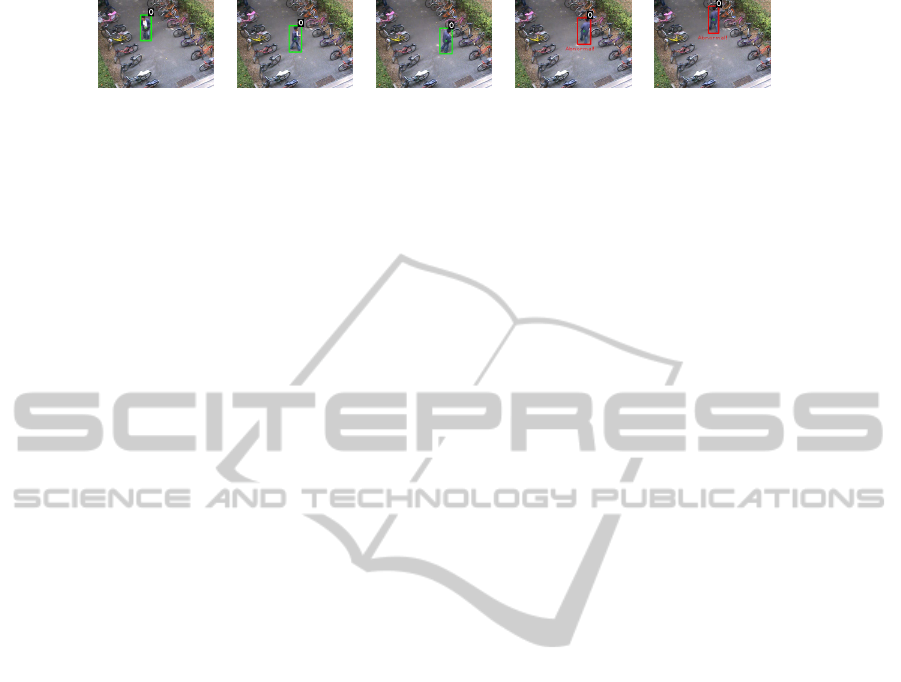
Frame 187 Frame 208 Frame 225 Frame 249 Frame 267
Figure 4: Example anomaly detected by the proposed method in Experiment I. The sequence contains a person walking
around looking for an unlocked bicycle.
distance to the separating hyperplane used for clas-
sification as normal or abnormal. Table 1 shows
the detailed anomaly detection results for SVMs at a
100% hit rate. Although the results are clearly better
than those obtained from k-NN or PCA, they are also
clearly inferior to those obtained in Experiment I.
5 DISCUSSION AND
CONCLUSIONS
We have proposed and evaluated a new method for
bootstrapping scene-specific anomalous human be-
havior detection systems. It requires minimal involve-
ment of a human operator; the only required action is
to label the patterns in a small bootstrap set as nor-
mal or anomalous. With a bootstrap set of 150 se-
quences, the method achieves a false positive rate of
merely 7.4% at a hit rate of 100%. The experiments
demonstrate that with a collection of simple HMMs,
it is possible to learn a complex set of varied behav-
iors occurring in a specific scene. Deploying our sys-
tem on a large video sensor network would potentially
lead to substantial increases in the productivity of hu-
man monitors.
The main limitation of our current method is that
the blob tracking process is not robust for complex
events involving multiple people. The method also
does not allow evolution of the learned bootstrap
model over time. In future work, we plan to address
these limitations.
ACKNOWLEDGEMENTS
This work was partly supported by a grant from the
Royal Thai Government to MND and by graduate fel-
lowships from the Royal Thai Government to KO. We
thank the AIT Computer Vision Group for valuable
discussions and comments on this work.
REFERENCES
Chang, C.-C. and Lin, C.-J. (2001). LIBSVM: a library for
support vector machines. http://www.csie.ntu.edu.tw/
∼cjlin/libsvm.
Gao, J., Hauptmann, A. G., Bharucha, A., and Wactlar,
H. D. (2004). Dining activity analysis using a hid-
den Markov model. In International Conference on
Pattern Recognition (ICPR), pages 915–918.
Li, H., Hu, Z., Wu, Y., and Wu, F. (2006). Behavior mod-
eling and recognition based on space-time image fea-
tures. In International Conference on Pattern Recog-
nition (ICPR), pages 243–246.
Nair, V. and Clark, J. (2002). Automated visual surveillance
using hidden Markov models. In Vision Interface Con-
ference, pages 88–92.
Oates, T., Firoiu, L., and Cohen, P. R. (2001). Using dy-
namic time warping to bootstrap HMM-based cluster-
ing of time series. In Sequence Learning: Paradigms,
Algorithms, and Applications, pages 35–52.
Ouivirach, K. and Dailey, M. N. (2010). Clustering hu-
man behaviors with dynamic time warping and hid-
den Markov models for a video surveillance system.
In ECTI-CON, pages 884–888.
Pass, G., Zabih, R., and Miller, J. (1996). Comparing im-
ages using color coherence vectors. In International
Conference on Multimedia, pages 65–73.
Poppe, C., Martens, G., Lambert, P., and de Walle, R. V.
(2007). Improved background mixture models for
video surveillance applications. In Asian Conference
on Computer Vision (ACCV), pages 251–260.
Sminchisescu, C., Kanaujia, A., Li, Z., and Metaxas, D.
(2005). Conditional random fields for contextual hu-
man motion recognition. In International Conference
on Computer Vision (ICCV), pages 1808–1815.
Swears, E., Hoogs, A., and Perera, A. (2008). Learning mo-
tion patterns in surveillance video using HMM clus-
tering. In IEEE Workshop on Motion and video Com-
puting (WMVC), pages 1–8.
Wu, X., Ou, Y., Qian, H., and Xu, Y. (2005). A detection
system for human abnormal behavior. In International
Conference on Intelligent Robots and Systems (IROS),
pages 1204–1208.
Xiang, T. and Gong, S. (2005). Video behaviour pro-
filing and abnormality detection without manual la-
belling. In International Conference on Computer Vi-
sion (ICCV), pages 1238–1245.
VISAPP 2012 - International Conference on Computer Vision Theory and Applications
658