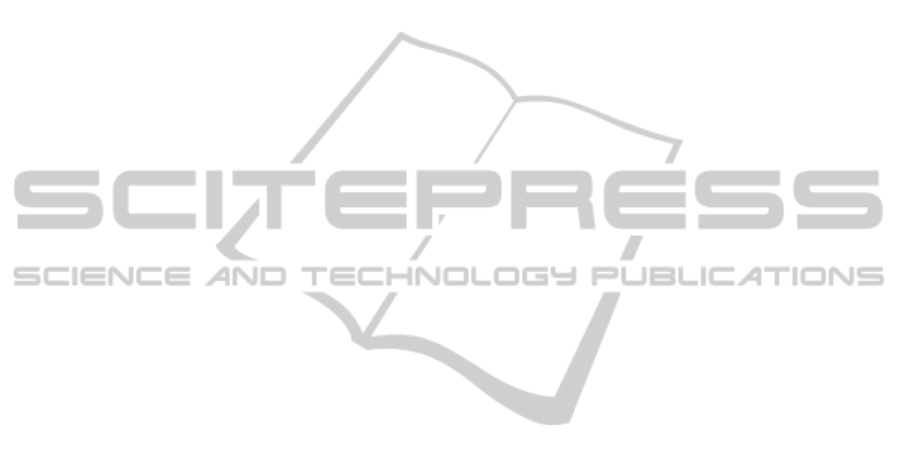
ria, originated from studying competitive encounters.
The insights from what kinds of strategies tend to
do well in Iterated Traveler’s Dilemma do not point
out a paradox, like K. Basu and some other early re-
searchers of TD claimed. Rather, in our opinion, they
expose a fundamental deficiency in applying notions
of rationality that are appropriate in strictly compet-
itive contexts to strategic encounters where both in-
tuition and mathematics suggest that being coopera-
tive is the best way to ensure high individual payoff
in the long run. We point out that some other, newer
notions of game solutions, such as that of regret equi-
libria (Halpern and Pass, 2009), may turn out to pro-
vide a satisfactory notion of individual rationality for
cooperation-rewarding games such as TD; further dis-
cussion of these novel concepts, however, is beyond
our current scope.
We briefly outline some other lessons learned
from detailed analysis of individual and team per-
formances in our round-robin Iterated TD tourna-
ment. These lessons include that (i) common-sense
unselfish greedy behavior (“bid high”) generally tends
to be rewarded in ITD, (ii) not all adaptable/learning
strategies are necessarily successful, even against
simple opponents, (iii) more complex models of an
opponent’s behavior may but need not result in better
performance, (iv) exact choices of critical parameters
may have a great impact on performance (such as with
various bucket-based strategies) or hardly any impact
at all (e.g., the learning rate in Q-learners), and (v)
collaboration via mutual reinforcement between con-
siderably different adaptable strategies appears to of-
ten be much better rewarded than self-reinforcement
between strategies that are very much alike.
Our analysis also raises several interesting ques-
tions, among which we are particularly keen to further
investigate (i) to what extent other variations of cog-
nitively simple models of learning can be expected to
help performance, (ii) to what extent complex mod-
els of the other agent really help an agent increase
its payoff in the iterated play, and (iii) assuming that
this phenomenon occurs more broadly than what we
have investigated so far, what general lessons can be
learned from the observed higher rewards for hetero-
geneous mutual reinforcement than for homogeneous
self-reinforcement?
Last but not least, in order to be able to draw gen-
eral conclusions less dependent on the selection of
strategies in a tournament, we are also pursuing evolv-
ing a population of strategies similar to the approach
found in (Beaufils et al., 1998). We hope to report
new results along those lines in the near future.
REFERENCES
Axelrod, R. (1980). Effective choice in the prisoner’s
dilemma. Journal of Conflict Resolution, 24(1):3 –25.
Axelrod, R. (1981). The evolution of cooperation. Science,
211(4489):1390–1396.
Axelrod, R. (2006). The evolution of cooperation. Basic
Books.
Basu, K. (1994). The traveler’s dilemma: Paradoxes of ra-
tionality in game theory. The American Economic Re-
view, 84(2):391–395.
Basu, K. (2007). The traveler’s dilemma. Scientific Ameri-
can Magazine.
Beaufils, B., Delahaye, J.-P., and Mathieu, P. (1998). Com-
plete classes of strategies for the classical iterated pris-
oner’s dilemma. In Evolutionary Programming, pages
33–41.
Becker, T., Carter, M., and Naeve, J. (2005). Experts play-
ing the traveler’s dilemma. Technical report, Depart-
ment of Economics, University of Hohenheim, Ger-
many.
Capra, C. M., Goeree, J. K., Gmez, R., and Holt, C. A.
(1999). Anomalous behavior in a traveler’s dilemma?
The American Economic Review, 89(3):678–690.
Dasler, P. and Tosic, P. (2010). The iterated traveler’s
dilemma: Finding good strategies in games with
“bad” structure: Preliminary results and analysis. In
Proc of the 8th Euro. Workshop on Multi-Agent Sys-
tems, EUMAS’10.
Dasler, P. and Tosic, P. (2011). Playing challenging iterated
two-person games well: A case study on iterated trav-
elers dilemma. In Proc. of WorldComp Foundations
of Computer Science FCS’11; to appear.
Goeree, J. K. and Holt, C. A. (2001). Ten little treasures
of game theory and ten intuitive contradictions. The
American Economic Review, 91(5):1402–1422.
Halpern, J. Y. and Pass, R. (2009). Iterated regret mini-
mization: a new solution concept. In Proceedings of
the 21st international jont conference on Artifical in-
telligence, IJCAI’09, pages 153–158, San Francisco,
CA, USA. Morgan Kaufmann Publishers Inc.
Land, S., van Neerbos, J., and Havinga, T. (2008). An-
alyzing the traveler’s dilemma Multi-Agent systems
project.
Littman, M. L. (2001). Friend-or-Foe q-learning in General-
Sum games. In Proc. of the 18th Int’l Conf. on Ma-
chine Learning, pages 322–328. Morgan Kaufmann
Publishers Inc.
Neumann, J. V. and Morgenstern, O. (1944). Theory of
games and economic behavior. Princeton Univ. Press.
Osborne, M. (2004). An introduction to game theory. Ox-
ford University Press, New York.
Pace, M. (2009). How a genetic algorithm learns to play
traveler’s dilemma by choosing dominated strategies
to achieve greater payoffs. In Proc. of the 5th interna-
tional conference on Computational Intelligence and
Games, pages 194–200.
Parsons, S. and Wooldridge, M. (2002). Game theory and
decision theory in Multi-Agent systems. Autonomous
Agents and Multi-Agent Systems, 5:243–254.
ICAART 2012 - International Conference on Agents and Artificial Intelligence
80