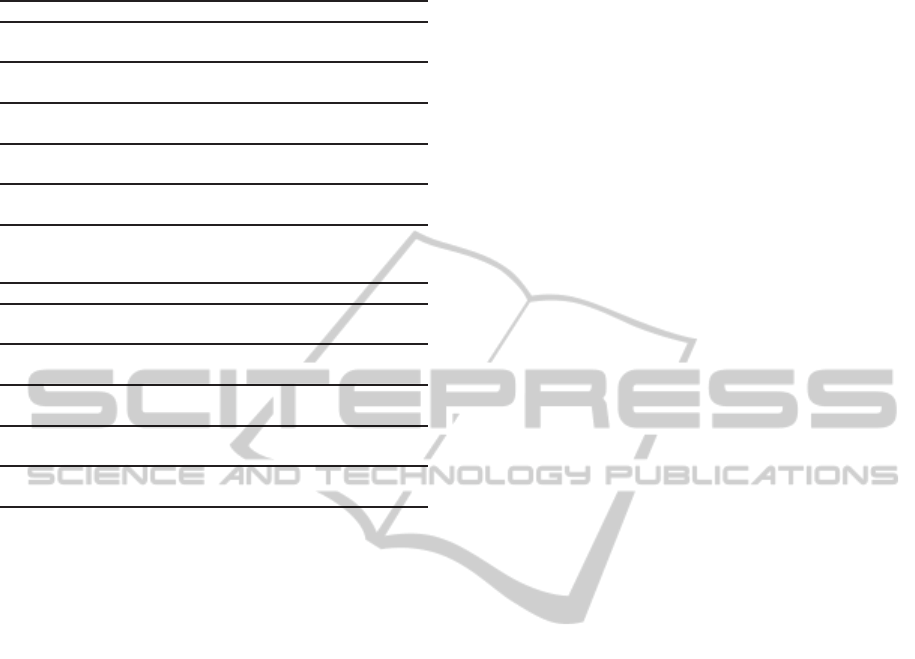
Table 5: Results for the Cancer Dataset.
NNeuro Average± Std Dev Min Max H h
1
h
2
2
95, 6%± 1, 5% 92,1% 98,6%
0 0 0
96%± 1, 5% 92,9% 98,6%
4
95, 3%± 1, 7% 92,1% 98,6%
0 0 0
95, 9%± 1, 4% 92,9% 98,6%
6
95, 1%± 1, 7% 90,7% 97,9%
0 0 1
96%± 1, 6% 92,1% 98,6%
8
96%± 1, 2% 94,3% 98,6%
0 0 0
96, 3%± 1, 3% 93,6% 98,6%
10
95, 8%± 1, 6% 92,1% 100%
1 0 0
96, 2%± 1, 4% 93,6% 99,3%
Table 6: Results for Blood Transfusion Dataset.
NNeuro Average± Std Dev Min Max H h
1
h
2
2
75, 9%± 3, 2% 68,5% 83,9%
0 0 0
75, 9%± 3, 2% 68,5% 83,9%
4
77, 7%± 3, 3% 70,5% 84,6%
0 0 0
77, 2%± 3, 2% 71,1% 85,2%
6
78, 8%± 3, 9% 67,8% 89,3%
0 1 0
77, 5%± 3, 4% 67,8% 85,2%
8
77, 5%± 2, 4% 71,1% 81,9%
0 1 0
75, 9%± 3% 67,8% 81,9%
10
77, 6%± 3, 5% 71,8% 85,2%
0 1 0
76%± 3, 5% 68,5% 84,6%
6 CONSIDERATIONS AND
FUTURE WORK
Considering the results obtained and previously dis-
cussed, we can say that:
• the genetic operators presented did not produced
good populations, given that the GA approach did
not overcome the traditional approach, except in
one case that can be seen in Table 5;
• the GA approach performance did not reach the
expectations when compared with the traditional
approach, because even in the situation was better,
this superiority was not unquestionable;
• there were two cases where none of the two ap-
proaches achieved a significant result: in the data
set “Contraceptive Method Choice”, the best per-
formances of both algorithms was below 70% on
all executions and below 60% on average. In the
case of the “Blood Transfusion”, despite the mean
CCP was higher 70%, a possible application in
reality would not be feasible because the model
would not be reliable;
• the training via GA produced results lower than
the pseudo-inversion in three of four sets tested,
considering the significance defined in the tests.
Taking an overview of the results, considering a
5% level of significance, we can say that the GA ap-
proach even being able to produce results as good
as the supposedly exact approach had a significantly
lower performance in the databases we’ve tested.
However, it is necessary to consider that the exact ap-
proach suffers with complexity and numerical round-
ing errors with the growth of the number of neurons in
the hidden layer. Thus, taking into account the adapt-
ability of GA, if new more robust genetic operators
are developed will overcome this limitation.
6.1 Future Works
Noting the apparent failure of the considerations
made in this study, below we’ve listed some possi-
ble suggestions for future works that may continue or
refute this line of thinking:
• test other GA operators;
• compare other search algorithms (and GA) with
the pseudo-inversion approach;
• compare other search algorithms with supervised
learning approach;
• use statistical techniques such as Principal Com-
ponent Analysis and Fator Analysis in the treat-
ment of data in order to see if they can help im-
prove performance in sets with similar character-
istics o the "Contraceptive Method Choice ", in
which both approaches had less than 70% perfor-
mance.
REFERENCES
Changbing, L. and Wei, H. (2010). Application of genetic
algorithm-rbf neural network in water environment
risk prediction. 2nd International Conference on
Computer Engineering and Technology 2010, pages
239–242.
Frank, A. and Asuncion, A. (2010). Uci machine learning
repository.
Haykin, S. (2001). Redes neurais - princípios e práticas.
Bookman.
Holland, J. (1975). Adaption in Natural and Artificial Sys-
tems. MIT Press.
Kurban, T. and Be¸sdok, E. (2009). A comparsion of rbf
neural netowrk training algorithms for inertial sen-
sor based terrian classification. Sensors, pages 6312–
6329.
Li, N. and Ling, H. (2011). Study of an algorithm of ga-
rbf neural network generalized predictive control for
generating unit. International Conference on Elec-
tric Information and Control Engineering 2011, pages
1723–1726.
Man, K., Tang, K., and Kwong, S. (1996). Genetic algo-
rithms: concepts and applications. IEEE Transactions
on Industrial Electronics, 43(5):519–534.
ICAART 2012 - International Conference on Agents and Artificial Intelligence
378