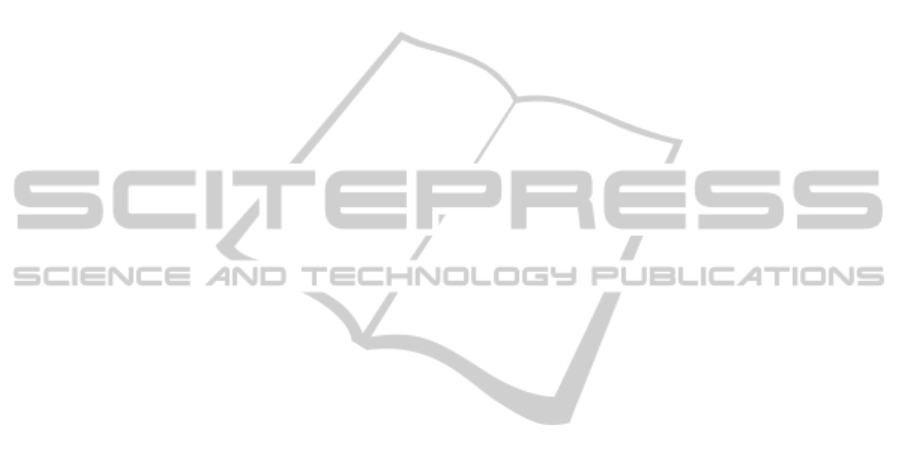
lead to many pedestrians switching routes simulta-
neously which decreases the efficiency with which
pedestrians are able to evacuate the building. In ev-
ery building layout tested, when pedestrians have only
learned distance information, the performance is the
worst of all possibilities considered. Interestingly
though, a pedestrian having system information but
ignoring current congestion levels and using only dis-
tance information is able to egress from most build-
ings quickly. However, the distance information of
such a pedestrian is complete. One would expect that
with enough training, pedestrians having learned only
distance cost would also be able to egress from build-
ings with similar efficiency.
7 CONCLUSIONS
Providing agents with perfect knowledge is unrealis-
tic for many pedestrian egress situations. However,
manually specifying specific route knowledge can be
a difficult and time-consuming task. We have shown
that reinforcement learning can be applied to success-
fully represent different levels of knowledge about a
building layout and produces egress times dependent
upon the knowledge level of the pedestrians. We have
also provided three different metrics for measuring
the amount of building knowledge an agent has.
Using reinforcement learning, we have also shown
that learning congestion cost in addition to distance
costs leads to quicker egress times. However, reacting
to current congestion levels has ambiguous results.
This is consistent with similar studies in the traffic
management domain. The layout of the building is
found to have an impact on the strategy a pedestrian
should use to minimize egress time.
REFERENCES
Antonini, G., Bierlaire, M., and Weber, M. (2006). Dis-
crete choice models of pedestrian walking behav-
ior. Transportation Research Part B: Methodological,
40(8):667–687.
Banerjee, B., Bennett, M., Johnson, M., and Ali, A.
(2008). Congestion avoidance in multi-agent-based
egress simulation. In IC-AI, pages 151–157.
Barnes, M., Leather, H., and Arvind, D. (2007). Emer-
gency evacuation using wireless sensor networks. Lo-
cal Computer Networks, Annual IEEE Conference on,
0:851–857.
Dia, H. (2002). An agent-based approach to modelling
driver route choice behaviour under the influence of
real-time information. Transportation Research Part
C: Emerging Technologies, 10(5-6):331–349.
Feuz, K. (2011). Pedestrian leadership and egress assistance
simulation environment. Master’s thesis, Utah State
University.
Gwynne, S., Galea, E. R., Lawrence, P. J., and Filippidis, L.
(2001). Modelling occupant interaction with fire con-
ditions using the buildingexodus evacuation model.
Fire Safety Journal, 36(4):327–357.
Helbing, D. and Johansson, A. (2009). Pedestrian,
crowd and evacuation dynamics. In Encyclopedia of
Complexity and Systems Science, pages 6476–6495.
Springer.
Helbing, D., Sch
¨
onhof, M., Stark, H., and Holyst, J. (2005).
How individuals learn to take turns: Emergence of al-
ternating cooperation in a congestion game and the
prisoner’s dilemma. Advances in Complex Systems,
8(1):87–116.
Hoogendoorn, S. and Bovy, P. (2004). Pedestrian route-
choice and activity scheduling theory and models.
Transportation Research Part B: Methodological,
38(2):169–190.
Kaelbling, L., Littman, M., and Moore, A. (1996). Rein-
forcement learning: A survey. Journal of Artificial
Intelligence Research, 4:237–285.
Koh, W. L., Lin, L., and Zhou, S. (2008). Modelling and
simulation of pedestrian behaviours. In PADS ’08:
Proceedings of the 22nd Workshop on Principles of
Advanced and Distributed Simulation, pages 43–50,
Washington, DC, USA. IEEE Computer Society.
Kray, C., Kortuem, G., and Kr
¨
uger, A. (2005). Adaptive
navigation support with public displays. In Proceed-
ings of the 10th international conference on Intelligent
user interfaces, IUI ’05, pages 326–328, New York,
NY, USA. ACM.
M
¨
uller, J., Jentsch, M., Kray, C., and Kr
¨
uger, A. (2008).
Exploring factors that influence the combined use of
mobile devices and public displays for pedestrian nav-
igation. In Proceedings of the 5th Nordic conference
on Human-computer interaction: building bridges,
NordiCHI ’08, pages 308–317, New York, NY, USA.
ACM.
Ozel, F. (2001). Time pressure and stress as a factor during
emergency egress. Safety Science, 38(2):95–107.
Pan, X. (2006). Computational Modeling of Human and
Social Behaviors for Emergency Egress Analysis. PhD
thesis, Stanford University, Stanford, California.
PTV AG (2011). http://www.ptvag.com/software/transport-
ation-planning-traffic-engineering/software-system-
solutions/vissim-pedestrians/. Accessed on: April 4,
2011.
Roughgarden, T. and Tardos,
´
E. (2002). How bad is selfish
routing? Journal of the ACM (JACM), 49(2):236–259.
Santos, G. and Aguirre, B. E. (2004). A critical review of
emergency evacuation simulation models. In Building
Occupant Movement during Fire Emergencies.
Wahle, J., Bazzan, A., Kl
¨
ugl, F., and Schreckenberg, M.
(2002). The impact of real-time information in a two-
route scenario using agent-based simulation. Trans-
portation Research Part C: Emerging Technologies,
10(5-6):399–417.
SIMULATING KNOWLEDGE AND INFORMATION IN PEDESTRIAN EGRESS
253