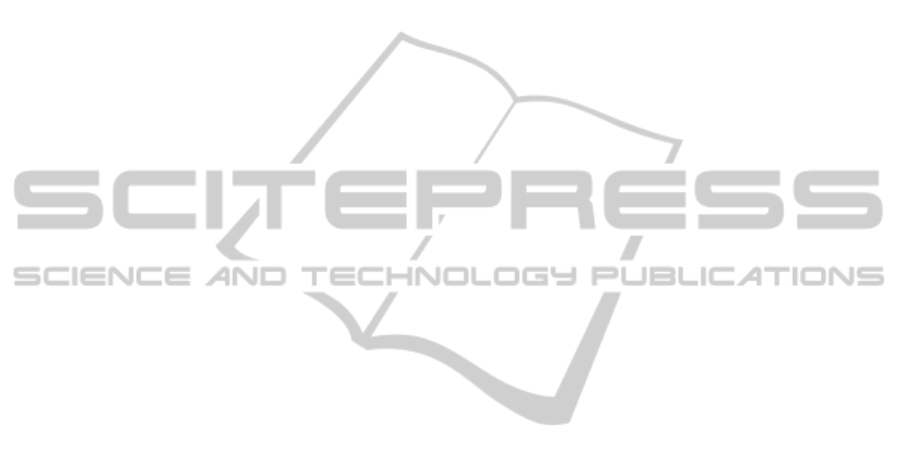
requiring different service types, each provided by
distinct servers, are accommodated in one common
queue. We have proposed a dual-class, two-server
queueing model with class-dedicated servers in dis-
crete time, operating under the global FCFS service
discipline, assuming independent arrivals from slot to
slot with a simple first-order Markovian class cluster-
ing model. The model is relatively simple so as to
allow for an analytical solution, but yet contains all
the important elements needed for a conceptual study
of the effect of “global FCFS” on this type of queue.
We emphasize that we have succeeded in deriving an
explicit formula for the pgf of the system occupancy,
under general assumptions with respect to the arrival
statistics. For the special case of geometric arrivals,
we have even been able to obtain explicit closed-form
expressions for the pmf’s, the mean values and the tail
distributions of system occupancy. The results reveal
the very direct and great influence of the degree of
“class clustering” in the arrival stream on the stability
and the performance of the system. We believe that
this is the main qualitative conclusion of the study.
In general, only few studies have focused on the
phenomenon of class clustering in the context of mul-
ticlass queueing systems, and this paper shows that
the effect of class clustering may be very impor-
tant, possibly not only in queues with class-dependent
servers and global FCFS, but also in other queueing
situations whereby the service mechanism is sensi-
tive to the order of service of customers of different
classes. For instance, we expect that class clustering
may also have substantial effects on the performance
of priority queues or queues whereby the lengths of
the service times depend on the way customers of dif-
ferent types succeed each other.
The model examined in this paper can be gen-
eralized in various directions. To start with, more
general service-time distributions can be considered
than the simple deterministic one-slot-per-customer
model studied in this paper. The extra difficulty is
that two customers of different types do not necessar-
ily leave the system in order of their arrival anymore,
exactly due to the variable service times. We note
that the simplest model in continuous time with ex-
ponential service times already has this difficulty (see
e.g. (M´elange et al., 2011)). Also, the assumption
of independent arrivals from slot to slot may be re-
laxed to allow for correlated or bursty types of arrival
processes. Depending on the precise details of the
class clustering model used, this may also affect the
performance of the system considerably. Finally, we
may consider more complicated models for the class
clustering mechanism than the simple one-parameter
model used in this paper. As already touched upon
in section 2, the current study is restricted to systems
whereby both customer classes are equiprobable and
the probability of having a next customer of the same
(or opposite) type as the previous one does not de-
pend on the type of the previous customer. Many dif-
ferent types of more general models than this can be
envisaged. For instance, the types of consecutive cus-
tomers in the arrival stream could be modeled as a
two-state Markov chain with general transition prob-
abilities, or the sizes of subsequent clusters of cus-
tomers of each type could be described by general
(rather than geometric) probability distributions, and
so on. We plan to tackle several of these generaliza-
tions in future work.
REFERENCES
Beekhuizen, P. and Resing, J. (2009). Performance analysis
of small non-uniform packet switches. Performance
Evaluation, 66:640–659.
Bruneel, H. and Kim, B. (1993). Discrete-time models for
communication systems including ATM. Kluwer Aca-
demic, Boston, USA.
Fiems, D. and Bruneel, H. (2002). A note on the discretiza-
tion of Little’s result. Operations Research Letters,
30:17–18.
Gonz´ales, M. (1992). Classical complex analysis. Marcel
Dekker, New York, USA.
Gouweleeuw, F. and Tijms, H. (1998). Computing loss
probabilities in discrete-time queues. Operations Re-
search, 46:149–154.
Karol, M., Hluchyj, M., and Morgan, S. (1987). Input ver-
sus output queueing on a space-division packet switch.
IEEE Transactions on Communications, 35:1347–
1356.
Kim, H. and Schroff, N. (2001). Loss probability calcu-
lations and asymptotic analysis for finite buffer mul-
tiplexers. IEEE/ACM Trans. on Networking, 9:755–
768.
Kleinrock, L. (1975). Queueing systems, part I. Wiley, New
York, USA.
Laevens, K. (1999). A processor-sharing model for input-
buffered ATM-switches in a correlated traffic environ-
ment. Microprocessors and Microsystems, 22:589–
596.
Liew, S. (1994). Performance of various input-buffered and
output-buffered ATM switch design principles under
bursty traffic: simulation study. IEEE Transactions on
Communications, 42:1371–1379.
Mandelbaum, A. and Reiman, M. (1998). On pooling in
queueing networks. Management Science, 44:971–
981.
M´elange, W., Bruneel, H., Steyaert, B., and Walraevens, J.
(2011). A two-class continuous-time queueing model
with dedicated servers and global fcfs service disci-
pline. In Analytical and Stochastic Modeling Tech-
niques and Applications, volume 6751 of Lecture
IMPACT OF BLOCKING WHEN CUSTOMERS OF DIFFERENT CLASSES ARE ACCOMMODATED IN ONE
COMMON QUEUE
37