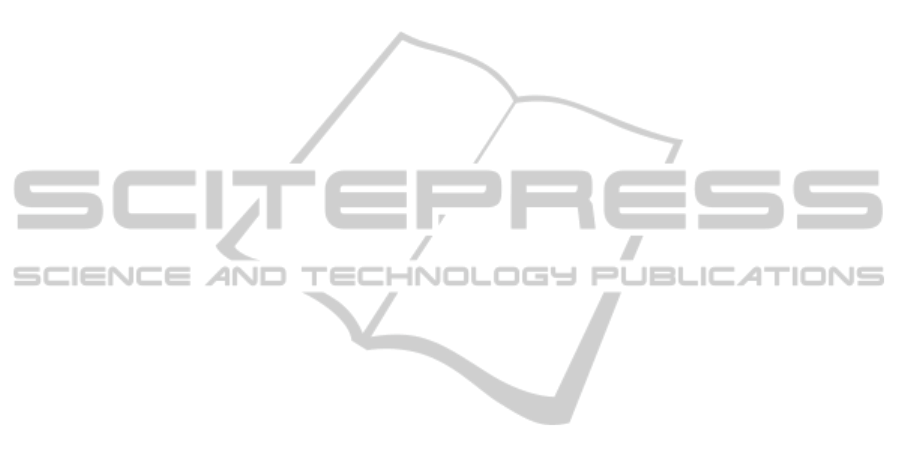
• EEG for stress and depression
• Activity Sensor for ambulatory activity
• ECG for Heart Rate and HR Variability
• Sub Dermal Cortisol Level Sampler
• Speech Analysis for depression score
The ECG and Activity sensors are worn 24/7
while the EEG sensor is to be worn once a day in the
evening for a few minutes in order to perform an
EEG recording and then removed. Data from all the
sensors is received wirelessly from each sensor by a
netbook computer called the HomePC. The HomePC
encrypts the data and transmits it to a central server
which processes the results.
Trials are to be conducted in Switzerland, Spain
and China over 4 weeks in order to collect
physiological data, volunteers’ self-reported data
and therapist interview data. By analysing the data
the plan is to derive heuristic rules linking
physiology to mental health prediction. When the
clinicians are satisfied with the rules the sensors will
be used as part of a Cognitive Behavioural Therapy
based on-line system. This system will be tested as
part of resilience treatment trials to be conducted in
the UK and Spain using the same sensors.
2.1 The Role of the EEG Sensor
The EEG is a record of the electrical potential
gradient oscillating around the brain and recorded
from electrodes on the human scalp and is often
labeled according to apparent frequency ranges
detected in the EEG signal power spectrum: delta (1-
4Hz), theta (4-8Hz), alpha (8-13Hz), beta (13-
20Hz), and gamma (roughly >20Hz). The scalp
electric potential amplitude is typically 20 to 100 μV
with a specific shape depending on the subject’s
state of relaxation (Srinivasan, 2006). Recent studies
have suggested that alpha rhythm is an oscillatory
component of the human EEG and applied widely in
many application fields, such as mental illness,
biometric identification, E-learning, etc. Both wake
and sleep EEG can provide biomarkers of depression
and anti-depressive therapy, respectively (Tang,
2009). Within OPTIMI the resting EEG is recorded
at three points on the forehead (FP1, FP2 and FPZ)
referenced to the two ear lobes (A1 and A2).
The majority of studies targeting depression
report characteristic differences in EEG asymmetry,
especially in the alpha band, recorded from
electrodes placed on frontal locations. In particular
the study performed by Miguel A. Diego supports
previous findings that indicate that greater relative
left frontal EEG alpha activity is evident in people
with depressive symptomology (Diego et al., 2002).
In addition the findings of Vuga et al support the
view that resting frontal EEG asymmetry reflects a
moderately stable individual difference in adults,
irrespective of sex and history of depression. [4]
Another good candidate for predicting
depression is the measurement of EEG signal
complexity. J L Nandrino et al. found that the EEG
dynamics of major depressive subjects is more
predictable, that is less complex, than that of control
subjects(Nandrino et al., 1994). Tang et al. showed
that alpha rhythm entropy in depressives is increased
during resting compared to when perfroming mental
arithmetic and when compared to healthy control
group (Tang, 2009).
Within OPTIMI the two above measures, Alpha
asymmetry and C0 Complexity, will be of particular
interest as well as Beta/Alpha and Alpha/Theta power
band ratios which can reflect a person’s mood.
The EEG sensor must reliably record the user’s
EEG signals over periods of approximately 90
seconds in order to obtain sufficient data for the
above algorithms. To achieve this the sensor must
meet certain electrical specifications which will be
described later. Additionally the sensor should
provide a means to grade the quality of contact
between the electrodes and the skin on the forehead
since this contact quality must be sufficient if the
raw data is to be useful.
Artefacts due to eye movements and forehead
muscle movements are often detected in EEG raw
data. Both 50Hz noise from electrical power lines
and artefacts must be removed by a denoising
algorithm to provide pure data before the algorithms
are applied.
At first the algorithms and de-noising will be
computed on the HomePC with the sensor purely
acting as a wireless EEG recorder controlled by the
HomePC. However once the results of the
calibration trials are fully interpreted the aim is to
move many of these data processing tasks to the
sensor, including a neuro feedback mode, making it
less platform dependent and highly portable.
3 SENSOR HARDWARE
The sensor electronics is depicted in Figure 1. The 5
electrodes on the left are connected first to a signal
conditioning circuit to clamp any voltage spikes that
may arise due to electrostatic discharge. In addition
passive filtering is performed to remove 100Hz or
higher input noise.
The electrodes connect to A1, the left ear lobe,
FP1, the left hand side of the mid forehead, FP Zero,
A LOW COST ERGONOMIC EEG SENSOR FOR PREDICTING MENTAL ILLNESS
165