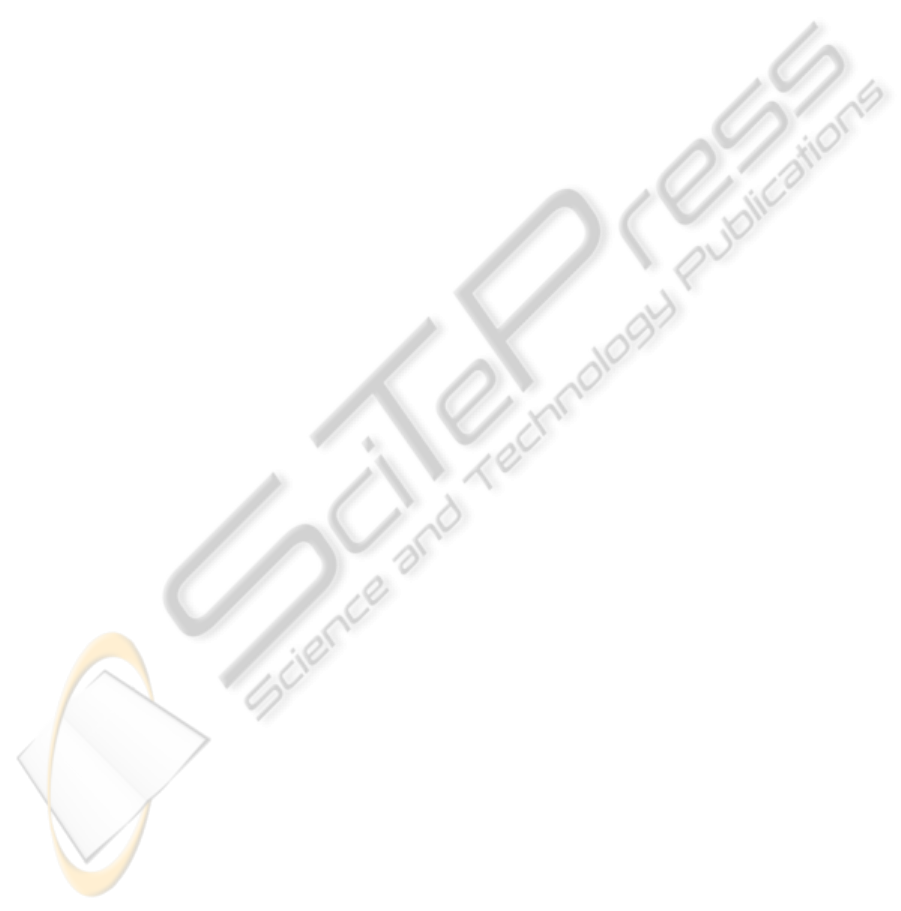
The intrinsic limitation presented in this paper
changes the way we should interpret some of the pre-
vious reported results, and has to be taken into ac-
count for designing new methods. It has an impact at
many levels: on the choice of the sensors best suited
for the pose recovery, on the choice of the approach
to follow (model-based, example-based, or learning-
based), as well as on the choice of the attributes to use
for learning-based methods.
REFERENCES
Agarwal, A. and Triggs, B. (2005). Monocular human mo-
tion capture with a mixture of regressors. In IEEE
Computer Society Conference on Computer Vision
and Pattern Recognition Workshops, volume 3, San
Diego, USA.
Agarwal, A. and Triggs, B. (2006). Recovering 3D human
pose from monocular images. IEEE Transactions on
Pattern Analysis and Machine Intelligence, 28(1):44–
58.
Barnich, O. and Van Droogenbroeck, M. (2011). ViBe: A
universal background subtraction algorithm for video
sequences. IEEE Transactions on Image Processing,
20(6):1709–1724.
Elgammal, A. and Lee, C. (2009). Tracking people on a
torus. IEEE Transactions on Pattern Analysis and Ma-
chine Intelligence, 31(3):520–538.
Geurts, P., Ernst, D., and Wehenkel, L. (2006). Extremely
randomized trees. Machine Learning, 63(1):3–42.
Girshick, R., Shotton, J., Kohli, P., Criminisi, A., and
Fitzgibbon, A. (2011). Efficient regression of general-
activity human poses from depth images. In In-
ternational Conference on Computer Vision (ICCV),
Barcelona, Spain.
Gond, L., Sayd, P., Chateau, T., and Dhome, M. (2008).
A 3D shape descriptor for human pose recovery. In
Perales, F. and Fisher, R., editors, Articulated Mo-
tion and Deformable Objects, volume 5098 of Lecture
Notes in Computer Science, pages 370–379. Springer.
Howe, N. (2004). Silhouette lookup for automatic pose
tracking. In IEEE Computer Society Conference on
Computer Vision and Pattern Recognition Workshops,
volume 1, pages 15–22, Washington, USA.
Moeslund, T., Hilton, A., and Kr
¨
uger, V. (2006). A sur-
vey of advances in vision-based human motion cap-
ture and analysis. Computer Vision and Image Under-
standing, 104:90–126.
Peng, B. and Qian, G. (2008). Binocular dance pose recog-
nition and body orientation estimation via multilinear
analysis. In IEEE Computer Society Conference on
Computer Vision and Pattern Recognition Workshops,
Anchorage, USA.
Pi
´
erard, S., Leroy, D., Hansen, J.-F., and Van Droogen-
broeck, M. (2011). Estimation of human orientation
in images captured with a range camera. In Advances
Concepts for Intelligent Vision Systems (ACIVS), vol-
ume 6915 of Lecture Notes in Computer Science,
pages 519–530. Springer.
Poppe, R. and Poel, M. (2006). Comparison of sil-
houette shape descriptors for example-based human
pose recovery. In International Conference on Auto-
matic Face and Gesture Recognition, pages 541–546,
Southampton, UK.
Rosales, R. and Sclaroff, S. (2001). Learning body pose via
specialized maps. In Proceedings of Neural Informa-
tion Processing Systems, pages 1263–1270, Vancou-
ver, Canada.
Shakhnarovich, G., Viola, P., and Darrell, T. (2003). Fast
pose estimation with parameter-sensitive hashing. In
International Conference on Computer Vision (ICCV),
volume 2, pages 750–757, Nice, France.
Shotton, J., Fitzgibbon, A., Cook, M., Sharp, T., Finocchio,
M., Moore, R., Kipman, A., and Blake, A. (2011).
Real-time human pose recognition in parts from sin-
gle depth images. In IEEE International Conference
on Computer Vision and Pattern Recognition (CVPR),
Colorado Springs.
Sminchisescu, C., Kanaujia, A., Li, Z., and Metaxas, D.
(2005). Discriminative density propagation for 3d hu-
man motion estimation. In IEEE International Con-
ference on Computer Vision and Pattern Recognition
(CVPR), volume 1, pages 390–397, San Diego, USA.
Sminchisescu, C. and Telea, A. (2002). Human pose es-
timation from silhouettes - a consistent approach us-
ing distance level sets. In International Conference
for Computer Graphics, Visualization and Computer
Vision, volume 10, pages 413–420, Plze
˘
n, Czech Re-
public.
Taylor, C. (2000). Reconstruction of articulated objects
from point correspondences in a single uncalibrated
image. Computer Vision and Image Understanding,
80(3):349–363.
ON THE HUMAN POSE RECOVERY BASED ON A SINGLE VIEW
315