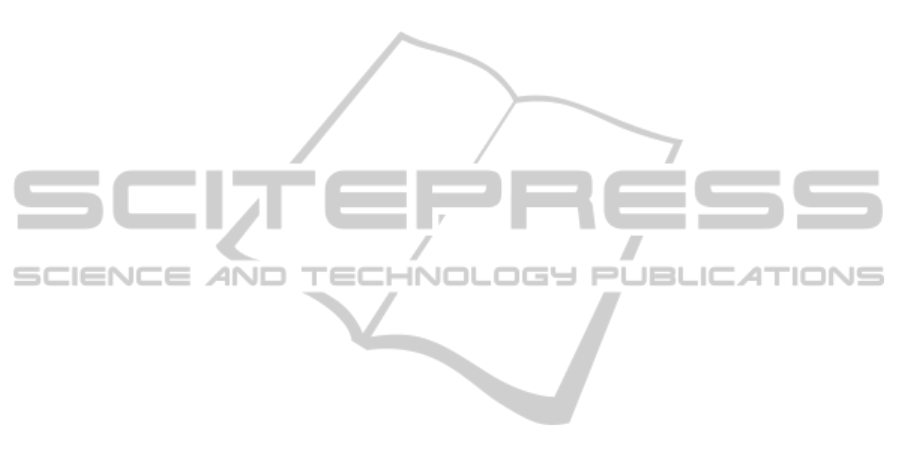
period in modern history, using costs of an Amazon
Web Service cloud instance, and where users submit
probabilities based on previous demand. It was
found that the coordinator profits in such a situation
in a number of market segments, thereby
demonstrating that a stable commercial
implementation is feasible.
It was also found that the Coordinator is better
off considering past performance when decided to
invest in another reserved instance, and this can
increase profits by up to 28%.
Wu et al.’s model provides a suitable theoretical
model for an options-market in computing resource.
However, the service provider would have to
provide specific pricing to support the Coordinator,
and this might not always be profitable for the
service provider. Our extension to this model does
not require new pricing to be agreed, but contract
restrictions on reselling may be a barrier to
commercial implementation.
Our work shows that a probability-based options
market in computing capability is a viable
commercial proposition, and that all parties can
potentially benefit as a result of such a system. The
advantage of this approach is that a forecast of future
usage requirements is obtained, which can
subsequently used to plan future capacity
requirements and so that targets on performance as
detailed in a Service Level Agreement can be met.
These are currently issues for widespread federated
cloud adoption.
The simulation has shown that the reservation
model may be suitable for real-world application.
The model provides a platform for further risk
assessment work to be undertaken and, as discussed,
the simulator can be further extended to simulate a
variety of market conditions, or specific user
demands.
The optimum threshold is the value at which
market demand is fully anticipated by the
Coordinator, and which is fully provisioned through
reserved instances. Determining this threshold
mathematically is likely to be challenging due to
difficultly in determining market dynamics over a
very long period. However, an empirical simulation
using actual market data could produce such a
threshold for commercial implementation.
By taking the results from this paper and
extending them with future research into the
performance of the model under different conditions
and inherent honesties, in different segments, a
commercial offering that is profitable to the
coordinator, beneficial to the user, and with a
calculated level of risk looks likely to be achievable.
ACKNOWLEDGEMENTS
We thank the UK EPSRC for funding the Large-
Scale Complex IT Systems Initiative
(www.lscits.org) as well as HP Labs Adaptive
Infrastructure Lab for providing additional financial
support.
REFERENCES
Buyya, R., Ranjan, R., & Calheiros, R. N. (2010).
InterCloud : Utility-Oriented Federation of Cloud
Computing Environments for Scaling of. Network,
13-31.
Buyya, R., Yeo, C. S., Venugopal, S., Broberg, J., &
Brandic, I. (2009). Cloud computing and emerging IT
platforms: Vision, hype, and reality for delivering
computing as the 5th utility. Future Generation
Computer Systems, 25(6)
Clearwater, S. H., & Huberman, Bernando. (2005). Swing
Options : A Mechanism for Pricing IT Peak Demand.
Proceedings of 11th International Conference on
Computing in Economics.
Hilley, D. (2009). Cloud Computing : A Taxonomy of
Platform and Infrastructure-level Offerings Cloud
Computing : A Taxonomy of Platform and
Infrastructure-level Offerings. Technology, (April).
Hull, J. C. (2005). Fundamentals of Futures and Options
Markets.
Khajeh-Hosseini, A., Sommerville, I., & Sriram, I. (2010).
Research Challenges for Enterprise Cloud Computing.
Arxiv preprint
Rogers, O. and Cliff, D. (2010a), The Effects of
Truthfulness on a Computing Resource Options
Market, Proceedings of the Conference on Advances
in Distributed and Parallel Computing
Rogers, O. and Cliff, D. (2010b), The Effects of Market
Demand on Truthfulness in a Computing Resource
Options Market, Proceedings of the 3rd International
Conference on Agents and Artificial Intelligence.
Sandholm, T., & Lai, K (2007), A statistical approach to
risk mitigation in computational markets. Proceedings
of Conference on High Performance Parallel and
Distributed Computing
Stage, A., & Setzer, T. (2009). Network-aware migration
control and scheduling of differentiated virtual
machine workloads. 2009 ICSE Workshop on
Software Engineering Challenges of Cloud
Computing, 9-14.
Wu, F., Zhang, L., & Huberman, Ba. (2008). Truth-telling
reservations. Proceedings of 11th International
Conference on Computing in Economics.
Yeo, C. S., & Buyya, R. (2006). A taxonomy of market-
based resource management systems for utility-driven
cluster. Cluster Computing, (June), 1381-1419. doi:
10.1002
FORECASTING DEMAND FOR CLOUD COMPUTING RESOURCES - An Agent-based Simulation of a Two Tiered
Approach
111