
Dose (Gy)
Percent Volume (%)
PTV left − 5 PSM
Left parotid − 5 PSM
PTV left − 5 equi
Left parotid − 5 equi
0 10 20 30 40 50 60
0
10
20
30
40
50
60
70
80
90
100
Dose (Gy)
Percent Volume (%)
PTV right − 5 PSM
Right parotid − 5 PSM
PTV right − 5 equi
Right parotid − 5 equi
Figure 2: Cumulative dose volume histogram comparing
the treatment plans 5 PSM and 5 equi.
prescribed dose. Typically, 95% of the PTV volume
is required. DVH results are displayed in Figure 2.
Since parotids are the most difficult organs to spare,
for clarity, the DVH only includes the targets and the
parotids. The asterisk indicates 95% of PTV volume
versus 95% of the prescribed dose. By observing Fig-
ure 2 we confirm that both treatment plans fulfill the
goal of having 95% of the prescribed dose for 95%
of the volume for both PTV right and PTV left. Fo-
cusing in parotid sparing we can observe that a better
parotid sparing can be obtained using the beam angle
solution obtained by SID-PSM.
5 CONCLUSIONS
The BAO problem is a continuous global highly non-
convex optimization problem known to be extremely
challengingand yet to be solved satisfactorily. Pattern
search methods framework is a suitable approach for
the resolution of the non-convex BAO problem due
to their structure, organized around two phases at ev-
ery iteration. The poll step, where convergence to a
local minima is assured, and the search step, where
flexibility is conferred to the method since any strat-
egy can be applied. We have shown that a beam angle
set can be locally improved in a continuous manner
using pattern search methods. The initial mesh-size
parameter importance and other strategies for a better
coverage and exploration of the BAO problem search
space were tested and debated. In future work, pat-
tern search methods improvement will be tested with
the incorporation of additional global strategies in the
search step such as response surface approaches or ra-
dial basis functions interpolation. We have to high-
light the low number of function evaluations required
by SID-PSM to obtain locally optimal solutions. The
efficiency on the number of function value computa-
tion is ofthe utmost importance, particularlywhen the
BAO problem is modeled using the optimal values of
the FMO problem. Thus, the global strategies to be
incorporated must comply with this requirement.
ACKNOWLEDGEMENTS
This work has been partially supported by FCT under
project grant PEst-C/EEI/UI0308/2011. The work of
H. Rocha was supported by the European social fund
and Portuguese funds from MCTES.
REFERENCES
Alberto, P., Nogueira, F., Rocha, H. and Vicente, L.
N. (2004). Pattern search methods for user-provided
points: Application to molecular geometry problems.
SIAM J. Optim., 14, 1216–1236.
Aleman, D. M., Kumar, A., Ahuja, R. K., Romeijn, H. E.
and Dempsey, J. F. (2008). Neighborhood search ap-
proaches to beam orientation optimization in inten-
sity modulated radiation therapy treatment planning.
J. Global Optim., 42, 587–607.
Craft, D., Halabi, T., Shih, H. and Bortfeld, T. (2006). Ap-
proximating convex Pareto surfaces in multiobjective
radiotherapy planning. Med. Phys., 33, 3399–3407.
Craft, D. (2007). Local beam angle optimization with linear
programming and gradient search. Phys. Med. Biol.,
52, 127–135.
Cust´odio, A. L. and Vicente, L. N. (2007). Using sam-
pling and simplex derivatives in pattern search meth-
ods. SIAM J. Optim., 18, 537–555.
Cust´odio, A. L., Rocha, H. and Vicente, L. N. (2010). Incor-
porating minimum Frobenius norm models in direct
search. Comput. Optim. Appl., 46, 265–278.
Das, S. K. and Marks, L. B. (1997). Selection of coplanar
or non coplanar beams using three-dimensional op-
timization based on maximum beam separation and
minimized nontarget irradiation. Int. J. Radiat. Oncol.
Biol. Phys., 38, 643–655.
Davis, C. (1954). Theory of positive linear dependence. Am.
J. Math., 76, 733–746.
Deasy, J. O., Blanco, A. I. and Clark, V. H. (2003). CERR:
A Computational Environment for Radiotherapy Re-
search. Med. Phys., 30, 979–985.
Lee, E. K., Fox, T. and Crocker, I. (2006). Simultane-
ous beam geometry and intensity map optimization in
intensity-modulated radiation therapy. Int. J. Radiat.
Oncol. Biol. Phys., 64, 301–320.
Mor´e, J. and Wild, S. (2009). Benchmarking Derivative-
Free Optimization Algorithms, SIAM J. Optim., 20,
172–191.
Romeijn, H. E., Ahuja, R. K., Dempsey, J. F., Kumar, A.
and Li, J. (2003). A novel linear programming ap-
proach to fluence map optimization for intensity mod-
ulated radiation therapy treatment planing. Phys. Med.
Biol., 48, 3521–3542.
Schreibmann, E., Lahanas, M., Xing, L. and Baltas, D.
(2004). Multiobjective evolutionary optimization of
the number of beams, their orientations and weights
for intensity-modulated radiation therapy. Phys. Med.
Biol., 49, 747–770.
ICORES 2012 - 1st International Conference on Operations Research and Enterprise Systems
360