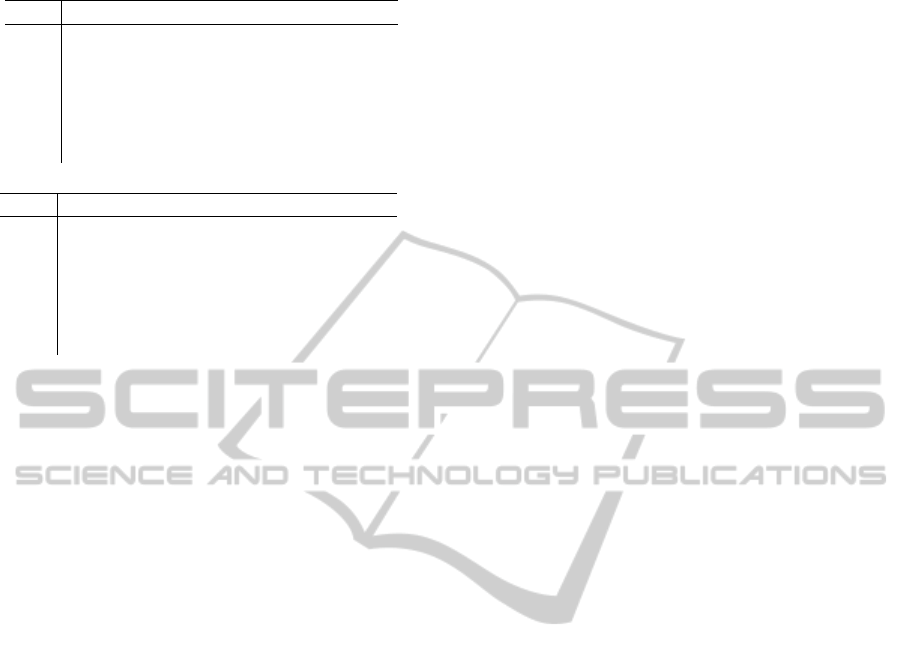
Table 4: Scores of Humans and Artificial Agent.
e j = 1 j = 2 j = 3 j = 4 j = 5
B1a 8.25 8.53 6.26 8.35 8.11
B1b 6.25 8.02 6.29 7.91 7.44
B1c 7.65 7.84 2.78 8.1 7.7
B2a 2.48 6.43 7.33 7.98 7.53
B2b 4.61 6.08 5.43 2.85 5.13
B2c 1.23 2.75 0.16 3.92 6.58
e j = 6 j = 7 j = 8 artificial agent
B1a 8.54 6.02 7.55 8.27
B1b 7.56 6.9 5.87 7.37
B1c 6.85 7.23 -2.06 7.4
B2a 5.79 4.63 5.66 6.7
B2b 2.7 4.6 0.2 5.62
B2c 2.44 -3.5 4.32 4.89
choice of financial position. Table 3 shows how Z
and m increases with the standard deviations. The be-
havior of the parameters which regulate the financial
decision also changes with the level of standard devi-
ations. For low levels of σ and
˜
σ (experiments B1a
and B1b) the values of these parameters are irrelevant
(∀ symbol). When the standard deviations have inter-
mediate levels (experiments B1c and B2a) the value
of h is high while that of λ is low. h decreases and
λ increases at high levels of the standard deviations
(experiments B2b and B2c).
To give a summary of the work done in this pa-
per, we report the scores (equation 3) of humans and
artificial agent in Table 4. The performance of the
artificial agent is in line with the average score of hu-
mans in the initial four experiments. In experiments
B2b and B2c, the artificial agent performs better than
humans, however a number of the latter still perform
similarly (or better) than the artificial agent.
6 CONCLUSIONS
In this paper we take the first step of a project aimed at
assessing Minsky’s FIH. Our aim is to create avatars
whose behavior mimic that of humans.
Here we focus on entrepreneurs whose behavior is
the cornerstone of FIH. A result of our analysis is that,
beside the traditional approach which assumes ratio-
nal maximizing subjects, the heuristic approach could
also provide for a valid alternative micro-foundation
for the financial decisions of entrepreneurs. A sec-
ond point worth to be mentioned probably provides
an element of novelty in the interpretation of Min-
sky’s thought: financial behaviors could mainly de-
pend on the volatility of demand and on the accuracy
of demand forecasts instead of depending on the busi-
ness cycle phases as usually pointed out by models
inspired by Minsky’s economic thought
The potential future developments of our model
are many. First of all, the agents’ choice under dif-
ferent assumptions on divisibility and reversibility of
investment could be analyzed. Second, a statistical
analysis of experimental data could lead us to iden-
tify a number of heterogeneous avatars. The third ex-
tension concerns interaction. It could be investigated
how the results change when selected information on
the other subjects are given to each experimental sub-
ject, and how the situation evolves when credit de-
mands are selected by a real life banker.
The final goal of our project is to build a multi-
agent artificial system made up of a large number of
different types of avatars interacting with each other
and with banks. The model could be used for mon-
itoring how the situation evolves at macroeconomic
level and to verify in what extend the FIH could be
judged as valid.
REFERENCES
Bagliano, F.-C. and Bertola, G. (2004). Models for Dynamic
Macroeconomics. Oxford University Press.
Gigerenzer, G., Hertwig, R., and Pachur, T., editors (2011).
Heuristics: The Foundations of Adaptive Behavior.
Oxford University Press, New York.
Giulioni, G. (2011). A Software to Generate Evenly Spaced
Points on the Unit N-Simplex. SSRN eLibrary. Avail-
able at
http://ssrn.com/paper=1822002
.
Koeva, P. (2009). Time-to-build and convex adjustment
costs. International Monetary Fund. Working Paper
n. 1.
Minsky, H. P. (1974). The modeling of financial instabil-
ity: an introduction. In Modelling and Simulation.
Proceedings of the 5th Annual Pittsburg Conference,
pages 267–272.
Minsky, H. P. (1975). John Maynard Keynes. Columbia
University Press, New York.
Minsky, H. P. (1982). Can ‘It’ Happen Again? Essays on
Instability and Finance. M.E. Sharpe, New York.
Minsky, H. P. (1986). Stabilizing an Unstable Economy.
Yale University Press, New Haven and London.
Schelling, T. C. (1960). The Strategy of Conflict. Harvard
University Press.
Storm, R. and Price, K. (1997). Differential evolution - a
simple and efficient heuristic for global optimization
over continuous spaces. Journal of Global Optimiza-
tion, 11(4):341–359.
Zuckerman, I., Kraus, S., and Rosenschein, J. S. (2007). Us-
ing focal point learning to improve tactic coordination
in human-machine interactions. In IJCAI’07, pages
1563–1569.
EXPLORING FIRMS FINANCIAL DECISIONS BY HUMAN AND ARTIFICIAL AGENTS - Towards an Assessment of
Minsky's Financial Instability Hypothesis
189