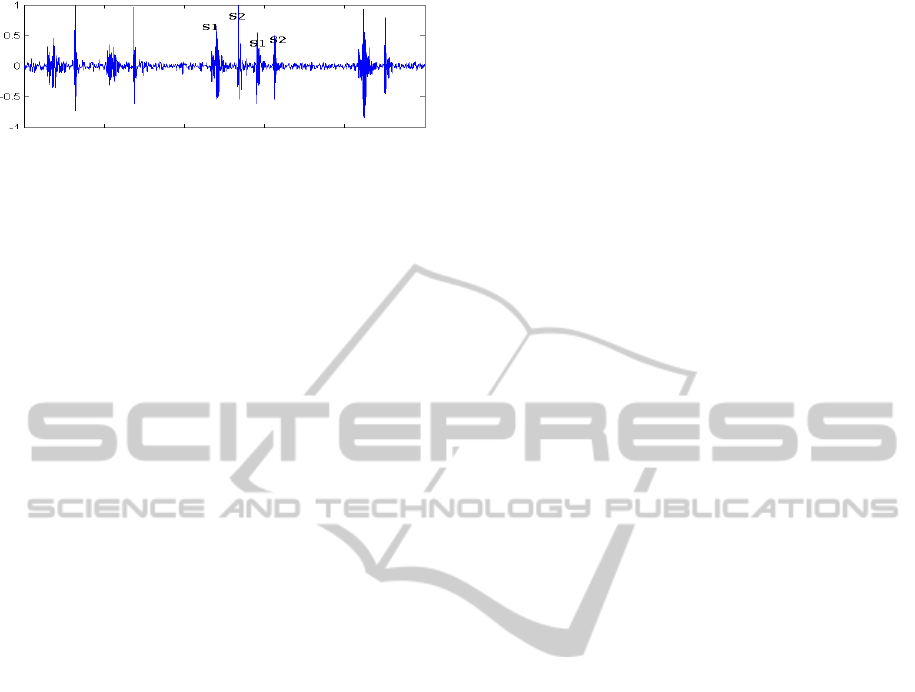
Figure 1: Example of an arrhythmic subject.
This paper is organized as following: sections 2.1
and 2.2 describe the dataset used in this study and
the acquisition and pre-processing of PCG signals,
respectively. In section 2.3 the localization and the
boundaries detection algorithms are presented
briefly, section 2.4 describes the feature extraction
methods. The results and discussion are presented in
section 3, while section 4. consists in conclusions.
2 MATERIAL AND METHODS
2.1 Data Set
Different cardiologists equipped with a prototype
electronic stethoscope with a Bluetooth standard
communication module, have contributed to a
campaign of heart auscultation in the Hospital of
Strasbourg. In parallel, 2 prototypes have been
dedicated to the MARS500 project, promoted by
ESA, in order to collect signals all two months from
6 volunteers (astronauts) in a confinement
experience lasting 520 days and transmitted to IBMP
station in Moscow as a real telemedicine
investigation. The use of prototype electronic
stethoscopes by different cardiologists makes the
database rich in terms of qualitative diversity for the
collected sounds and create the condition for a real
life database.((.n more realistic.))
The dataset contains 80 subjects, including 40
cardiac pathologic auscultation sounds which
contain different systolic murmurs and tachycardia
and or arrhythmia cases. Each recorded auscultation
corresponds to one patient. The length of each
recording lasts between 8 and 12 seconds which
represents generally the time of tolerated apnoea.
2.2 Acquisition and Pre-processing of
PCG Signals
The sounds are recorded with 16 bits accuracy and
8000Hz sampling frequency in a wave format, using
the software “Stetho” developed under Alcatel-
Lucent license. The original signal is decimated by
factor 4 to 2000 Hz sampling frequency and then the
signal is filtered by a high-pass filter with cut-off
frequency of 30 Hz to eliminate the noise collected
by the prototype stethoscope. The filtered signal is
pre-filtered reverse direction so that there is no time
delay in the resulting signal. Then, the
Normalization is applied by setting the variance of
the signal to a value of 1.
2.3 Localization and Boundaries
Detection of Heart Sounds
In this study, the localization of heart sounds is
established by using the SRBF method based on S-
transform and radial basis functions (RBF) neural
network (Moukadem et al., 2011). The boundaries of
the heart sounds are determined by the first local
minima before and after the located sound.
The results were visually inspected by a
cardiologist and erroneously extracted heart sounds
were excluded from the study.
2.4 Classification of S1 and S2
The initial component of S1 is related mitral and
tricuspid valve closure, due to contraction of
ventricles, thus identifying the onset of ventricular
systole and the end of mechanical diastole. The S2 is
produced by the aortic and pulmonic valves closing
when left ventricular pressure decreases under
diastolic aortic pressure. The vibrations of S2 occur
at the end of ventricular contraction and identify the
onset of ventricular diastole and the end of
mechanical systole (Felner, 1990). These
physiological differences lead to different time and
frequency content behaviour between S1 and S2.
Two feature extraction methods for
distinguishing between S1 and S2 are examined.
The feature extraction process extracts a feature
vector per extracted sound H
i
and each of these
vectors is averaged across available extracted sounds
from each subject. So from each subject in the
database, we obtain one S1 feature vector and one
S2 feature vector to use in the training and
classification process.
2.4.1 Feature Extraction using the
s-Transform
The SVD technique is a powerful tool to represent
the time-frequency matrix in a compact manner.
Hassanpour et al. proposed a feature extraction
method based on SVD technique to classify EEG
seizures (Hassanpour et al., 2004). The advantage of
this approach, that it incorporates information from
the eigenvectors, which contains relevant
STUDY OF TWO FEATURE EXTRACTION METHODS TO DISTINGUISH BETWEEN THE FIRST AND THE
SECOND HEART SOUNDS
347