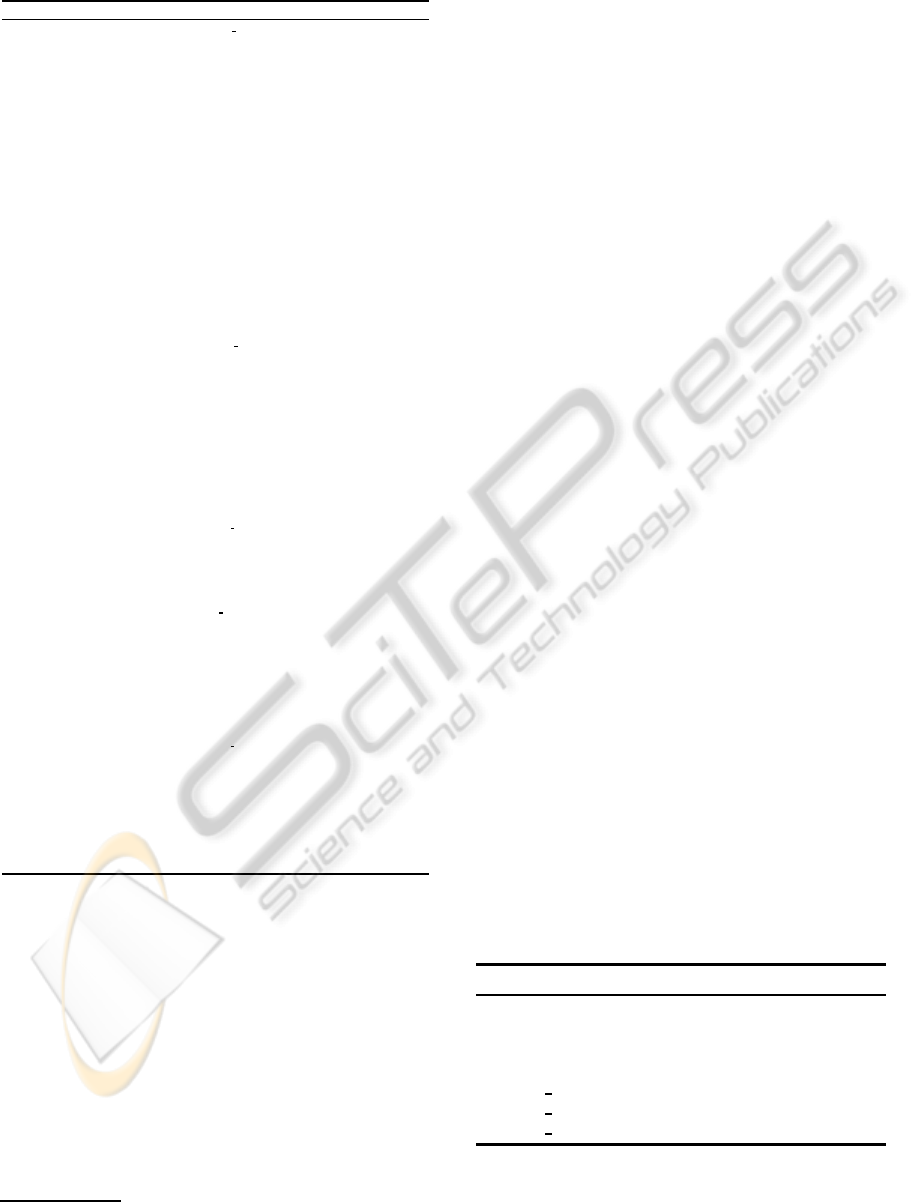
Table 2: Summary of imbalanced data-sets.
Data-sets #Ex. #Atts. Class (-,+) %Class(-; +) IR
Glass1 214 9 (build-win-non float-proc; remainder) (35.51, 64.49) 1.82
Ecoli0vs1 220 7 (im; cp) (35.00, 65.00) 1.86
Wisconsin 683 9 (malignant; benign) (35.00, 65.00) 1.86
Pima 768 8 (tested-positive; tested-negative) (34.84, 66.16) 1.90
Iris0 150 4 (Iris-Setosa; remainder) (33.33, 66.67) 2.00
Glass0 214 9 (build-win-float-proc; remainder) (32.71, 67.29) 2.06
Yeast1 1484 8 (nuc; remainder) (28.91, 71.09) 2.46
Vehicle1 846 18 (Saab; remainder) (28.37, 71.63) 2.52
Vehicle2 846 18 (Bus; remainder) (28.37, 71.63) 2.52
Vehicle3 846 18 (Opel; remainder) (28.37, 71.63) 2.52
Haberman 306 3 (Die; Survive) (27.42, 73.58) 2.68
Glass0123vs456 214 9 (non-window glass; remainder) (23.83, 76.17) 3.19
Vehicle0 846 18 (Van; remainder) (23.64, 76.36) 3.23
Ecoli1 336 7 (im; remainder) (22.92, 77.08) 3.36
New-thyroid2 215 5 (hypo; remainder) (16.89, 83.11) 4.92
New-thyroid1 215 5 (hyper; remainder) (16.28, 83.72) 5.14
Ecoli2 336 7 (pp; remainder) (15.48, 84.52) 5.46
Segment0 2308 19 (brickface; remainder) (14.26, 85.74) 6.01
Glass6 214 9 (headlamps; remainder) (13.55, 86.45) 6.38
Yeast3 1484 8 (me3; remainder) (10.98, 89.02) 8.11
Ecoli3 336 7 (imU; remainder) (10.88, 89.12) 8.19
Page-blocks0 5472 10 (remainder; text) (10.23, 89.77) 8.77
Ecoli034vs5 200 7 (p,imL,imU; om) (10.00, 90.00) 9.00
Yeast2vs4 514 8 (cyt; me2) (9.92, 90.08) 9.08
Ecoli067vs35 222 7 (cp,omL,pp; imL,om) (9.91, 90.09) 9.09
Ecoli0234vs5 202 7 (cp,imS,imL,imU; om) (9.90, 90.10) 9.10
Glass015vs2 172 9 (build-win-non
float-proc,tableware, (9.88, 90.12) 9.12
build-win-float-proc; ve-win-float-proc)
Yeast0359vs78 506 8 (mit,me1,me3,erl; vac,pox) (9.88, 90.12) 9.12
Yeast02579vs368 1004 8 (mit,cyt,me3,vac,erl; me1,exc,pox) (9.86, 90.14) 9.14
Yeast0256vs3789 1004 8 (mit,cyt,me3,exc; me1,vac,pox,erl) (9.86, 90.14) 9.14
Ecoli046vs5 203 6 (cp,imU,omL; om) (9.85, 90.15) 9.15
Ecoli01vs235 244 7 (cp,im; imS,imL,om) (9.83, 90.17) 9.17
Ecoli0267vs35 224 7 (cp,imS,omL,pp; imL,om) (9.82, 90.18) 9.18
Glass04vs5 92 9 (build-win-float-proc,containers; tableware) (9.78, 90.22) 9.22
Ecoli0346vs5 205 7 (cp,imL,imU,omL; om) (9.76, 90.24) 9.25
Ecoli0347vs56 257 7 (cp,imL,imU,pp; om,omL) (9.73, 90.27) 9.28
Yeast05679vs4 528 8 (me2; mit,me3,exc,vac,erl) (9.66, 90.34) 9.35
Ecoli067vs5 220 6 (cp,omL,pp; om) (9.09, 90.91) 10.00
Vowel0 988 13 (hid; remainder) (9.01, 90.99) 10.10
Glass016vs2 192 9 (ve-win-float-proc; build-win-float-proc, (8.89, 91.11) 10.29
build-win-non
float-proc,headlamps)
Glass2 214 9 (Ve-win-float-proc; remainder) (8.78, 91.22) 10.39
Ecoli0147vs2356 336 7 (cp,im,imU,pp; imS,imL,om,omL) (8.63, 91.37) 10.59
Led7digit02456789vs1 443 7 (0,2,4,5,6,7,8,9; 1) (8.35, 91.65) 10.97
Glass06vs5 108 9 (build-win-float-proc,headlamps; tableware) (8.33, 91.67) 11.00
Ecoli01vs5 240 6 (cp,im; om) (8.33, 91.67) 11.00
Glass0146vs2 205 9 (build-win-float-proc,containers,headlamps, (8.29, 91.71) 11.06
build-win-non
float-proc;ve-win-float-proc)
Ecoli0147vs56 332 6 (cp,im,imU,pp; om,omL) (7.53, 92.47) 12.28
Cleveland0vs4 177 13 (0; 4) (7.34, 92.66) 12.62
Ecoli0146vs5 280 6 (cp,im,imU,omL; om) (7.14, 92.86) 13.00
Ecoli4 336 7 (om; remainder) (6.74, 93.26) 13.84
Yeast1vs7 459 8 (nuc; vac) (6.72, 93.28) 13.87
Shuttle0vs4 1829 9 (Rad Flow; Bypass) (6.72, 93.28) 13.87
Glass4 214 9 (containers; remainder) (6.07, 93.93) 15.47
Page-blocks13vs2 472 10 (graphic; horiz.line,picture) (5.93, 94.07) 15.85
Abalone9vs18 731 8 (18; 9) (5.65, 94.25) 16.68
Glass016vs5 184 9 (tableware; build-win-float-proc, (4.89, 95.11) 19.44
build-win-non
float-proc,headlamps)
Shuttle2vs4 129 9 (Fpv Open; Bypass) (4.65, 95.35) 20.5
Yeast1458vs7 693 8 (vac; nuc,me2,me3,pox) (4.33, 95.67) 22.10
Glass5 214 9 (tableware; remainder) (4.20, 95.80) 22.81
Yeast2vs8 482 8 (pox; cyt) (4.15, 95.85) 23.10
Yeast4 1484 8 (me2; remainder) (3.43, 96.57) 28.41
Yeast1289vs7 947 8 (vac; nuc,cyt,pox,erl) (3.17, 96.83) 30.56
Yeast5 1484 8 (me1; remainder) (2.96, 97.04) 32.78
Ecoli0137vs26 281 7 (pp,imL; cp,im,imU,imS) (2.49, 97.51) 39.15
Yeast6 1484 8 (exc; remainder) (2.49, 97.51) 39.15
Abalone19 4174 8 (19; remainder) (0.77, 99.23) 128.87
4 of them (80%) as training and test. For each data-set
we consider the average results of the five partitions.
The data-sets used in this study use the partitions pro-
vided by the repository in the imbalanced classifica-
tion data-set section
3
.
Furthermore, we have to identify the misclassifi-
cation costs associated to the positive and negative
class for the cost-sensitive learning versions. If we
misclassify a positive sample as a negative one the
associated misclassification cost is the IR of the data-
set (C(+, −) = IR) whereas if we misclassify a nega-
tive sample as a positive one the associated cost is 1
3
http://www.keel.es/imbalanced.php
(C(−, +) = 1). The cost of classifying correctly is 0
(C(+, +) = C(−, −) = 0) because guessing the cor-
rect class should not penalize the built model.
Finally, statistical analysis needs to be carried out
in order to find significant differences among the re-
sults obtained by the studied methods (Demˇsar, 2006;
Garc´ıa et al., 2009; Garc´ıa et al., 2010). Since
the study is split in parts comparing a group of al-
gorithms, we use non-parametric statistical tests for
multiple comparisons. Specifically, we use the Iman-
Davenport test (Sheskin, 2006) to detect statistical
differences among a group of results and the Shaf-
fer post-hoc test (Shaffer, 1986) in order to find out
which algorithms are distinctive among an n×n com-
parison.
Furthermore, we consider the average ranking of
the algorithms in order to show graphically how good
a method is with respect to its partners. This rank-
ing is obtained by assigning a position to each algo-
rithm depending on its performance for each data-set.
The algorithm which achieves the best accuracy in a
specific data-set will have the first ranking (value 1);
then, the algorithm with the second best accuracy is
assigned rank 2, and so forth. This task is carried out
for all data-sets and finally an average ranking is com-
puted as the mean value of all rankings.
4.2 Contrasting Preprocessing and
Cost-sensitive Learning in
Imbalanced Data-sets
Table 3 shows the average results in training and test
together with the corresponding standard deviation
for the seven versions of the C4.5 algorithm used in
the study: the base classifier, the base classifier used
overthe preprocessed data-sets, the cost-sensitive ver-
sion of the algorithm and the hybrid versions of it. We
stress in boldface the best results achievedfor the pre-
diction ability of the different techniques.
Table 3: Average table of results using the AUC measure
for the C4.5 variety of algorithms.
Algorithm AUC
tr
AUC
tst
C4.5 0.8774 ± 0.0392 0.7902 ± 0.0804
C4.5 SMOTE 0.9606 ± 0.0142 0.8324 ± 0.0728
C4.5 SENN 0.9471 ± 0.0154 0.8390 ± 0.0772
C4.5CS 0.9679 ± 0.0103 0.8294 ± 0.0758
C4.5 Wr
SMOTE 0.9679 ± 0.0103 0.8296 ± 0.0763
C4.5 Wr
US 0.9635 ± 0.0139 0.8245 ± 0.0760
C4.5 Wr
SENN 0.9083 ± 0.0377 0.8145 ± 0.0712
From this table of results it can be observed that
the highest average value corresponds to preprocess-
ICPRAM 2012 - International Conference on Pattern Recognition Applications and Methods
104