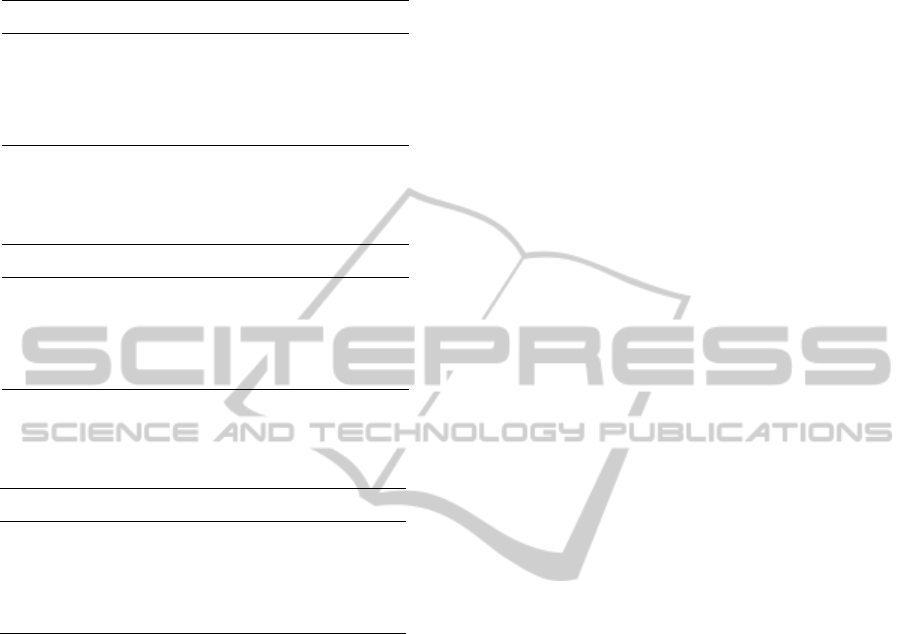
Table 1: Measuring algorithms performance for the
KROABC100 instance with multi - objective optimization
metrics.
GVND SPACING GD IGD
SMOSA 1095 0,0599588 26,5312636 36172,0838
CMOSA 1817 0,04035959 14,3588074 29172,6591
UMOSA 2564 0,03498623 3,4396281 3333,40773
EMOSA 3194 0,03144919 2,38276567 2482,34369
EMODS 34077 0,01365555 0,05108237 129,865643
Table 2: Measuring algorithms performance for the
KROBCD100 instance with multi - objective optimization
metrics.
GVND SPACING GD IGD
SMOSA 1097 0,05749539 25,2829385 33752,4241
CMOSA 1795 0,04647346 14,1155524 28168,342
UMOSA 2472 0,03581236 3,46956584 3227,63056
EMOSA 3143 0,03150265 2,3144672 2321,81408
EMODS 34824 0,01307551 0,04979096 131,915227
Table 3: Average measuring algorithms performance for the
KROABC100 and KROBCD100 instances with multi - ob-
jective optimization metrics.
GVND SPACING GD IGD
SMOSA 1097 0,05749539 25,2829385 33752,4241
CMOSA 1795 0,04647346 14,1155524 28168,342
UMOSA 2472 0,03581236 3,46956584 3227,63056
EMOSA 3143 0,03150265 2,3144672 2321,81408
EMODS 34824 0,01307551 0,04979096 131,915227
only of 0.4 (4%).
Lastly, the metrics values in the table 5 are aver-
aged. It can be seen the superiority of EMODS solu-
tions having the lowest error distance (GD) value of
4.6%. In addition, in all the cases, the values of the
metrics applied to EMODS solutions are distant from
the others and those show the best performance.
5 CONCLUSIONS
A novel metaheuristic named EMODS was proposed.
EMODS is an Evolutionary Metaheuristic to solve
Combinatorial Problems. It is based in the Natu-
ral Selection Theory for avoiding Local Optimums.
Besides, it is based in MODS algorithm to repre-
sent the feasible solution space under the Automata
Theory. Hence, a search direction is used to ex-
plore the feasible solution space (Multiobjective De-
terministic Finite Automata). The proposed algo-
rithm was tested against metaheuristic from the spe-
cialized literature using well known instances from
the TSPLIB. EMODS showed the best performance
in all the metrics worked and in some cases the error
rate of EMODS was 4%.
REFERENCES
Fung, R., Tang, J., and Zhang, J. (2009). A multi-depot
vehicle routing problem with weight-related costs. In
Computers Industrial Engineering, 2009. CIE 2009.
International Conference on, pages 1028 –1033.
Glover, F. and Laguna, M. (1997). Tabu Search. Kluwer
Academic Publishers, Norwell, MA, USA.
Heidelberg, U. O. Tsplib - office research group dis-
crete optimization - university of heidelberg. http://
comopt.ifi.uni-heidelberg.de/software/TSPLIB95/.
Hu, B. and Raidl, G. (2008). Solving the railway traveling
salesman problem via a transformation into the classi-
cal traveling salesman problem. In Hybrid Intelligent
Systems, 2008. HIS ’08. Eighth International Confer-
ence on, pages 73 –77.
Jingyu, Y., Chongguo, L., Zhi, W., Lei, D., and Demin, S.
(2007). Diversity metrics in multi-objective optimiza-
tion: Review and perspective. In Integration Tech-
nology, 2007. ICIT 07. IEEE International Conference
on, pages 553–557.
Li, H. and Landa-Silva, D. (2008). Evolutionary multi-
objective simulated annealing with adaptive and com-
petitive search direction. In Evolutionary Com-
putation, 2008. CEC 2008. (IEEE World Congress
on Computational Intelligence). IEEE Congress on,
pages 3311 –3318.
Lim, A. and Wang, F. (2005). Multi-depot vehicle routing
problem: a one-stage approach. Automation Science
and Engineering, IEEE Transactions on, 2(4):397 –
402.
Ni˜no, E. D., Ardila, C., Donoso, Y., and Jabba, D. (2010). A
novel algorithm based on deterministic finite automa-
ton for solving the mono-objective symmetric travel-
ing salesman problem. International Journal of Arti-
ficial Intelligence, 5(A10):101 – 108.
Ni˜no, E. D., Ardila, C., Donoso, Y., Jabba, D., and Barrios,
A. (2011). Mods: A novel metaheuristic of determin-
istic swapping for the multi objective optimization of
combinatorials problems. Computer Technology and
Application, 2(4):280 – 292.
Oberlin, P., Rathinam, S., and Darbha, S. (2009). A trans-
formation for a heterogeneous, multiple depot, multi-
ple traveling salesman problem. In American Control
Conference, 2009. ACC ’09., pages 1292 –1297.
Pretorius, W. and Helberg, A. (2004). Application of
an adapted evaluation process using numerical amp;
qualitative weighted sum techniques. In AFRICON,
2004. 7th AFRICON Conference in Africa, volume 1,
pages 367 –372 Vol.1.
Sauer, J. and Coelho, L. (2008). Discrete differential evolu-
tion with local search to solve the traveling salesman
problem: Fundamentals and case studies. In Cyber-
netic Intelligent Systems, 2008. CIS 2008. 7th IEEE
International Conference on, pages 1 –6.
EMODS: A NOVEL EVOLUTIONARY METAHEURISTIC BASED IN THE AUTOMATA THEORY FOR THE
MULTIOBJECTIVE OPTIMIZATION OF COMBINATORIALS PROBLEMS
403