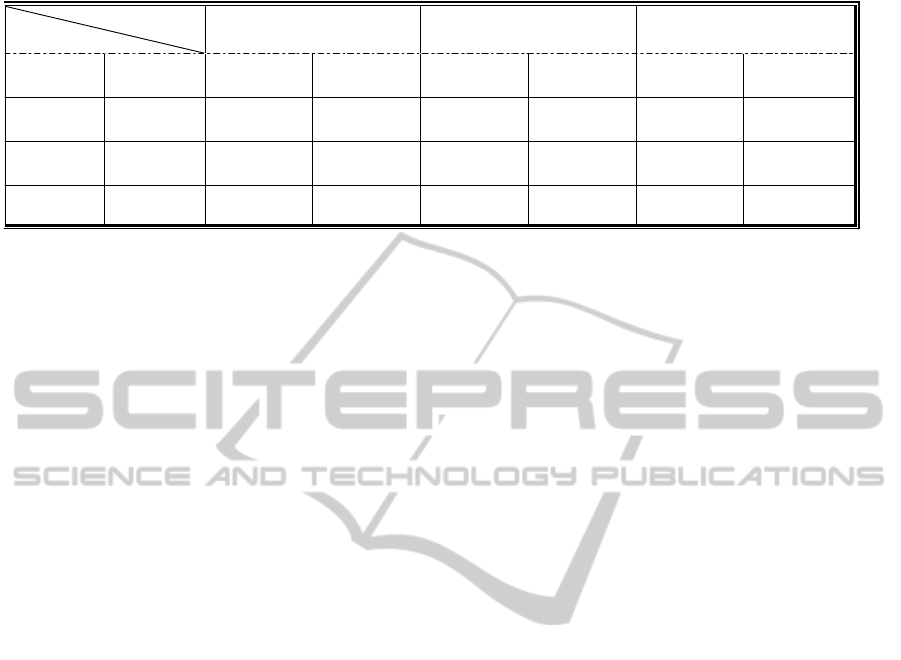
Table 1: Evaluation of the obtained concepts using CR, CR_IDF and TF_IDF mesures.
Measure
Extracted concepts
CR CR_IDF
TF_IDF
Type Number
Affirmed
Concepts
Precision
(%)
Affirmed
Concepts
Precision
(%)
Affirmed
Concepts
Precision
(%)
One-word
Concept
105 44 41.90% 71 67.62%
61 58.09%
Two-word
Concept
29 16 55.17% 18 62.07%
9 31.03%
Total 134 60 44.78% 89 66.42%
70 52.24 %
specialized dictionary in astronomy and space
(Cotardière and Penot, 1999). We have also fixed
w
Bold
=3, w
Italic
=2, w
url
=2 and w
rest
=1.
In table 1, we present the results obtained using
CR (Corpus Relevance) and CR_IDF measures. The
CR_IDF measure gives more interesting results than
CR ones. This can be explained by the fact that the
use of IDF factor removes CT which are the less
specific to the considered field. To evaluate our
approach, we compare our results (CR_IDF) with
the most used measure in the literature TF_IDF. We
note that our CR_IDF measure which considers the
documents structure gives better results than
TF_IDF. These results show the impact of
considering the documents structure in the extraction
of concepts by giving the most relevant ones.
6 CONCLUSIONS AND FUTURE
WORK
In this paper, we have defined a strategy which
extracts automatically the concepts of the ontology
from a corpus of Web documents. This strategy is
based on the study of the document structure by
extracting the typographical titles, links and
markings. Indeed, the structure of the documents
provides interesting information on the significance
contained in the texts. We can extend the work
performed by analyzing the hierarchy of the titles in
each document in order to extract the hierarchical
links to lead to ontology. The text can be then
apprehended not like a linear succession of blocks of
various natures, but like a structure of elements of
high level which include other elements.
REFERENCES
Berners-Lee, T. Hendler, J., Lassila, O, 2001. The
Semantic Web. Scientific American, pp. 28–37
Bourrigault, D., Fabre, C., Frérot, C., Jacques, M. P.,
Ozdowska, S., 2005. Syntex, analyseur syntaxique de
corpus. In: Actes des 12èmes journées sur le
Traitement Automatique des Langues Naturelles, pp.
17-20, Dourdan, France
Cotardière, P., Penot, J. P., 1999. Dictionnaire de
l'Astronomie et de l'Espace. eds. Larousse-Bordas
Hazman, M., El-Beltagy, S.R., Rafaa, A., 2009. Ontology
Learning from Domain Specific Web Documents.
International Journal of Metadata, Semantics and
Ontologies, Vol 4, Number 1-2, pp. 24-33
Joachims, T., 1997. A probabilistic analysis of the
Rocchio algorithm with TFIDF for text categorization.
In: Proc. 14th International Conference on Machine
Learning, pp. 143- 151, Morgan Kaufmann
Karoui, L., Aufaure, M. and Bennacer, N., 2004. Ontology
Discovery from Web Pages: Application to Tourism.
In: Knowledge Discovery and Ontologies Workshop at
ECML/PKDD
Lopez, C., Prince, V., Roche, M., 2010. Automatic Titling
of Electronic Documents with Noun Phrase
Extraction. In: Proceedings of Soft Computing and
Pattern Recognition, SOCPAR'10, pp. 168-171, Paris,
France
Morin, E., 1999. Using Lexico-Syntactic Patterns to
Extract Semantic Relations between terms from
Technical Corpus. In: Proceedings of the 5
th
International Congress on Terminology and
Knowledge Engineering (TKE'99), pp 268-278
Schmid, H., 1994. Probabilistic Part-of-Speech Tagging
Using Decision Trees. In: Proceedings of the
International Conference on New Methods in
Language Processing
Séguéla, P., 1999. Adaptation semi-automatique d'une
base de marqueurs de relations sémantiques sur des
corpus spécialisés. Revue Terminologies, Number 19,
pp. 52-61
Velardi, P., Fabriani, P. and Missikoff, M. , 2002. Using
text processing techniques to automatically enrich a
domain ontology, In Proceedings of the ACM
Conference on Formal Ontologies and Information
Systems, pp 270-284
ICAART 2012 - International Conference on Agents and Artificial Intelligence
506