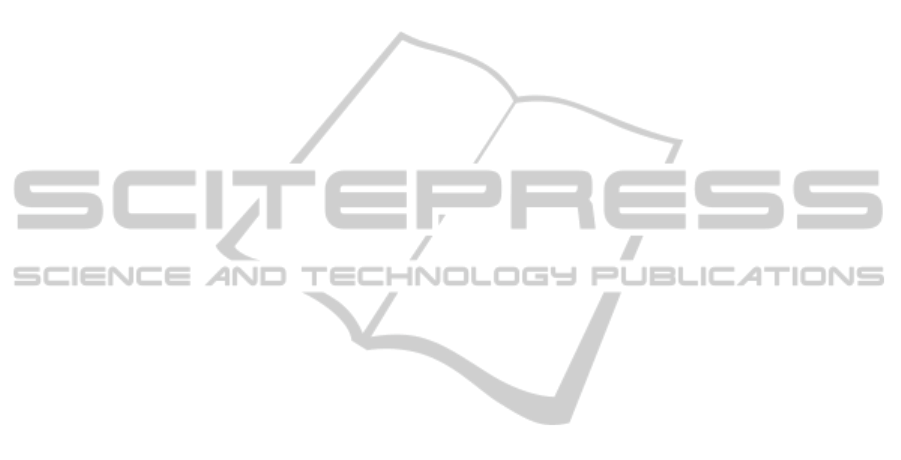
a seizure occurs, all the tracks are labeled as
epileptic. The test set consists also of 100 normal
motion tracks and 67 epileptic motion tracks from 5
myoclonic seizures. For the time being, the
classification is based on the single tracks, so not on
the whole seizures, thus we have 113 positive
samples in the training set and 67 in the test set, each
of them being labeled as part of a myoclonic shock.
After training the linear SVM model once, we
tested it on our test set which resulted in 54 true
positives, 67 true negatives, 33 false positives and
13 false negatives. This corresponds to a sensitivity
of 80.60%, a Positive Predictive Value of 62.07%
and a specificity of 67.00%.
4 DISCUSSION
The classification is carried out on individual motion
tracks. The motion tracks extracted during an
epileptic shock are possibly not all from the epileptic
movement itself, but also from e.g. movements of
the bed because of the seizure. So the classification
can be improved on this point. Moreover, the
features from different tracks originating from one
seizure can be combined, to further improve the
detection.
The training and testing is now performed on a
small dataset. To have more solid validation, a larger
dataset should be used. These results are preliminary
but give an indication that the detection of specific
types of seizures by the proposed algorithm is
possible.
The obtained results in this paper are less optimal
than in (Karayiannis et al., 2006), namely a
sensitivity of 80.60% and a specificity of 67.00%
compared to a sensitivity and specificity above 90%
in (Karayiannis et al., 2006). But the circumstances
in our setup were more difficult as the patients’ body
parts are most of the time not clearly visible.
Removing the blankets is not an option as it would
reduce the sleeping quality of the patients too much.
But notice that there is still some room for
improvement in our algorithm.
5 CONCLUSIONS
The detection of seizures based on motion tracks
extracted from the optical flow calculation and the
mean shift clustering algorithm is possible. In the
first test on 15 myoclonic shocks a sensitivity of
80.60% and a positive predictive value of 62.07% is
reached. Further research is required to confirm
these first results and to test the algorithm on other
seizures.
ACKNOWLEDGEMENTS
Research supported by Research Council KUL:
GOA-MANET, IWT: TBM070713-Accelero,
Belgian Federal Science Policy Office IUAP P6/04
(DYSCO, ‘Dynamical systems, control and
optimization, 2007–2011); EU: Neuromath
(COSTBM0601). Kris Cuppens is funded by a Ph.D
grant of the Institute for the Promotion of Innovation
through Science and Technology in Flanders (IWT-
Vlaanderen)
REFERENCES
Karayiannis N. B., Xiong Y., Tao G., Frost J. D. Jr., Wise
M. S., Hrachovy R. A. and Mizrahi E. M., Automated
detection of videotaped neonatal seizures of epileptic
origin. Epilepsia, vol. 47, pp. 966-980, 2006.
Min J. H., Kasturi R. and Camps O., Extraction and
temporal segmentation of multiple motion trajectories
in human motion. Image and Vision Computing, vol.
26, pp. 1621-1635, 2008.
Crocker J. C. and Grier D. G., Methods of digital video
microscopy for colloidal studies. Journal of Colloid
and Interface Science, vol. 179, pp. 298-310, 1996.
Yilmaz A., Javed O. and Shah M., Object tracking: A
survey. Acm Computing Surveys, vol. 38, 2006.
Horn B. K. P. and Schunck B. G., Determining Optical-
Flow. Artificial Intelligence, vol. 17, pp. 185-203,
1981.
Cuppens K., Lagae L., Ceulemans B., Van Huffel S. and
Vanrumste B., Automatic video detection of body
movement during sleep based on optical flow in
pediatric patients with epilepsy. Medical & biological
engineering & computing, vol. 48, N° 9, pp. 923-931,
2010.
Cheng Y. Z., Mean Shift, Mode Seeking, And Clustering.
IEEE Transactions on Pattern Analysis and Machine
Intelligence, vol. 17, pp. 790-799, 1995.
Fukunaga K. and Hostetler L. D., Estimation Of Gradient
Of A Density-Function, With Applications In Pattern-
Recognotion. IEEE Transactions on Information
Theory, vol. 21, pp. 32-40, 1975.
Hu W. M., Tan T. N., Wang L., Maybank S., A survey on
visual surveillance of object motion and behaviors.
IEEE Transactions on Systems Man and Cybernetics
Part C-Applications and Reviews, vol. 34, pp. 334-
352, 2004.
Turaga P., Chellappa R., Subrahmanian V. S., Udrea O.,
Machine Recognition of Human Activities: A Survey.
IEEE Transactions on Circuits and Systems for Video
Technology, vol. 18, pp. 1473-1488, 2008.
AUTOMATIC VIDEO DETECTION OF NOCTURNAL EPILEPTIC MOVEMENT BASED ON MOTION TRACKS
345