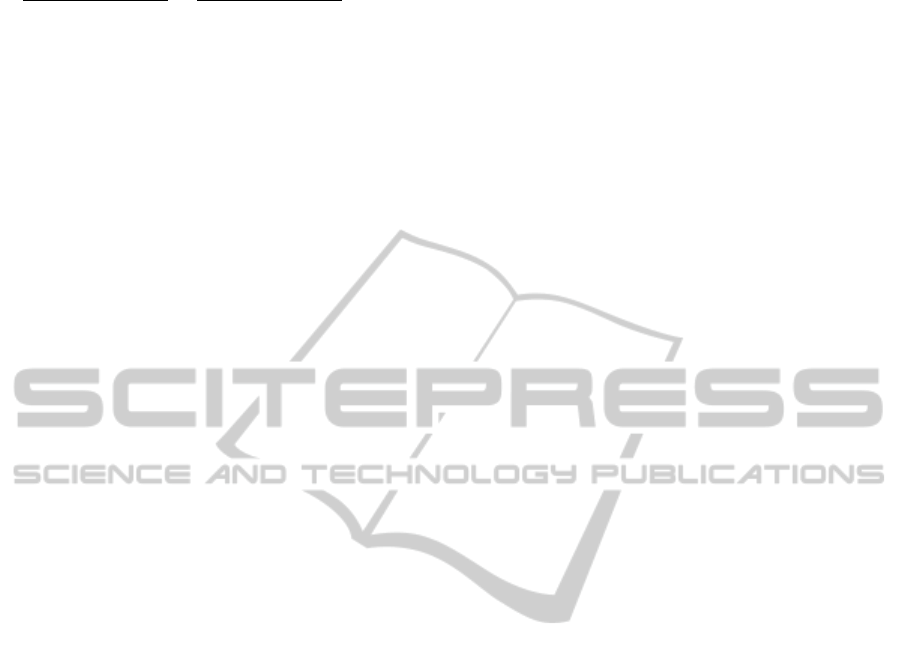
p
opt
− p
relaxCPLEX
p
opt
− p
relaxSDP
>
p
′
opt
− p
relaxCPLEX
p
′
opt
− p
relaxSDP
(19)
On average, the gap improves from 1.80% to
1.71% with original SDP relaxation and 1.56% with
addition of valid equalitites. This latter improve-
ment is promising, even though it comes at high ad-
ditional computational cost, particularly on the larger
instances. This can be ascribed to the fact that SDP
solvers are only in their infancy, especially compared
to a commercial solver like CPLEX.
Finally, the randomized rounding yields satisfying
results: due to the random aspect of the procedure,
there are still some data set where the continuous re-
laxation gives better results than the semidefinite re-
laxation, but on average the loss of optimality reduces
from 7.75% to 6.41% and 5.59%, which is significant
when considering the huge amount at stake.
5 CONCLUSIONS AND
PROSPECTS
We investigated in this paper, semidefinite relaxations
for a MIQP (Mixed-Integer Quadratic Program) ver-
sion of the scheduling of nuclear power plants out-
ages. Comparison of the results obtained on signifi-
cant data sets shows the following main results. First,
our MIQP is extremely hard to solve with CPLEX.
Second, semidefinite relaxations provide a tighter
convex relaxation than the continuous relaxation. In
our experiments the gap between the optimal solu-
tion and the continuous relaxation is on average equal
to 1.80% whereas the semidefinite relaxation yields
an average gap of 1.56%. Third, the computational
time for computing these semidefinite relaxations is
reasonable. Exploiting those results in a randomized
rounding procedure instead of the result of the contin-
uous relaxation leads to a significant improvement of
the feasible solution.
In the view of these preliminary results, additional
investigations will concern i) introduction of more
valid inequalities, ii) evaluation of others SDP resolu-
tion techniques, for instance Conic Bundle for facing
problems of huge size.
REFERENCES
Borchers, B. (1999). Csdp, a C library for semidefinite pro-
gramming.
Helmberg, C. and Rendl, F. (2000). A spectral bundle
method for semidefinite programming. SIAM Journal
on Optimization, 10(3):673–696.
Helmberg, C., Rendl, F., Vanderbei, R. J., and Wolkowicz,
H. (1996). An interior-point method for semidefinite
programming. SIAM Journal on Optimization, 6:342–
361.
Khemmoudj, M. O. I., Bennaceur, H., and Porcheron,
M. (2006). When constraint programming and local
search solve the scheduling problem of electricit´e de
france nuclear power plant outages. In 12th Interna-
tional Conference on Principles and Practice of Con-
straint Programming (CP’06), pages 271–283. LNCS.
Laurent, M., , , and Rendl, F. (2005). Semidefinite program-
ming and integer programming. In K. Aardal, G. N.
and Weismantel, R., editors, Handbook on Discrete
Optimization, pages 393–514.
Porcheron, M., Gorge, A., Juan, O., Simovic, T., and
Dereu, G. (2009). Challenge roadef/euro 2010: A
large-scale energy management problem with var-
ied constraints. Technical report, EDF R&D.
http://challenge.roadef.org.
Sherali, H. D. and Adams, W. P. (1990). A hierarchy of
relaxations between the continuous and convex hull
representations for zero-one programming problems.
SIAM Journal on Discrete Mathematics, 3(3):411–
430.
Todd, M. J. (2001). Semidefinite optimization. Acta Nu-
merica, 10:515–560.
Vandenberghe, L. and Boyd, S. (1994). Semidefinite pro-
gramming. SIAM Review, 38:49–95.
Wolkowicz, H., Saigal, R., and Vandenberghe, L., editors.
Handbook of Semidefinite Programming: Theory, Al-
gorithms, and Applications, volume 27.
SEMIDEFINITE RELAXATIONS FOR THE SCHEDULING NUCLEAR OUTAGES PROBLEM
391