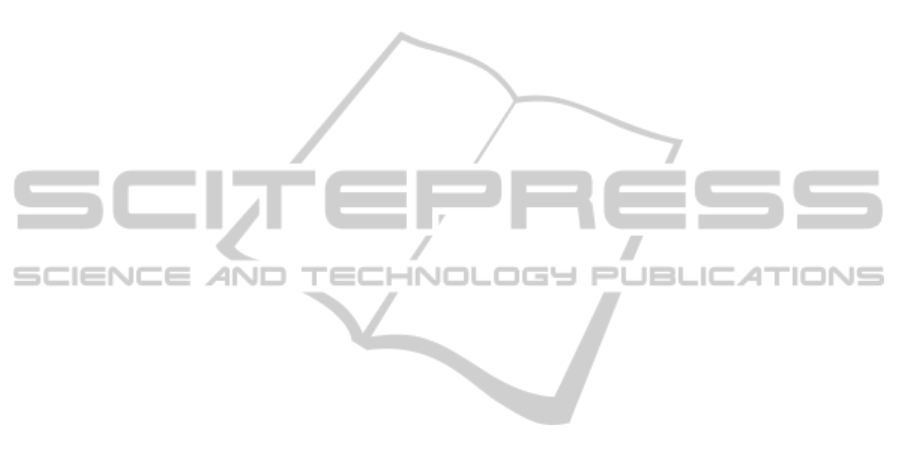
in the context of the Topic Detection and Tracking
(TDT) research program (Allan, 2002). Within the
TDT research, Allan determined five tasks (i.e., Story
Segmentation, First Story Detection, Cluster Detec-
tion, Tracking, and Story Link Detection) for detect-
ing the several topics outlined in a text-based news-
cast. Further offline approaches compute the co-
herence between documents via similarity measures
(e.g., (Makkonen et al., 2004; Zhang and Wang,
2010)). Others rank Wikipedia articles according
to their relevance to a given text fragment, for ex-
ample via text classification algorithms (Gabrilovich
and Markovitch, 2007) or by simply exploiting the
Wikipedia article titles and categories (Sch
¨
onhofen,
2006). One recent approach uses the Wikipedia cate-
gory network as a conceptual taxonomy and derives a
directed acyclic graph for each document by mapping
terms to a concept in the category network (Chahine
et al., 2011).
Approaches for the online identification of topics
in natural language dialogs are rare. One work re-
alizing a “Dynamic Topic Tracking” of natural lan-
guage conversations between a human and a robot
roughly adapted the five tasks from the TDT project
(see above) to make the robot more situation aware in
human-robot interaction (Maas et al., 2006). Thereby
the amount of topics and the according topic names
are created dynamically by gathering the topic names
from content words most occurring in the dialog utter-
ances. On the contrary, existing taxonomies can serve
as a source for topic labels, for example derived from
the online encyclopedia Wikipedia (Breuing et al.,
2011; Waltinger et al., 2011). Furthermore, con-
versation clusters visually highlight topics discussed
in conversations using Explicit Semantic Analysis
based on Wikipedia articles (Bergstrom and Kara-
halios, 2009).
7 CONCLUSIONS AND FUTURE
WORK
We presented an approach for the automatic emula-
tion of humanlike topic awareness in ongoing small
talk dialogs to extend the conversational abilities of a
virtual agent in human-agent interactions. More pre-
cisely, we proposed solutions for both tasks the auto-
matic identification of dialog topics and the integra-
tion of the resulting topic information into the agent’s
existing system architecture. The several associated
processes fulfill the requirements given by a face-to-
face encounter between a human and a conversational
agent and enable both a coherent and socially ade-
quate dialog between the human and the artificial in-
terlocutors. Thereby, we exploit Wikipedia knowl-
edge and hence the benefits originated from collab-
orative work (namely the existence of information
whose maintenance and expansion is carried out by
numerous volunteers and the reflection of the partici-
pants’ common perception of conceptual structures).
In future, we will extend our approach by detect-
ing and linking topical affiliations to previous dialog
topics to handle short side trips to past topics. More-
over, we will resolve ambiguities by taking into ac-
count the current dialog topic to influence the concept
detection process.
ACKNOWLEDGEMENTS
This work is kindly supported by the Deutsche
Forschungsgemeinschaft (DFG) in the context of the
KnowCIT research project in the Center of Excel-
lence Cognitive Interaction Technology (CITEC) at
Bielefeld University. We thank Birgit Endrass and
Elisabeth Andr
´
e from the University of Augsburg for
providing parts of their CUBE-G corpus.
REFERENCES
Allan, J. (2002). Topic Detection and Tracking: Event-
based Information Organization. Kluwer Academic
Publishers.
Bergstrom, T. and Karahalios, K. (2009). Conversa-
tion Clusters: Grouping Conversation Topics through
Human-Computer Dialog. In Proceedings of the Inter-
national Conference on Human Factors in Computing
Systems (CHI09).
Breuing, A., Waltinger, U., and Wachsmuth, I. (2011).
Harvesting Wikipedia Knowledge to Identify Topics
in Ongoing Natural Language Dialogs. In Proceed-
ings of the 2011 IEEE/WIC/ACM International Joint
Conference on Web Intelligence and Intelligent Agent
Technology, pages 445–450.
Bublitz, W. (1989). Topical Coherence in Spoken Dis-
course. Studia Anglica Posnaniensia, 22:31–51.
Cassell, J., Bickmore, T., Campbell, L., Vilhj
´
almsson, H.,
and Yan, H. (2000). Human Conversation as a Sys-
tem Framework: Designing Embodied Conversational
Agents. In Cassell, J., Sullivan, J., and Churchill, E.,
editors, Embodied Conversational Agents, pages 29–
63. MIT Press.
Chahine, C. A., Chaignaud, N., Kotowicz, J.-P., and
P
´
ecuchet, J.-P. (2011). Conceptual Indexing of Doc-
uments Using Wikipedia. In Proceedings of the 2011
IEEE/WIC/ACM International Joint Conference on
Web Intelligence and Intelligent Agent Technology,
pages 195–202.
Clark, H. H. (1996). Using Language. Cambridge Univ.
Press.
ICAART 2012 - International Conference on Agents and Artificial Intelligence
70