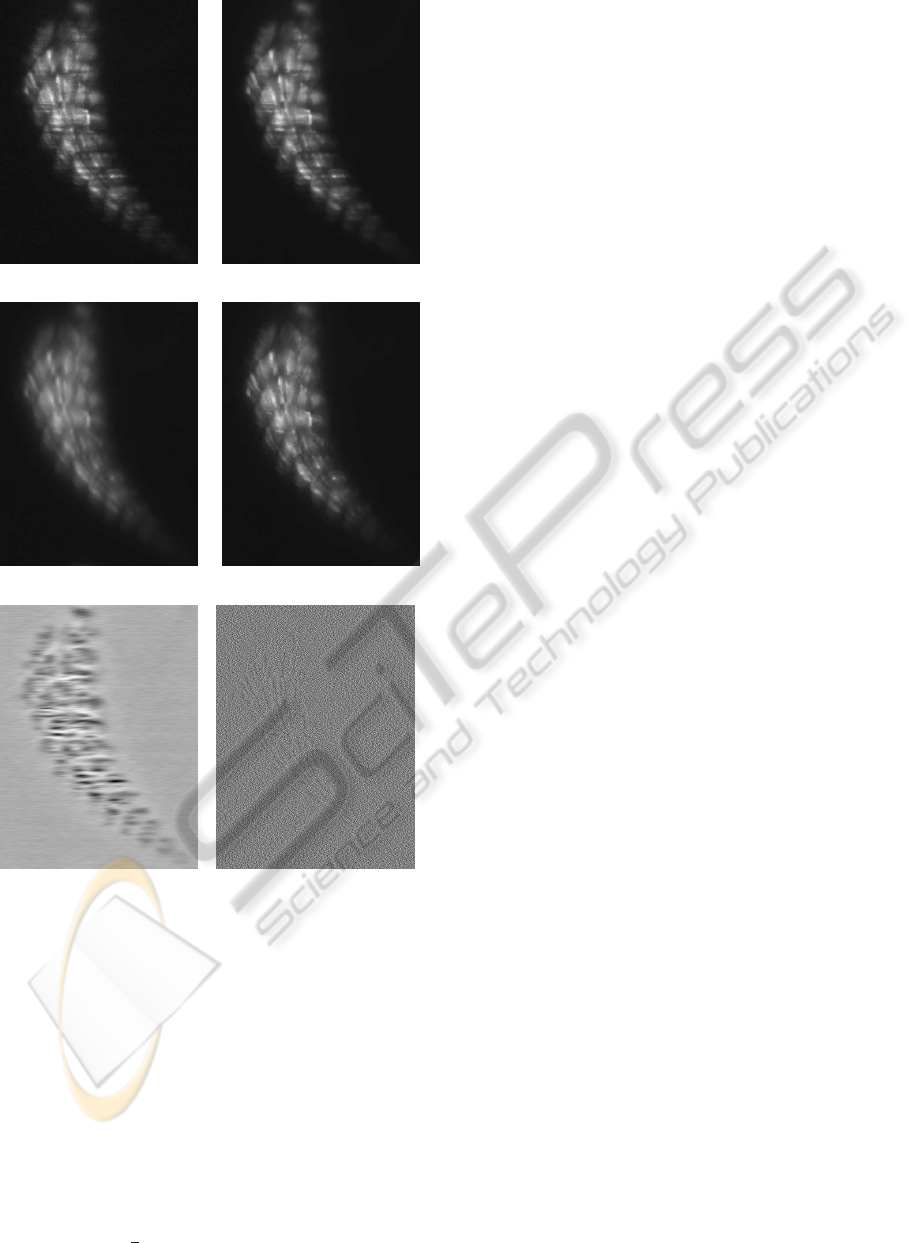
(a) (d)
(b) (e)
(c) (f)
Figure 5: Top-Left: original image zebrafish embryo
Tg.SMYH1:GFP Slow myosin Chain I specific fibers - Top-
Right: TV-L2 denoising - Mid-Left: H
1
-Gabor restoration
- Mid-Right: TV-Gabor restoration - Bottom-Left: stripes
identified by our algorithm - Bottom-Right: white noise.
The original image is presented in Figure 5(a), and
the result of Algorithm 1 is presented in Figure 5(e).
We also present a comparison with two other algo-
rithms in Figures 5(d,b):
• a standard TV-L
2
denoising algorithm. The algo-
rithm is unable to remove the stripes as the prior
is unadapted to the noise.
• an “H
1
-Gabor” algorithm which consists in set-
ting F(·) =
1
2
k · k
2
2
in equation (2). The image
prior thus promotes smooth solutions and pro-
vides blurry results.
ACKNOWLEDGEMENTS
The authors wish to thank Julie Batut for providing
the images of the zebrafish. They also thank Val´erie
Lobjois, Bernard Ducommun, Raphael Jorand and
Franc¸ois De Vieilleville for their support during this
work.
REFERENCES
Aujol, J.-F., Gilboa, G., Chan, T., and Osher, S. (2006).
Structure-texture image decomposition - modeling, al-
gorithms, and parameter selection. Int. J. Comput. Vi-
sion, vol. 67(1), pp. 111-136.
Chambolle, A. and Pock, T. (2011). A first-order primal-
dual algorithm for convex problems with applications
to imaging. J. Math. Imaging Vis. 40(1): 120-145.
Fadili, M., Starck, J.-L., Bobin, J., and Moudden, Y. (2010).
Image decomposition and separation using sparse rep-
resentations: an overview. Proc. of the IEEE, Spe-
cial Issue: Applications of Sparse Representation, vol.
98(6), pp. 983-994,.
Garnett, J., Le, T., Meyer, Y., and Vese, L. (2007). Image
decompositions using bounded variation and general-
ized homogeneous besov spaces. Appl. Comput. Har-
mon. Anal., 23, pp. 25-56.
Huisken, J., Swoger, J., Bene, F. D., Wittbrodt, J., and
Stelzer, E. (2004). Optical sectioning deep inside live
embryos by selective plane illumination microscopy.
Science, vol. 305, 5686, p.1007.
Kowalski, M. (2009). Sparse regression using mixed norms.
Appl. Comput. Harmon. A. 27, 3, 303–324.
Meyer, Y. (2001). Oscillating patterns in image process-
ing and in some nonlinear evolution equations, in 15th
Dean Jacqueline B. Lewis Memorial Lectures. AMS.
Ng, M., Weiss, P., and Yuan, X.-M. (2010). Solving con-
strained total-variation image restoration and recon-
struction problems via alternating direction methods.
SIAM Journal on Scientific Computing, 32.
Osher, S., Sole, A., and Vese, L. (2003). Image decomposi-
tion and restoration using total variation minimization
and the h
−1
norm. SIAM Multiscale Model. Sim. 1(3),
pp. 339-370.
Rockafellar, T. (1970). Convex Analysis. Princeton Univer-
sity Press.
Shiryaev, A. (1996). Probability, Graduate Texts in Mathe-
matics 95. Springer.
Starck, J., Elad, M., and Donoho, D. (2005). Image decom-
position via the combination of sparse representations
and a variational approach. IEEE Trans. Im. Proc.,
vol. 14(10).
Vese, L. and Osher, S. (2003). Modeling textures with total
variation minimization and oscillating patterns in im-
age processing. J. Sci. Comput., 19(1-3), pp. 553-572.
ICPRAM 2012 - International Conference on Pattern Recognition Applications and Methods
342