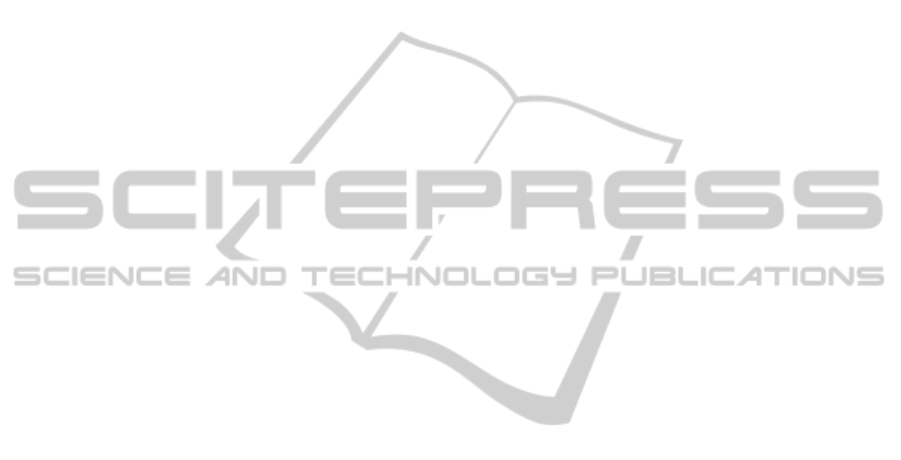
put. We believe that our approach of interactive topic
graph extraction and exploration, together with its im-
plementation on a mobile device, helps users explore
and find new interesting information on topics about
which they have only a vague idea or even no idea at
all.
Our next future work will consider the integration
of open shared knowledge bases into the learn search
activity, e.g., Wikipedia or other similar open web
knowledge sources and the extraction of relations,
and finally to merge information from these different
resources. We already have embedded Wikipedia’s
infoboxes as background knowledge but not yet in-
tegrated them into the extracted web topic graphs,
cf. (Neumann and Schmeier, 2011) for some more
details. If so done, we will investigate the role of
Wikipedia and the like as a basis for performing dis-
ambiguation of the topic graphs. For example, cur-
rently, we cannot distinguish the associated topics ex-
tracted for a query like “Jim Clark” whether they are
about the famous formula one racer or the Netscape
founder or even about another person.
In this context, the extraction of semantic relations
will be important. Currently, the extracted topic pairs
only express certain semantic relatedness, but the na-
ture and meaning of the underlying relationship is un-
clear. We have begun investigating this problem by
extending our approach of chunk–pair–distance ex-
traction to the extraction of triples of chunks with al-
ready promising initial results.
ACKNOWLEDGEMENTS
The presented work was partially supported by grants
from the German Federal Ministry of Economics and
Technology (BMWi) to the DFKI THESEUS project
(FKZ: 01MQ07016).
REFERENCES
Banko, M., Cafarella, M. J., Soderland, S., Broadhead, M.,
and Etzioni, O. (2007). Open information extraction
from the web. In Proceedings of IJCAI–2007, pp
2670–2676.
Baroni, M. and Evert, S. (2008). Statistical methods for
corpus exploitation. In A. L¨udeling and M. Kyt¨o
(eds.), Corpus Linguistics. An International Hand-
book, Mouton de Gruyter, Berlin.
Dingare, S., Nissim, M., Finkel, J., Grover, C., and Man-
ning, C. D. (2004). A system for identifying named
entities in biomedical text: How results from two eval-
uations reflect on both the system and the evaluations.
In Comparative and Functional Genomics 6:pp 77-85.
Drozdzynski, W., Krieger, H.-U., Piskorski, J., Sch¨afer, U.,
and Xu, F. (2004). Shallow processing with unifica-
tion and typed feature structures — foundations and
applications. K¨unstliche Intelligenz, pages 17–23.
Etzioni, O. (2007). Machine reading of web text. In
Proceedings of the 4th international Conference on
Knowledge Capture, Whistler, BC, Canada, pp 1-4.
Geraci, F., Pellegrini, M., Maggini, M., and Sebastiani, F.
(2006). Cluster generation and labeling for web snip-
pets: A fast, accurate hierarchical solution. Journal of
Internet Mathematics, 4(4):413–443.
Giesbrecht, E. and Evert, S. (2009). Part-of-speech tagging
- a solved task? an evaluation of pos taggers for the
web as corpus. In Proceedings of the 5th Web as Cor-
pus Workshop.
Gimenez, J. and Marquez., L. (2004). Svmtool: A gen-
eral pos tagger generator based on support vector ma-
chines. In Proceedings of LREC’04, pp. 43 - 46.
Manning, C. D., Raghavan, P., and Sch¨utze, H. (2008). In-
troduction to information retrieval. In Cambridge Uni-
versity Press.
Marchionini, G. (2006). Exploratory search: from finding
to understanding. Commun. ACM, 49(4):41–46.
Nadeau, D. and Sekine, S. (2007). A survey of named entity
recognition and classification. Journal of Linguisticae
Investigationes, 30(1):1–20.
Neumann, G. and Schmeier, S. (2011). A mobile touchable
application for online topic graph extraction and ex-
ploration of web content. In Proceedings of the ACL-
HLT 2011 System Demonstrations.
Osinski, S., Stefanowski, J., and Weiss, D. (2004). Lingo:
Search results clustering algorithm based on singular
value decomposition. In Proceedings of the Inter-
national IIS: Intelligent Information Processing and
Web Mining Conference. Advances in Soft Computing,
Springer.
Osinski, S. and Weiss, D. (2008). Carrot2: Making sense of
the haystack. In ERCIM News.
Turney, P. (2001). Mining the web for synonyms: PMI-IR
versus LSA on TOEFL. In Proceedings of ECML–
2002. Freiburg, Germany, pp 491-502.
Yates, A. (2007). Information extraction from the web:
Techniques and applications. In Ph.D. Thesis, Uni-
versity of Washington, Computer Science and Engi-
neering.
EXPLORATORY SEARCH ON THE MOBILE WEB
91