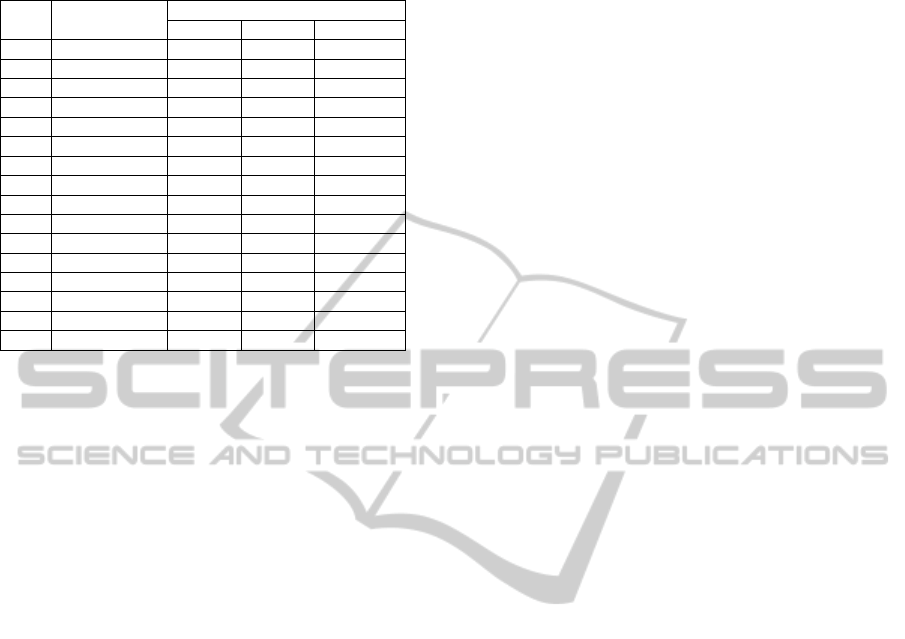
Table 5: The per-category performance comparison for
different models during testing.
Cat. Measure
Method
RBF MLP DT
s
1
Sn 100 98 86.5
Sp 99.67 99.83 95.83
PPV 99.01 99.49 87.37
NPV 100 99.34 95.51
s
2
Sn 100 100 89
Sp 99.67 99 96.5
PPV 99.01 97.09 89.45
NPV 100 100 96.34
s
3
Sn 90 87 79
Sp 98.67 97.5 92.67
PPV 95.74 92.06 78.22
NPV 96.24 95.74 92.98
s
4
Sn 95 94 77.5
Sp 97 96.67 92.33
PPV 91.35 90.38 77.11
NPV 98.31 94.1 92.49
5 CONCLUSIONS
In this paper we described a novel approach for
automatic classification of deformable geometric
shapes based on RBF networks and transform-based
features. The performance of the proposed system is
empirically evaluated and compared with other
classification algorithms. Results showed that the
proposed approach has better performance than the
other considered classification algorithms in terms
of classification accuracy, sensitivity, specificity,
positive predictive value, and negative predictive
value. As a future work we are comparing the
proposed approach with other classifiers and we are
investigating other ways to improve the results
further.
ACKNOWLEDGEMENTS
The authors would like to acknowledge the support
of the Intelligent Systems Research Group and
Deanship of Scientific Research at King Fahd
University of Petroleum and Minerals (KFUPM),
Dhahran, Saudi Arabia.
REFERENCES
Barutcuoglu, Z., DeCoro, C., 2006. Hierarchical shape
classification using Bayesian aggregation. In
Proceedings of the IEEE International Conference on
Shape Modeling and Applications, Matsushima, Japan.
Bishop, C. M., 1995. Neural networks for pattern
recognition, Oxford University Press, New York.
Chang, C. C., Hwang, S. M., Buehrer, D. J., 1991. A shape
recognition scheme based on relative distances of
feature points from the centroid. Pattern Recognition,
24(11): 1053–1063.
Chen, S., Hong X., Harris, C. J., 2005. Orthogonal
forward selection for constructing the radial basis
function network with tunable nodes. In Proceedings
of the IEEE International Conference on Intelligent
Computing.
Chen, L., Feris, R. S., Turk, M., 2008. Efficient partial
shape matching using Smith-Waterman algorithm. In
Workshop on Non-Rigid Shape Analysis and
Deformable Image Alignment (NORDIA'08), in
conjunction with CVPR'08, Anchorage, Alaska.
Chen, L., McAuley, J., Feris, R., Caetano, T., Turk, M.,
2009. Shape classification through structured learning
of matching measures. In Proceeding of the IEEE
Conference on Computer Vision and Pattern
Recognition (CVPR 2009), Miami, Florida.
Costa, L., Cesar Jr., R. M., 2000. Shape analysis and
classification: Theory and practice, CRC Press.
El-Alfy, E.-S. M., 2008. Abductive learning approach for
geometric shape recognition. In Proceedings of the
International Conference on Intelligent Systems and
Exhibition, Bahrain.
Lazzerini, B., Marceelloni, F., 2001. A fuzzy approach to
2-D shape recognition. IEEE Transactions on Fuzzy
Systems, 9(1): 5-16.
George, N., Wang, S., Venable, D. L., 1989. Pattern
recognition using the ring-wedge detector and neural
network software. SPIE, 1134: 96-106.
Gorelick, L., Galun, M., Sharon, E., Basri, R., Brandt, A.,
2006. Shape representation and classification using the
Poisson equation. IEEE Transactions on Pattern
Analysis and Machine Intelligence, 28(12): 1991-
2005.
Haykin, S., 2009. Neural networks and learning machines.
Third Edition, Prentice-Hall.
Ling, H., Jacobs, D. W., 2007. Shape classification using
the inner-distance. IEEE Transactions on Pattern
Analysis and Machine Intelligence, 29(2): 286-299.
McNeil, G., Vijayakumar, S., 2005. 2D shape
classification and retrieval. In Proceedings of the
International Joint Conference on Artificial
Intelligence (IJCAI’05), Edinburgh, Scotland.
Moorehead, L. B., Jones, R. A., 1988. A neural network
for shape recognition. In Proceedings of the IEEE
Region 5 Conference, Piscataway, NJ.
Neruda, R., Kudova, P., 2005. Learning methods for radial
basis functions networks. Future Generation
Computer Systems, 21: 1131-1142.
Powel, M., 1985. Radial-basis functions for multivariable
interpolation: A review. In Proceedings of the IMA
Conference on Algorithms for the Approximation of
Functions and Data, Shrivenham, England.
Pun, C.-M., Lin, C., 2010. Geometric invariant shape
classification using hidden Markov model. In
Proceedings of the IEEE International Conference on
Digital Image Computing: Techniques and
Applications (DICTA 2010).
CLASSIFICATION OF DEFORMABLE GEOMETRIC SHAPES - Using Radial-Basis Function Networks and
Ring-wedge Energy Features
361