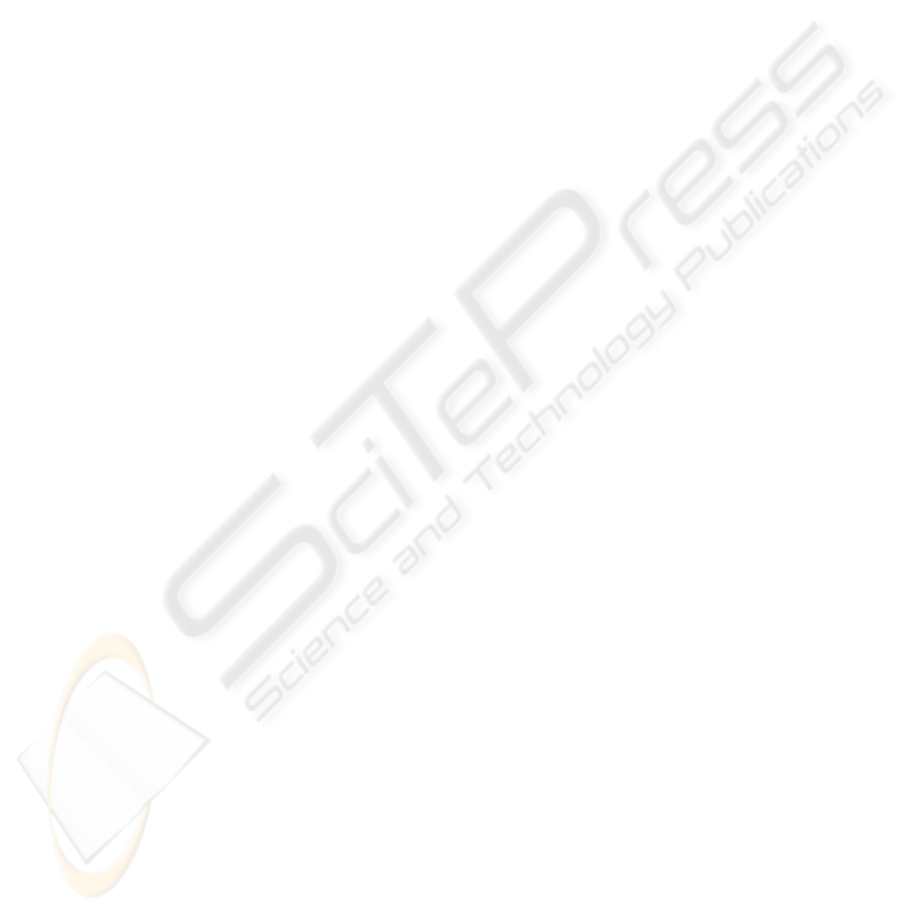
DETECTION AND RECOGNITION OF SUBPIXEL TARGETS
WITH HYPOTHESES DEPENDENT BACKGROUND POWER
Victor Golikov and Olga Lebedeva
Ingineering Faculty, Autonomous University of Carmen, 56 st., No. 4, Ciudad del Carmen, Camp., Mexico
Keywords: Statistical Detection and Recognition, Subpixel Targets.
Abstract: We consider the problem of detecting and recognizing the subpixel targets in sea background when the
background power may be different under the null hypothesis – where it is assumed to be known – and the
alternative multiple hypotheses. This situation occurs when the presence of the target triggers a decrease in
the background power (subpixel targets). We extend the formulation of the Matched Subspace Detector
(MSD) to the case where the background power is only known under the null hypothesis using the
generalized likelihood ratio test (GLRT) for the multiple hypotheses case. The obtained multiple hypotheses
test is based on the Modified MSD test (MMSD). We discuss the difference between the two detection and
recognition systems: based on the MSD and MMSD tests. Numerical simulations attest to the validity of the
performance analysis.
1 INTRODUCTION
Among the various frameworks in which pattern
recognition has been traditionally formulated, the
statistical approach has been most intensively
studied and used in practice (Webb, 2002). Target
detection and recognition in the remotely sensed
image sequences can be conducted spatially,
temporally or spectrally. The need for subpixel
temporally (or spectrally) detection-recognition in
remotely sensed image sequences arises from the
fact that the targets sampling distances are generally
larger than the sizes of targets of interest. In this
case, the target is embedded in a single pixel
sequence and cannot be detected or recognized
spatially. As a result, traditional spatial-temporal
analysis-based image sequence processing
techniques are not applicable. Matched subspace
detection-recognition is used to recognize the
mulptiple hypotheses of different targets presence or
absence of targets that are expected to lie in
particular subspaces of the measurements. Standard
approach in this case bases on calculating for each
possible target of the GLR and determination of the
target with maximum value of the GLR (Izenman,
2008). The common drawback of this approach is
the assumption that the background power under
hypothesis H
0
remains the same one as under
hypotheses H
k
. In digital optical systems, it is
typically that the background has the same
covariance structure under hypotheses H
0
and H
k
, but
different variances (Manolakis and Shaw, 2002),
which is directly related to the fill factor, that is, the
percentage of the pixel area occupied by the
background. Because the background power is
changed if any of the targets is present, the
detection-recognition system is not optimum and,
therefore, it is necessary to modify the MSD
(Golikov, Lebedeva 2011). As a result, we assume
that the proposed detection-recognition system can
achieve a significant performance advantage against
conventional one.
In this paper, we focus on the detection-
recognition of small targets in the case of unknown
power of Gaussian background under hypothesis H
i
.
We assume that different targets have the different
subspace dimensions. In section 2, we formulate the
subpixel detection-recognition problem using the
linear mixing model and the concepts of targets and
background subspaces. We derive the GLRT for the
problem at hand and the distributions under the
hypotheses. In Section 3, we investigated the
detection-recognition performance losses in the case
of background power variations between multiple
hypotheses in a Gaussian environment for proposed
and canonical detection-recognition systems in the
presence of a mismatch between the designed and
actual background power. Here, the numerical
simulations are included to verify the validity of the
555
Golikov V. and Lebedeva O. (2012).
DETECTION AND RECOGNITION OF SUBPIXEL TARGETS WITH HYPOTHESES DEPENDENT BACKGROUND POWER.
In Proceedings of the 1st International Conference on Pattern Recognition Applications and Methods, pages 555-558
DOI: 10.5220/0003756405550558
Copyright
c
SciTePress