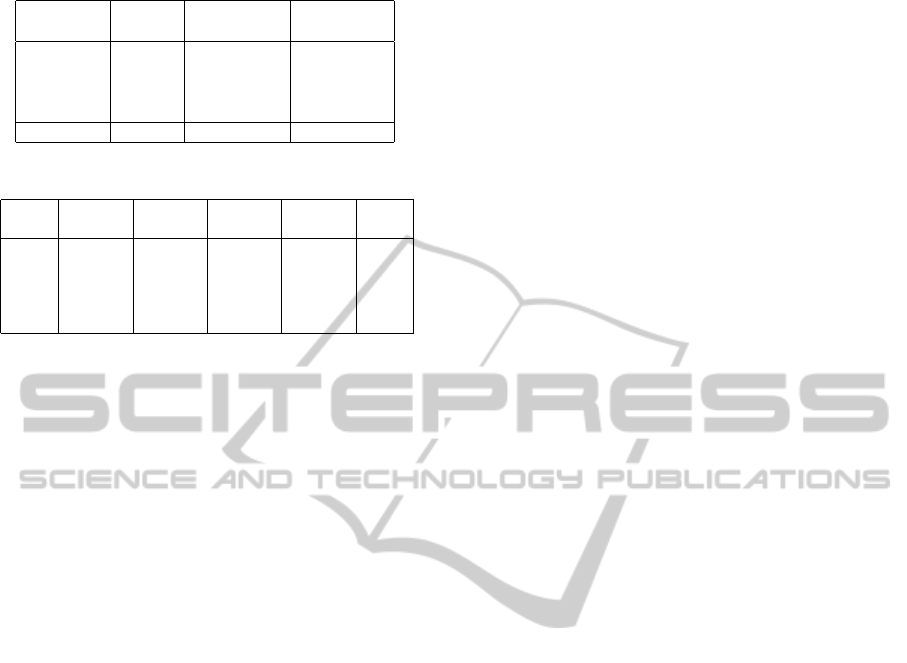
Table 1: Summary of the 4-cross validation.
Cross Hits Room level Mean error
validation (%) (%) (m)
1st 88.75 92.50 0.77
2nd 86.25 100.00 0.79
3rd 97.50 100.00 0.71
4th 95.00 95.00 0.73
Mean 91.875 96.875 0.75
Table 2: Number of misclassifications by zone.
Cross- Cross- Cross- Cross-
Zone valid. 1 valid. 2 valid. 3 valid. 4 Total
c1 3 4 2 - 9
c3 - 1 - - 1
l1 2 - - - 2
n2 4 4 - 4 12
s1 - 2 - - 2
is 91.875% for the 4-cross validations, as shown in
Table 1.
Following the method for the mean error calcula-
tion developed by us in previous work (Orozco-Ochoa
et al., 2011) and based on the results from Table 2, an
average error of 0.75 meter was obtained. The mean
error is below other researchers’ mean error, which is
also around 2 meters, as has been shown in section 2.
5 CONCLUSIONS AND FUTURE
WORK
This paper describes an algorithm suitable for indoor
location based on a Bayesian classifier and Weibull
distribution. The results are very promising, better
than those of the literature.
This method would allow the monitoring of el-
derly people at home, not only the room where the
person is at each moment, but also the area within the
room where the person is. In this way, we can try
to identify the actions he is performing (sitting on the
couch, standing by the window, etc). We plan to study
the problem detected in areas close to a BS. A possi-
ble fix may be changing the type of filter used for re-
moving the reflected waves. Eventually, we will work
on the integration of our algorithm with multisenso-
rial and intelligent monitoring system: a system that
includes the use of video cameras and other sensors.
ACKNOWLEDGEMENTS
This work was supported by the Xunta de Galicia un-
der grant 08SIN002206PR.
REFERENCES
Aparicio, S., P
´
erez, J., Tarr
´
ıo, P., Bernardos, A., and Casar,
J. (2008). An indoor location method based on a fu-
sion map using bluetooth and wlan technologies. Int.
Symp. on Distributed Computing and Artificial Intel-
ligence (DCAI), 50:702–710.
Figueiras, J., Schwefel, H.-P., and Kovacs, I. (2005). Accu-
racy and timing aspects of location information based
on signal-strength measurements in bluetooth. IEEE
16th Int. Symp. on Personal, Indoor and Mobile Radio
Communications (PIMRC, 4:2685–2690.
Forno, F., Malnati, G., and Portelli, G. (2005). Design and
implementation of a bluetooth ad hoc network for in-
door positioning. IEE Proc. Software, 152(5):223–
228.
Fritsche, C. and Klein, A. (2009). On the performance of
hybrid gps/gsm mobile terminal tracking. IEEE Int.
Conf. on Comm. Workshops (ICC Workshops), pages
1–5.
G
´
omez-Conde, I., Olivieri-Cecchi, D., Vila-Sobrino, X.,
and Orosa-Rodr
´
ıguez, A. (2011). Intelligent video
monitoring for anomalous event detection. 2nd Int.
Symp. on Ambient Intelligence (ISAmI), 92:101–108.
Gu, Y., Lo, A., and Niemegeers, I. (2009). A survey of
indoor positioning systems for wireless personal net-
works. IEEE Comm. Surveys Tutorials, 11(1):13–32.
Hossain, A. M., Van, H. N., Jin, Y., and Soh, W. (2007).
Indoor localization using multiple wireless technolo-
gies. IEEE Int. Conf. on Mobile Adhoc and Sensor
Systems, pages 1–8.
Liu, H., Darabi, H., Banerjee, P., and Liu, J. (2007).
Survey of wireless indoor positioning techniques
and systems. IEEE Transactions on Systems, Man
and Cybernetics, Part C (Applications and Reviews),
37(6):1067–1080.
Nurmi, P., Bhattacharya, S., and Kukkonen, J. (2010). A
grid-based algorithm for on-device gsm positioning.
Proc. of the 12th ACM Int. Conf. on Ubiquitous Com-
puting, pages 227–236.
Orozco-Ochoa, S., Vila-Sobrino, X., Rodr
´
ıguez-Dami
´
an,
M., and Rodr
´
ıguez-Li
˜
nares, L. (2011). Bluetooth-
based system for tracking people localization at home.
Int. Symp. on Distributed Computing and Artificial In-
telligence (DCAI), 91:345–352.
Pei, L., Chen, R., Liu, J., Tenhunen, T., Kuusniemi, H., and
Chen, Y. (2010). An inquiry-based bluetooth indoor
positioning approach for the finnish pavilion at shang-
hai world expo 2010. Proc. of IEEE/ION PLANS,
pages 1002–1009.
Vaughan-Nichols, S. (2009). Will mobile computing’s
future be location, location, location? Computer,
42(2):14–17.
AN INDOOR LOCALIZATION ALGORITHM BASED IN WEIBULL DISTRIBUTION AND BAYESIAN
CLASSIFIER
341