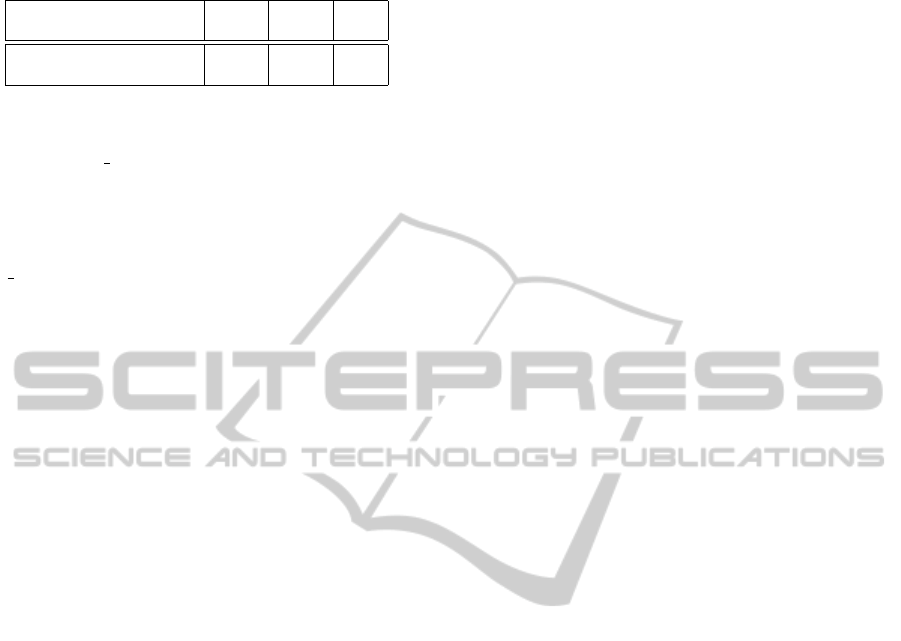
Table 1: Average gain of decision depending on the average
vehicle speed.
`
`
`
`
`
`
`
`
`
`
Gain
Vehicle speed
30 40 50
Flow of 400 veh/h 6,79 5,56 5,67
Flow of 600 veh/h 15,62 11,88 9,25
dition) when the agents knows it will not be able to
cross because of an incoming vehicle, the number of
decision(s), no condition it would have to make with-
out the no perception condition.
Table 1 presents the average gain of perception-
decision cycles with the no perception condition : it
is the difference between the average of condition and
no condition for all the agents. We see an impor-
tant gain, even for high speeds. It is not surprising
that this gain also increases with a higher flow, since
the agent has less opportunities to cross. The road-
crossing model diminishes the number of treatments,
both in terms of road-crossing decisions and of num-
ber of vehicles perceived, while keeping acceptable
behavior results.
6 CONCLUSIONS
We have presented a pedestrian agent model encom-
passing both action (locomotion) and decision (street
crossing). Both tasks are highly dependent on the per-
ception of the situation, but do not rely on the same
pieces of information. Hence, we have shown that the
refinement of the perception, based on a goal-oriented
filtering of the percepts, enables the decrease of the
number of objects treated by the agents while keep-
ing consistent results in term of behavior.
The difference between the raw perception and the
filtered perception has been evaluated and depends
on the model: from 70% to 90% for the locomotion
model. The filtering we have proposed is not an im-
plementation artefact to ameliorate the time complex-
ity of our agents, but a part of the human cognitive
process. We note that humans can only acknowledge
and handle a limited amount of information. Future
works needs to be done to establish which percepts
must be kept to ameliorate the perception process.
More research is needed to better understand and
reproduce a group behavior (Moussa
¨
ıd et al., 2010),
on particular in the street crossing case. Donikian
notes that groups show bolder behavior by accepting
shorter time gaps and by forcing the vehicle drivers to
slow down (Donikian, 2004). Several problems arise
(group identification and formation). Another inter-
esting possibility to model large areas would be to
switch from a microscopic to a macroscopic model
at run-time.
REFERENCES
Auberlet, J.-M., Bodard, V., Bourgois, L., Br
´
emond, R.,
D
´
esir
´
e, L., Maestracci, M., Grani
´
e, M.-A., Rabier, R.,
and Tom, A. (2011). Simulation de travers
´
ee de car-
refour par des pi
´
etons. Technical report, Fondation
S
´
ecurit
´
e Routi
`
ere, projet SiCAP. (to be published).
Blue, V. J. and Adler, J. (2001). Cellular automata mi-
crosimulation for modeling bi-directional pedestrian
walkways. Transportation Research Journal Part B.
Bourgois, L., Oulhaci, A., and Auberlet, J.-M. (2010). Sim-
ulation de d
´
eplacement de pi
´
etons : Vers un mod
`
ele
de perception et de pr
´
ediction d’action chez autrui. In
Journ
´
ees Francophones des Syst
`
emes Multi-Agent.
Donikian, S. (2004). Mod
´
elisation, contr
ˆ
ole et animation
d’agents virtuels autonomes
´
evoluant dans des en-
vironnements inform
´
es et structur
´
es. Habilitation
`
a
Diriger des Recherches, Institut de Recherche en In-
formatique et Syst
`
emes Al
´
eatoires.
Guy, S. J., Lin, M. C., and Manocha, D. (2010). Modeling
collision avoidance behavior for virutal humans. In
9th International Conference on Autonomous Agents
and Multi-agent Systems.
Helbing, D. and Molnar, P. (1995). Social force model for
pedestrian dynamics. Physical Review E.
Hoogendoorn, S. and Bovy, P. (2000). Gas-kinetic model-
ing and simulation of pedestrian flows. Transportation
Research Record.
Johansson, A., Helbing, D., and Shukla, P. (2007). Specifi-
cation of the social force pedestrian model by evolu-
tionary adjustment to video tracking data. Advances
in Complex Systems.
Ketenci, U., Br
´
emond, R., Auberlet, J.-M., and Griselin,
E. (2010). Bounded active perception. In Proceedings
EUMAS, European Workshop on Multi-agent Systems.
Lobjois, R. and Cavallo, V. (2009). The effects of aging
on street-crossing behavior: From estimation to actual
crossing. Accident Analysis and Prevention.
Moussa
¨
ıd, M., N.Perozo, Garnier, S., Helbing, D., and
Theraulaz, G. (2010). The walking behaviour of
pedestrian social groups and its impact on crowd dy-
namics. In PLoS ONE.
Reynolds, C. (2000). Interaction with groups of au-
tonomous characters. In Game Developers Confer-
ence.
Staplin, L. (1995). Simulator and field measures of driver
age difference in left-turn gap judgments. Transport
Research Record.
Weidmann, U. (1993). Transporttechnik der fussg
¨
anger,
transporttechnische eigenschaften des fuss-
gngerverkehrs (literturauswertung). Technical
report, Schriftenreihe des IVT Nr. 90, Zweite,
erg
¨
anzte Auflage, Z
¨
urich.
Weyns, D., Steegmans, E., and Holvoet, T. (2004). Towards
active perception in situated multiagent systems. Spe-
cial Issue of Journal on Applied Artificial Intelligence.
ICAART 2012 - International Conference on Agents and Artificial Intelligence
202