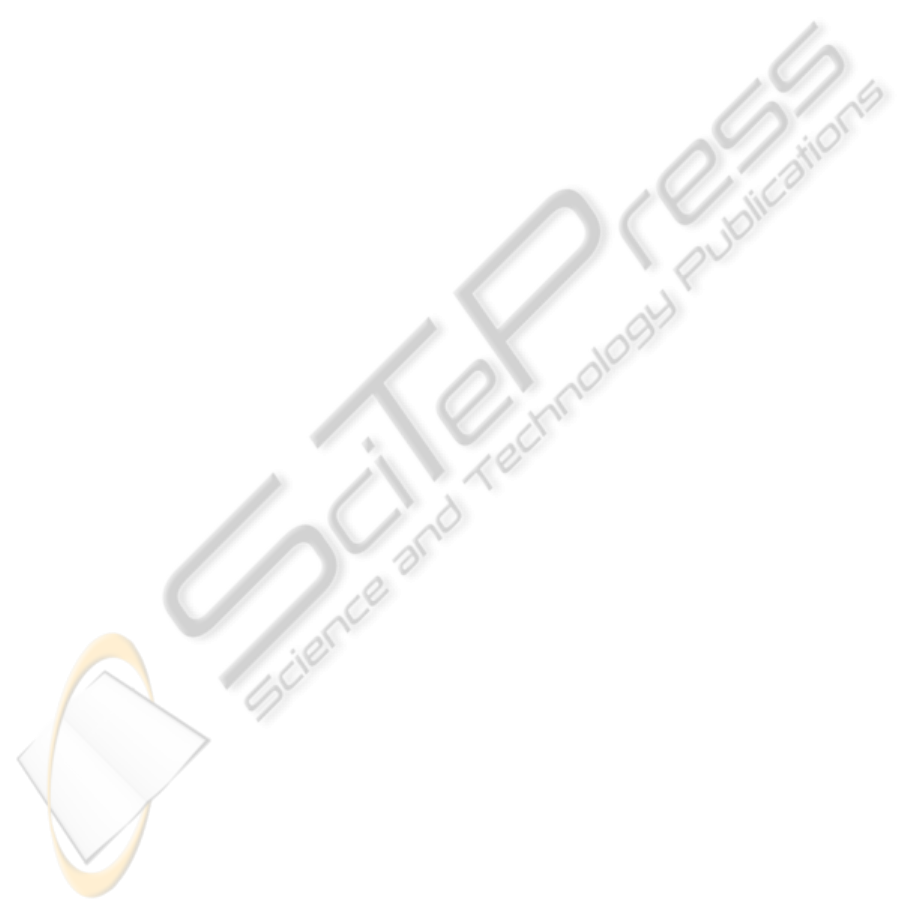
7 CONCLUSIONS
In this work we have presented a super-voxel based
segmentation and classification method for 3D urban
scenes. For segmentation a link-chain method is pro-
posed, which is followed by a classification of objects
using local descriptors and geometrical models. In
order to evaluate our work we have introduced a new
evaluation metric which incorporates both segmenta-
tion and classification results. The results show an
overall segmentation accuracy of 87% and a classifi-
cation accuracy of about 90%.
Our study shows that the classification accuracy
improves by reducing voxel size (with an appropriate
value of c
D
) but at the cost of processing time. Thus
a choice of an optimal value, as discussed, is recom-
mended.
The study also demonstrates the importance of us-
ing intensity values along with RGB colors in the seg-
mentation and classification of urban environment as
they are illumination invariant and more consistent.
The proposed method can also be used as an add-
on boost for other classification algorithms.
ACKNOWLEDGEMENTS
This work is supported by the Agence Nationale de
la Recherche (ANR - the French national research
agency) (ANR CONTINT iSpace&Time – ANR-10-
CONT-23) and by “le Conseil G´en´eral de l’Allier”.
The authors would like to thank Pierre Bonnet and all
the other members of Institut Pascal who contributed
to this project.
REFERENCES
Anguelov, D., Taskar, B., Chatalbashev, V., Koller, D.,
Gupta, D., Heitz, G., and Ng, A. (2005). Discrim-
inative Learning of Markov Random Fields for Seg-
mentation of 3D Scan Data. In Computer Vision and
Pattern Recognition, IEEE Computer Society Confer-
ence on, volume 2, pages 169–176, Los Alamitos, CA,
USA. IEEE Computer Society.
Douillard, B., Brooks, A., and Ramos, F. (2009). A 3D
Laser and Vision Based Classifier. In 5th Int. Conf. on
Intelligent Sensors, Sensor Networks and Information
Processing (ISSNIP), page 6, Melbourne, Australia.
Douillard, B., Underwood, J., Kuntz, N., Vlaskine, V.,
Quadros, A., Morton, P., and Frenkel, A. (2011). On
the Segmentation of 3D LIDAR Point Clouds. In
IEEE Int. Conf. on Robotics and Automation (ICRA),
page 8, Shanghai, China.
Felzenszwalb, P. and Huttenlocher, D. (2004). Ef-
ficient Graph-Based Image Segmentation. Inter-
national Journal of Computer Vision, 59:167–181.
10.1023/B:VISI.0000022288.19776.77.
Golovinskiy, A. and Funkhouser, T. (2009). Min-Cut Based
Segmentation of Point Clouds. In IEEE Workshop on
Search in 3D and Video (S3DV) at ICCV, pages 39 –
46.
Halma, A., ter Haar, F., Bovenkamp, E., Eendebak, P.,
and van Eekeren, A. (2010). Single spin image-ICP
matching for efficient 3D object recognition. In Pro-
ceedings of the ACM workshop on 3D object retrieval,
3DOR ’10, pages 21–26, New York, NY, USA.
Johnson, A. (1997). Spin-Images: A Representation for 3-
D Surface Matching. PhD thesis, Robotics Institute,
Carnegie Mellon University, Pittsburgh, PA.
Kazhdan, M., Funkhouser, T., and Rusinkiewicz, S. (2003).
Rotation Invariant Spherical Harmonic Representa-
tion of 3D Shape Descriptors. In Proceedings of the
2003 Eurographics/ACM SIGGRAPH symposium on
Geometry processing, SGP ’03, pages 156–164, Aire-
la-Ville, Switzerland, Switzerland. Eurographics As-
sociation.
Klasing, K., Althoff, D., Wollherr, D., and Buss, M. (2009).
Comparison of Surface Normal Estimation Methods
for Range Sensing Applications. In IEEE Int. Conf. on
Robotics and Automation, pages 3206 – 3211, Kobe,
Japan.
Knopp, J., Prasad, M., and Gool, L. V. (2010). Orien-
tation invariant 3D object classification using hough
transform based methods. In Proceedings of the ACM
workshop on 3D object retrieval, 3DOR ’10, pages
15–20, New York, NY, USA. ACM.
Lam, J., Kusevic, K., Mrstik, P., Harrap, R., and Greenspan,
M. (2010). Urban Scene Extraction from Mobile
Ground Based LiDAR Data. In International Sympo-
sium on 3D Data Processing Visualization and Trans-
mission, page 8, Paris, France.
Lim, E. and Suter, D. (2007). Conditional Random Field
for 3D Point Clouds with Adaptive Data Reduction.
In International Conference on Cyberworlds, pages
404–408, Hannover.
Lim, E. H. and Suter, D. (2008). Multi-scale Condi-
tional Random Fields for Over-Segmented Irregular
3D Point Clouds Classification. In Computer Vision
and Pattern Recognition Workshop, volume 0, pages
1–7, Anchorage, AK, USA. IEEE Computer Society.
Liu, Y., Zha, H., and Qin, H. (2006). Shape Topics-A Com-
pact Representation and New Algorithms for 3D Par-
tial Shape Retrieval. In IEEE Conf. on Computer Vi-
sion and Pattern Recognition, volume 2, pages 2025–
2032, New York, NY, USA. IEEE Computer Society.
Lu, W. L., Okuma, K., and Little, J. J. (2009). A Hy-
brid Conditional Random Field for Estimating the Un-
derlying Ground Surface from Airborne LiDAR Data.
IEEE Transactions on Geoscience and Remote Sens-
ing, 47(8):2913–2922.
Moosmann, F., Pink, O., and Stiller, C. (2009). Segmen-
tation of 3D Lidar Data in non-flat Urban Environ-
ments using a Local Convexity Criterion. In Proc. of
the IEEE Intelligent Vehicles Symposium (IV), pages
215–220, Nashville, Tennessee, USA.
CLASSIFICATION OF 3D URBAN SCENES - A Voxel based Approach
69