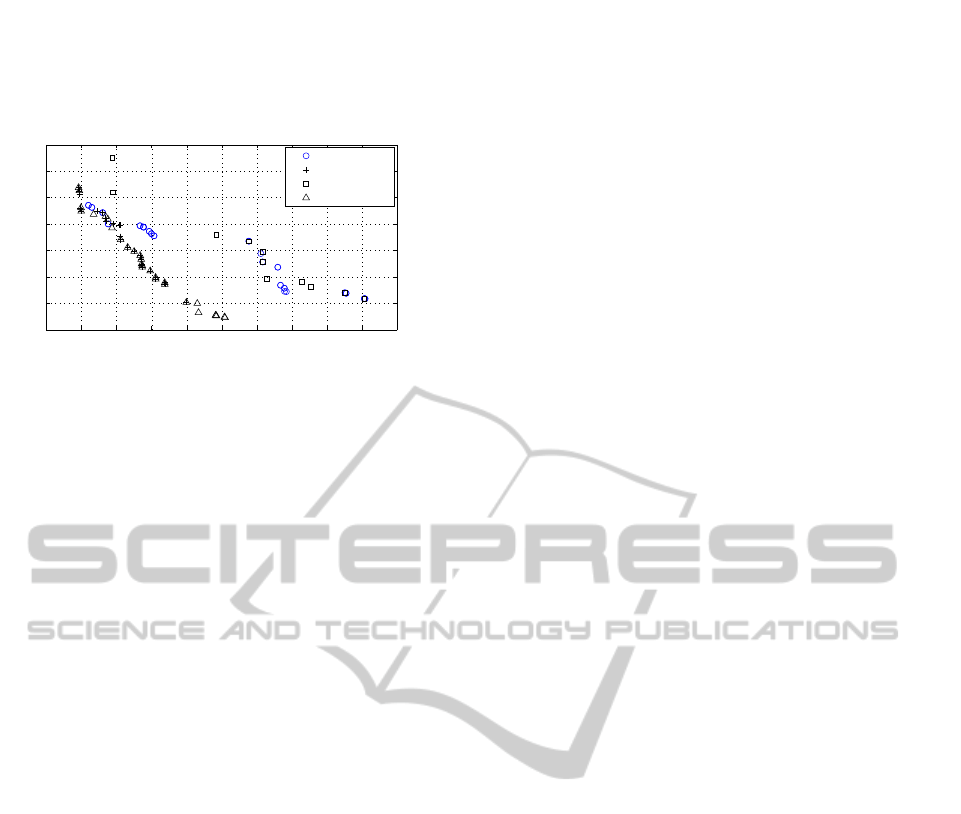
7.5 7.6 7.7 7.8 7.9 8 8.1 8.2 8.3 8.4 8.5
x 10
5
4
4.5
5
5.5
6
6.5
7
7.5
x 10
5
Fuel cost (x1000) [monetary units]
NOx concentration (x10000) [mg/Nm
3
]
NSGA
SPEA
NPGA
BRKGA.NSGA
Figure 3: Pareto-optimal fronts obtained from from differ-
ent algorithms in a single run.
nates 88.5% of the nondominated solutions found
by NSGA II, while the front obtained by NSGA II
dominates less than 11 % of the nondominated solu-
tions produced by BRKGA. Moreover, the BRKGA
front dominates on average 75% of the correspond-
ing NPGA front while the nondominatedset produced
by NPGA dominates less than 22.3 % of the non-
dominated solutions produced by BRKGA. Finally,
SPEA2 front dominates on average 84.8% of the cor-
respondingBRKGA front while the nondominated set
produced by BRKGA dominates 30.3 % of the non-
dominated SPEA2 solutions.
5 CONCLUSIONS
In this paper a new approach is used to find Pareto sets
for multiobjective unit commitment problem. The
proposed algorithm combines the biased selection and
biased crossover of the BRKGA approach with non-
dominated sorting procedure and crowded compari-
son operator used in NSGA II technique.
The algorithm maintains a finite-sized archive of
nondominated solutions which gets iteratively up-
dated in the presence of new solutions based on the
concept of pareto dominance. The multiple Pareto
optimal solutions can be found in one simulation run
such as in other multiobjective techniques.
The proposed approach has been assessed through
a comparative study, for two case study problems,
with other multiobjective optimization techniques.
The best results are obtained for SPEA2 and BRKGA
approachs. The results shows that BRKGA can be
an effective method for producing tradeoff curves.
Tradeoff curves such as those presented here may give
decision makers the capability of making better deci-
sions. Given that the approachs have similar decode
procedures, the improvement in performance is most
likely due to elitism. Elitism also guarantees that no
good solutions are lost.
ACKNOWLEDGEMENTS
The financial support by FCT, POCI 2010
and FEDER, through project PTDC/EGE-
GES/099741/2008 is gratefully acknowledged.
REFERENCES
Abido, M. A. (2003a). Environmental/economic power dis-
patch using multiobjective evolutionary algorithms.
IEEE Trans. Power Syst., ‘18:‘1529–1537.
Abido, M. A. (2003b). A niched pareto genetic algorithm
for multiobjective environmental/economic dispatch.
Electr. Power Energy Syst., ‘25:97–105.
Abido, M. A. (2003c). A novel multiobjective evolution-
ary algorithm for environmental/economic power dis-
patch. Electr. Power Syst. Res., ‘65:‘71–81.
Abido, M. A. (2006). Multiobjective evolutionary algo-
rithms for electric power dispatch problem. IEEE
Transactions on Evolutionary Computation, ‘10:315–
329.
Basu, M. (2008). Dynamic economic emission dispatch us-
ing non-dominated sorting genetic algorithm-ii. Elec-
tric Power Energy System, 30:140–149.
Deb, K. and Agrawal, R. B. (1995). Simulated binary
crossover for continuous search space. Complex Sys-
tems, 9:115–148.
Deb, K., Pratab, A., Agarwal, S., and Meyarivan, T. (2002).
A fast and elitist multiobjective genetic algorithm:
Nsga-ii. IEEE Trans. Evol. Comput., 6:182–197.
Gonc¸alves, J. F. and Resende, M. G. C. (2010). Biased
random-key genetic algorithms for combinatorial op-
timization. Journal of Heuristics, 17:487–525.
Granelli, G. P., Montagna, M., Pasini, G. L., and Maran-
nino, P. (1992). Emission constrained dynamic dis-
patch. ‘Electr. Power Syst. Res., ‘24:‘56–62.
Horn, J., Nafpliotis, N., and Goldberg, D. E. (1994). A
niched pareto genetic algorithm for multiobjective op-
timization. In 1st IEEE Conf. Evol. Comput., IEEE
World Congr. Comput. Intell., volume 1, pages 67–72.
Hsiao, Y. T., Chiang, H. D., Liu, C. C., and Chen, Y. L.
(1994). A computer package for optimal multiobjec-
tive var planning in large scale power systems. IEEE
Trans. Power Syst., ‘9:‘668–676.
Roque, L., Fontes, D. B. M. M., and Fontes, F. A. C. C.
(2011). A biased random key genetic algorithm ap-
proach for unit commitment problem. Lecture Notes
in Computer Science, 6630:327–339.
Sawaragi, Y., Nakayama, H., and Tanino, T. (1985). Theory
of multiobjective optimization. Orlando: Academic
Press.
Srinivas, N. and Deb, K. (1994). Multiobjective function
optimization using nondominated sorting genetic al-
gorithms. Evol. Comput., 2:221–248.
Wang, S., Shahidehpour, M., Kirschen, D. S., Mokhtari,
S., and Irissari, G. (1995). Short-term generation
scheduling with transmission and environmental con-
straints using an augmented lagrangian relaxation.
IEEE Trans Power Systems, ‘10:1294–300.
BRKGA ADAPTED TO MULTIOBJECTIVE UNIT COMMITMENT - Solving Pareto Frontier for UC Multiobjective
Problem using BRKGA SPEA2 NPGA and NSGA II Techniques
71