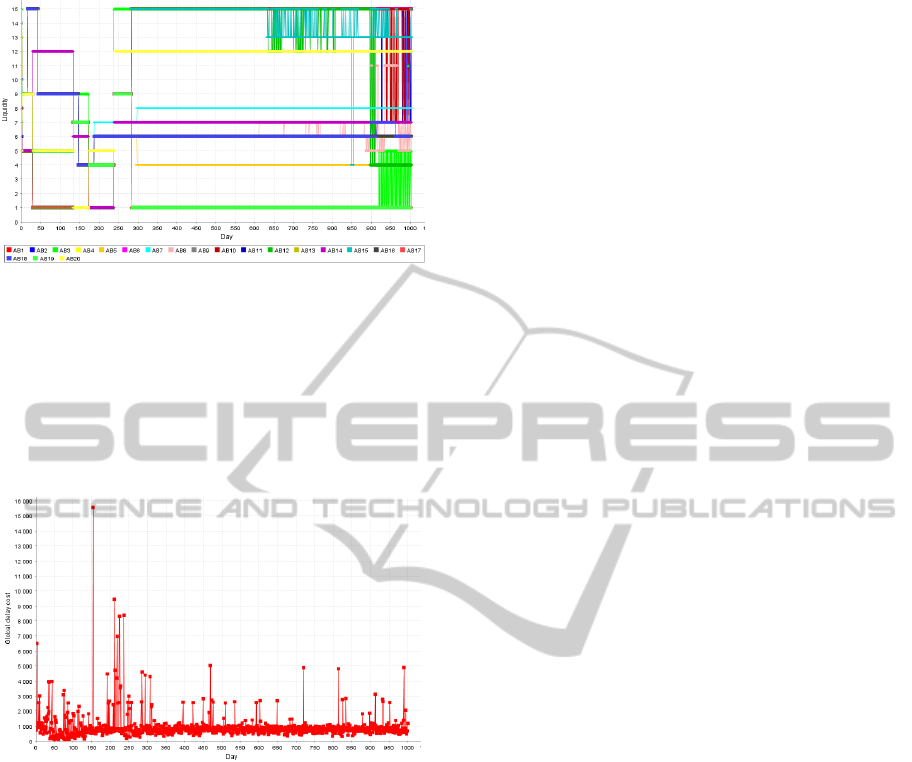
Figure 6: Liquidity of the 20 BA α =1%; β=10%.
Figure 6 shows that liquidity value for each BA
is more stable when α and β are small and that β
coefficient influences the system evolution more
than α. Figure 7 shows total cost of delaying. We
notice that as the number of BA increases, the cost
of delaying decreases. This indicates that more there
is sources of liquidity, less there is delaying.
Figure 7: Cost of delaying of 10 BA α=10%; β=10%.
These results show that the best configuration of the
coefficients is α=10%; β=1%. For these values,
global liquidity is more stable. BA agents propose
closer liquidity values and minimized costs of
delaying. All these experiments show that efficient
RTGS management is possible with smart choices of
costs coefficients. They also help to determine daily
liquidity values that stabilize the system and allow
RTGS decision makers implementing policies
specifying liquidity values that banks must choose.
6 CONCLUSIONS
In this paper, we have presented a multi-agent model
for simulating RTGS systems. This model has been
implemented and tested with different parameters.
Our tool can be used as a decision support system by
adapting it with real RTGS data in the codification
of real liquidity and cost values in CS rules.
Decision support is possible by searching liquidity
values, costs coefficients that cause stabilities or
instabilities. Our simulation model is intended for
central banks, private banks, specialists or any
person interested by RTGS systems. Some
improvements could be added to this work such as:
(1) considering payments of different priorities; (2)
taking other parameters such as bank reputation.
REFERENCES
Angelini, P., 1998. An analysis of competitive
externalities in gross settlement systems, JBF, vol.22.
Arciero, L., Biancott, C., D’Aurizio, L. L., Impenna, C.,
2009. Exploring agent-based methods for the analysis
of payment systems: a crisis model for StarLogo TNG,
Working paper, Banca d’Italia.
BIS: Bank of International Settlements, 1997. Real-Time
Gross Settlement systems, Report of the Committee on
Payment and Settlement Systems of central banks of
Group of 10 countries, www.bis.org.
Bech, M., Garratt, R., 2003. The intraday liquidity
management game, JET, vol. 109(2), pp. 198-219.
Bech, M., Garratt, R., 2006. Illiquidity in the Interbank
Payment System Following, Wide-Scale Disruptions,
Federal Reserve, New-York, Report No. 239.
Beyeler, W. E., Glass, R. J., Bech, M., Soramäki, K.,
2007. Congestion and cascades in payment systems,
Physica A, doi:10.1016/j.physa.2007.05.061.
Devriese, J., Mitchell, J., 2006. Liquidity Risk in
Securities Settlement, Journal of Banking and
Finance, vol. 30, iss. 6, pp. 1807-1834.
Galbiati, M., Soramäki, K., 2007. A competitive multi-
agent model of interbank payment systems, arXiv.org.
Holland, J. H., 1987. Genetic algorithms and classifier
systems: Foundations and future directions. In J.J.
Grefenstette, the 2
nd
ICGAA, pp. 82-89.
Koponen, R., Soramäki, K., 1998. Intraday liquidity needs
in a modern inter-bank payment system – A
simulation approach, Studies in Economics and
Finance E: 14, Bank of Finland, Helsinki.
Leinonen, H., 2005. Liquidity, risks and speed in
payments and settlement systems - A simulation
approach, Bank of Finland Studies.
Mezzetti, C., Dindoš M., 2006. Better-reply dynamics and
global convergence to Nash equilibrium in aggregative
games, Games and Economic Behaviour, vol. 54, pp.
261-292.
Parunak, H. V. D., Savit, R., Riolo, R., 1998. Agent-based
modeling vs. equation-based modeling. In Multi-agent
systems and Agent-Based Systems, MABS'98, LNAI
1534, pp. 10-25. Springer.
Thisse, J.-F., 2004. Théorie des jeux : une introduction,
Notes de cours, Université catholique de Louvain,
Département des sciences économiques.
INTERBANK PAYMENT SYSTEM (RTGS) SIMULATION USING MULTI-AGENT APPROACH
365