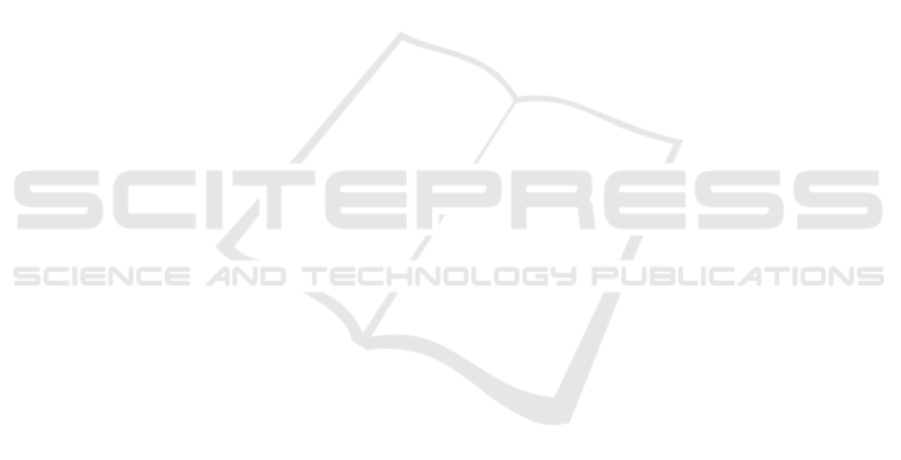
ECONOMIC-PROBABILISTIC MODEL FOR RISK ANALYSIS
IN PROJECTS
Rogério Feroldi Miorando, José Luis Duarte Ribeiro, Maria A. C. Tinoco
and Carla Schwengber ten Caten
Federal University of Rio Grande do Sul, Porto Alegre, Brasil
Keywords: Risk analysis, Project management, Simulation.
Abstract: This paper presents an economic-probabilistic model to conduct risk analysis in projects. The model
integrates risk and economic project analysis by quantifying both value and probability of occurrence of
potential cash flow deviations, thus resulting in an economic-probabilistic analysis of the expected returns.
The model allows calculating risk-adjusted values for cash flow groups and projecting net present value
through stochastic simulation. As a result, the model provides both the risk-adjusted project economic return
with the associated probability distribution to its NPV and the variability that each risk factor generates in
the project return.
1 INTRODUCTION
Risk analysis is growing in importance in the current
economy, as most economic decisions are taken in
uncertainty-prone scenarios. Uncertainty sources are
multiple and extensive, encompassing risks
associated to markets, suppliers, weather,
technology, etc. (Chavas, 2004).
Among the risk analysis models for project
available in academic literature, few directly indicate
the actual risks. Moreover, among the models that
propose specific tools for risk analysis, most focus
only on the success probability for the overall
project itself, without considering their economic
dimension. There are also models, such as
Benaroch’s (2002, 2007), that use real options to
value IT projects given the assumed risks, but these
models only conduct economic analysis and do not
face the problem of identifying and quantifying the
risks involved.
The objective of this paper is to present the
application of an economic-probabilistic model for
analysing risks in project investments. The model
integrates risk and economic project analysis by
quantifying both value and probability of occurrence
of potential cash flow deviations, thus resulting in an
economic-probabilistic analysis of the expected
returns.
2 MODEL STRUCTURE
The model presented in this paper was based on the
models by Karolak (1996), Foo and Murugananthan
(2000) and Schimitz et al. (2006). The application of
the model is conducted according to four steps: cash
flow structure completion; risk assessment structure
completion; determination of cash flow group risk-
adjusted Net Present Value (NPV); and
determination of risk-adjusted Net Present Value for
the project.
In the step of cash flow structure completion, the
benefit and cost items are distributed among nine
cash flow groups. The groups are divided as follows:
(i) Benefits, (ii) Financial costs, (iii) Infrastructure,
(iv) Licensing and equipment, (v) Labor, (vi)
Training, (vii) Outsourced services, (viii)
Consumables and (ix) Other expenses.
The risk assessment structure completion is
carried out through a questionnaire that combines
the categories of risk with the project's cash flow.
Risk categories and associated risk factors were
identified through literature analysis coupled with
expert opinions. The questionnaire is composed of
six fields (Figure 1): (i) matching of cash flow
groups and risk categories; (ii) assessment of risk
factor impact; (iii) assessment of probability of
occurrences for the risk factors’ impact ranges; (iv)
indication of analysts’ knowledge level about each
risk factor assessment; (v) indication of reasoning
208
Feroldi Miorando R., Luis Duarte Ribeiro J., A. C. Tinoco M. and Schwengber ten Caten C..
ECONOMIC-PROBABILISTIC MODEL FOR RISK ANALYSIS IN PROJECTS.
DOI: 10.5220/0003737302080211
In Proceedings of the 1st International Conference on Operations Research and Enterprise Systems (ICORES-2012), pages 208-211
ISBN: 978-989-8425-97-3
Copyright
c
2012 SCITEPRESS (Science and Technology Publications, Lda.)