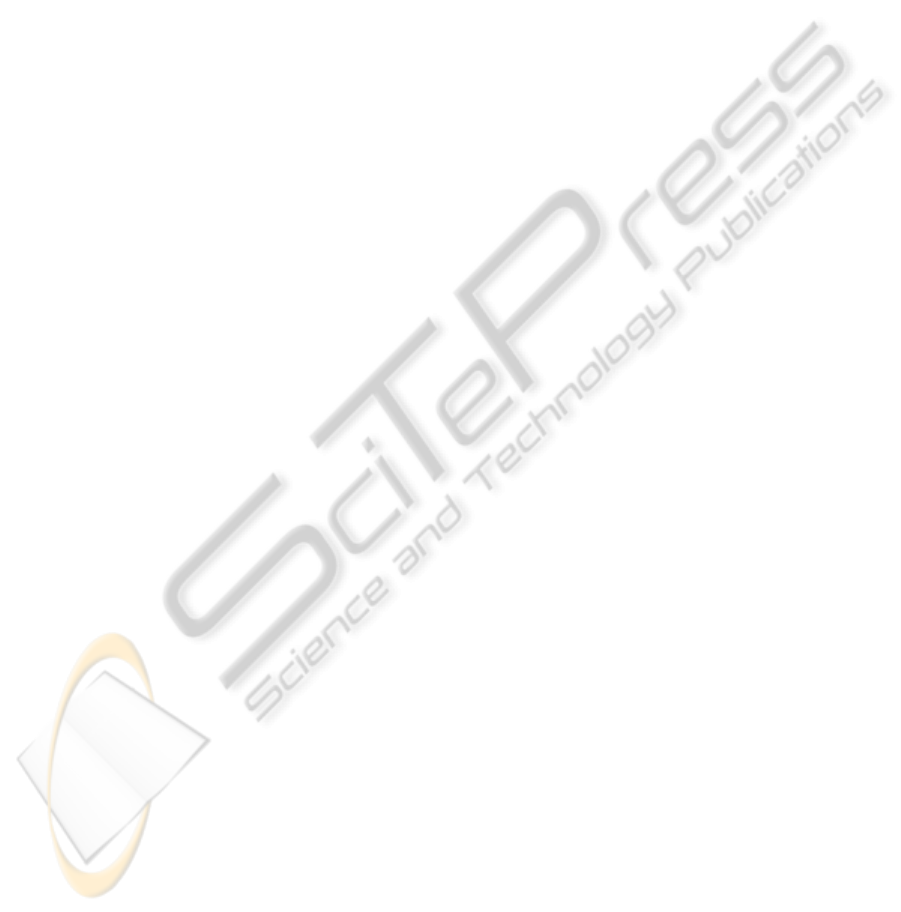
representation approach for classification of moving
vehicles. We have made a successful attempt to
explore the applicability of symbolic data concepts
to classify the traffic vehicles. The newly presented
representation model has an ability to capture the
variations of the features among the training sample
vehicles. In the proposed method, we get a number
of feature vectors which is equivalent to the number
of vehicle categories. Our proposed approach is able
to deal with different types of deformations on the
shape of vehicles even in cases of change in size,
direction and viewpoint. Results show the robustness
and efficiency of our classification model.
REFERENCES
Buch, Norbert, Yin, Fei, Orwell, James, Makris,
Dimitrios, Velastin, Sergio A., 2009. Urban Vehicle
Tracking using a Combined 3D Model Detector and
Classifier. In 13th International Conference on
Knowledge-Based and Intelligent Information &
Engineering Systems, vol. 5711, pp. 169-176.
Chen, X., Zhang, Ch., 2007. Vehicle Classification from
Traffic Surveillance Videos at a Finer Granularity. In
13th International Multimedia Modeling Conference,
part I, pp. 772-781.
Costa, Luciano da Fontoura, Cesar, Roberto Marcondes,
2001. Shape Analysis and Classification, CRC Press
LLC, USA.
Dallalzadeh, Elham, Guru, D. S., Manjunath, S., Suraj, M.
G., 2011. Corner-Based Tracking of Moving Vehicles
in Traffic Videos. In 5th Indian International
Conference on Artificial Intelligence.
Gowda, K. C., Diday, E., 1991. Symbolic Clustering using
a New Dissimilarity Measure. Pattern Recognition,
vol. 24(6), pp. 567-578.
Gupte, S., Masoud, O., Martin, R. F. K., Papanikolopou-
los, N.P., 2002. Detection and Classification of
Vehicles. IEEE Transactions on Intelligent Transpor-
tation Systems, vol. 3(1), pp. 37-47.
Guru, D. S., Nagendraswamy, H. S., 2007. Symbolic Re-
presentation of Two-Dimensional Shapes. Pattern
Recognition Letters, vol. 28 (1), pp. 144-155.
Guru, D. S., Prakash, H. N., 2009. Online Signature
Verification and Recognition: An Approach Based on
Symbolic Representation. IEEE Transactions on
Pattern Analysis and Machine Intelligence, vol. 31(6),
pp. 1059-1073.
Hsieh, J. W., Yu, S. H., Chen, Y. S, Hu, W. F., 2006.
Automatic Traffic Surveillance System for Vehicle
Tracking and Classification. IEEE Transactions on
Intelligent Transportation Systems, vol. 7(2), pp. 175-
188.
Maurin, B., Masoud, O., Papanikolopoulos, N., 2002.
Monitoring Crowded Traffic Scenes. In 5th Interna-
tional Conference on Intelligent Transportation Sys-
tems, pp. 19-24.
Maurin, B., Masoud, O., Papanikolopoulos, N. P., 2005.
Tracking All Traffic: Computer Vision Algorithms for
Monitoring Vehicles, Individuals, and Crowds. In
Robotics and Automation Magazine, vol. 12(1), pp.
29-36.
Ottlik, A., Nagel, H.-H, 2008. Initialization of Model-
Based Vehicle Tracking in Video Sequences of Inner-
City Intersections. International Journal of Computer
Vision, vol. 80(2), pp. 211-225.
Techmer, A., 2001. Contour-Based Motion Estimation and
Object Tracking for Real-Time Applications. In
International Conference on Image Processing, pp.
648-651.
Ticiano, A. C. B., Gabriel, I. S. R., Victor, A. P. B,
Marcus, V. L., Daniel, A., George, L. T., Yaeko, Y.,
2008. A New Approach to Multiple Vehicle Tracking
in Intersections using Harris Corners and Adaptive
Background Subtraction. In Intelligent Vehicles
Symposium Eindhoven University of Technology
Eindhoven, pp. 548-553.
Wu, W., Zhang, Q., Wang, M., 2001. A Method of
Vehicle Classification using Models and Neural
Networks. In Vehicular Technology Conference
, vol.
4, pp. 3022-3026.
Zhang, Ch., Chen, X., Chen, W.-bang, 2006. A Pca-Based
Vehicle Classification Framework. In 22nd Interna-
tional Conference on Data Engineering Workshops,
pp. 17-26.
Zhang, D., Lu, G., 2003. A Comparative Study on Shape
Retrieval using Fourier Descriptors with Different
Shape Signatures. Journal of Visual Communication
and Image Representation, vol. 14 (1), pp. 41-60.
ICPRAM 2012 - International Conference on Pattern Recognition Applications and Methods
356