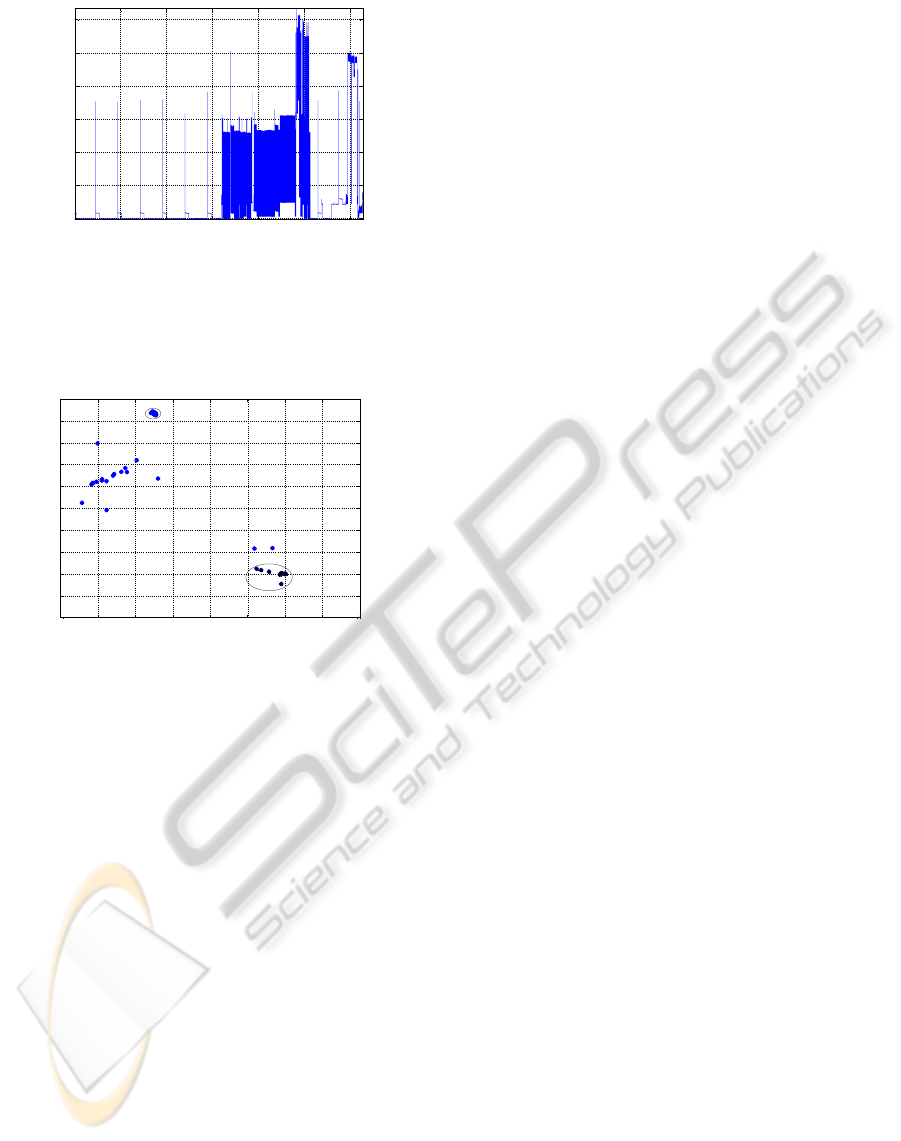
0 2 4 6 8 10 12
0
500
1000
1500
2000
2500
3000
time [h]
|S| [VA]
Figure 7: Measurement for the analysis of the ANN.
from the other events. Additional clusters show up,
one of which representing the oven which was oper-
ated from 6:30 to 10:15. For our future work the ANN
-60 -50 -40 -30 -20 -10 0 10 20
-20
-10
0
10
20
30
40
50
60
70
80
y
1
y
2
Refrigerator
Oven
Figure 8: Outputs of the ANN.
is to be applied to additional measurements. This will
show if the ANN method is suitable for the classi-
fication of other equipment. An option for increas-
ing the classification accuracy and classification abil-
ity is to increase the dimension of the output vector y
from currently two to higher values. In order to use
the ANN method in time variant systems an adaptive
learning algorithm might be used.
7 CONCLUSIONS
This paper provides an overview of the state of the art
of the area of NALM. The research project with the
goal to find methods for the identification of individ-
ual appliances in the load profile of private apartments
and houses was presented. This project is divided
into four parts, one of which is to develop disaggre-
gation algorithms which was shown in more detail. A
method for the detection of periodically switching ap-
pliances was discussed. This method is based on arti-
ficial neural networks. The description of the method
was accompanied by first simulation results.
REFERENCES
Baranski, M. (2006). Energie-Monitoring im privaten
Haushalt. In Ph.D. Thesis, University of Paderborn.
Benyoucef, D., Bier, T., and Klein, P. (2010a). Plan-
ning of energy production and management of energy
resources with SmartMeters. In International Con-
ference on Advances in Energy Engineering (ICAEE
2010), pages 170–173, Beijing, China. IEEE.
Benyoucef, D., Klein, P., and Bier, T. (2010b). Smart Meter
with Non-Intrusive Load Monitoring for Use in Smart
Homes. In IEEE Energy 2010. IEEE.
Benyoucef, D., Klein, P., and Bier, T. (2010c). Smart me-
tering: Disaggregation von endverbrauchern. In VDE-
Kongress 2010. Smart Cities, Leipzig. VDE.
Bertoldi, P. and Atanasiu, B. (2006). Electricity consump-
tion and efficiency trends in the enlarged European
Union. In Status Report, Institute for Environment
and Sustainability. JRC European Commission.
Cichocki, A. and Unbehauen, R. (1993). Neural networks
for optimization and signal processing. Stuttgart :
Teubner, Chichester u.a.: Wiley, 1993.
EU (2009). Electricity Consumption and Efficiency Trends.
In JRCTechnicalReport. EU.
Hart, G. (1992). Non-intrusive Appliance Load Monitoring.
In JRC Technical Report, pages 1870–1891, Proceed-
ings of the IEEE. IEEE.
Haykin, S. (2002). Adaptive filter theory. Prentice-
Hall, Great Britain: Pearson Education International,
c2002.
Laughman, C., Lee, K., Cox, R., Shaw, S., Leeb, S., Nor-
ford, L., and Armstrong, P. (2003). Power signature
analysis. In Power and Energy Magazine, pages 56–
63. IEEE.
Lee, K., Norford, L., and Leeb, S. (2003). Development of
a functioning centrally located electrical-load monitor.
In Technical Report. MIT.
Murata, H. and Onoda, T. (2000). Applying support vector
machines and boosting to a non-intrusive monitoring
system for household electric appliances with invert-
ers.
Murata, H. and Onoda, T. (2002). Estimation of power
consumption for household electric appliances. In 9th
International Conference on Neural Information Pro-
cessing.
Najmeddine, H., Drissi, K. K., Pasquier, C., Faure, C., Ker-
roum, K., Diop, A., Jouannet, T., and Michou, M.
(2008). State of art on load monitoring methods. In
2nd IEEE International Conference on Power and En-
ergy (PECon 08), Johor Baharu, Malaysia.
NRW, E. (2006). Durchschnittliche stromkosten gemittelt
ber alle haushaltsgren. Presseinfo vom 06.04.2006.
Pihala, H. (1998). Non-intrusive appliance load monitoring
sytem based on modern kwh-meter. Technical report,
Technical Research Center of Finland.
Shaw, S. (2000). System identification and modeling for
nonintrusive load diagnostics. In Ph.D. Thesis. Mas-
sachusetts Institute of Technology.
ICPRAM 2012 - International Conference on Pattern Recognition Applications and Methods
550