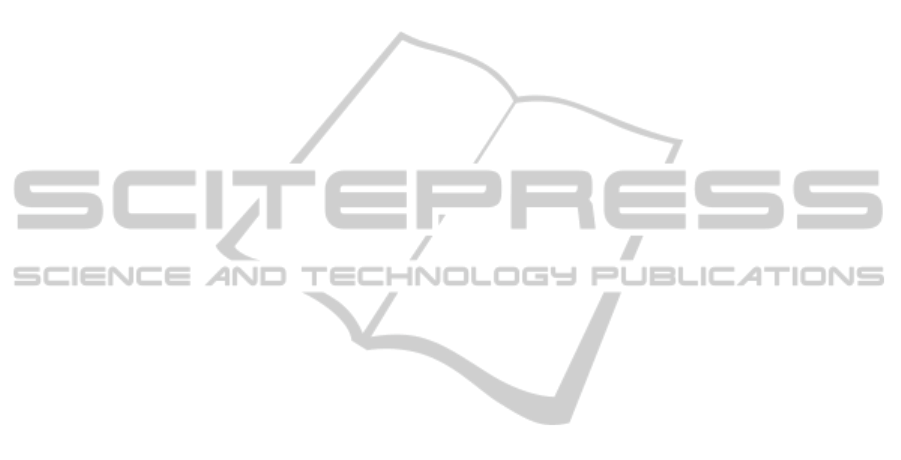
reach each one some muscle strength is applied.
Another difference is the use of amplitude envelope
instead of some other feature combination in time or
frequency domain. This may be the reasons to the
smaller classification accuracies for a number of
classes greater than 5 compared to the results
obtained from other authors, which continue with
classification rates above 90 for these class
configurations (Hargrove, Englehart and Hudgins,
2007; Ahsan, Ibrahimy and Khalifa, 2010; Basu,
Bhattacharyya and Kim, 2010; Scheme and
Englehart, 2011). However, considering the type of
movement and distinctive classes, the low level of
contraction and the use of the amplitude envelope,
which require a minimum processing effort, for a
small number of classes, the systems had performed
well.
On the other hand, the process based on RLDA
was very fast, while the process based on MLP was
time consuming as much to define adequate
parameters as for as network training. There was no
pattern for the number of hidden layers and the
number of neurons. These parameters varied for each
volunteer and for each class configuration, aimed at
obtaining the best classification accuracies. Usually, 2
or 3 hidden layers were enough, but the number of
neurons varied from 9 to 100 depending on the case.
Englehart et al. (1999) and other researchers such as
Basu, Battacharyya and Kin (2010) and Zhang (2000)
defend that MLP, as long as properly trained and with
an appropriate configuration will always match, if not
exceed, the performance of an RLDA. But usually,
due to the need to automate MLP training over a large
number of interactions, the number of hidden layers
and also the number of neurons are fixed. For a given
subject and a specific number of classes however, the
configuration may be inappropriate, and will be
inhibit the generalization performance of the MLP.
The RLDA, on the other hand does not require these
specifications, and performs very well.
5 CONCLUSIONS
This study showed the same performance for RLDA
and MLP in a problem of elbow angular position
classification, based on the SME amplitude envelope.
Both methods achieved average classification
accuracies above 80% for a number of classes until 4
but individually, 5 subjects achieved similar results in
a 5-class setup, which means a 20o shift between
consecutive classes. May be a better classification
accuracy can be reached with another feature instead
of amplitude envelope that was used. However, this
probably will also change the comparative
performance between the methods. Considering that
for MLP there is a great effort to define the
architecture and also learning parameters, its use is
only justified if there is a need of generalization that
cannot be achieved by the RLDA that does not
require the predefinition of parameters, it is practical
and fast.
ACKNOWLEDGEMENTS
The author thanks FEI and FAPESP for sponsoring.
REFERENCES
Ahsan, Md. R., Ibrahimy, M. I., and Khalifa ,O. O. (2010).
Advances in Electromyogram Signal Classification to
Improve the quality of Life for the Disable and Aged
People. J. Comput Sci., 6(7), 705-715.
Basu, J. K., Bhattacharyya, D. and Kim, T. (2010). Use of
Artificial Neural Network in Pattern Recognition. Int. J.
Software Eng. And Its Applications, 4(2),
Castro, M. C. F. (2011). Statistical Approach for Angular
Position Separability Classes of EMG Data. Proc.
ISSNIP Biosignals and Biorobotics Conferenc., Vitoria,
Brazil. DOI: 10.1109/BRC.2011.5740663.
Cheng, B. and Titterington, D. M. (1994). Neural
Networks: A Review from a Statistical Perspective.
Statistical Sci., 9(1), 2-30.
Englehart, K., Hudgins, B., Parker, P. A., and Stevenson,
M. (1999). Classification of the myoelectric signal
using time-frequency based representations. Med. Eng.
Phys. – Especial Issue on Intell. Data Anal.
Electromyog. Electroneurog., 21, Jul., 431-438.
Guo, Y., Hastie, T., and Tibshirani, R. (2007). Regularized
linear discriminant analysis and its application in
microarrays. Biostat., 8(1), 86-100.
Hargrove, L. J., Englehart, K. and Hudgins, B. (2007). A
Comparison of Surface and Intramuscular Myoelectric
Signal Classification. IEEE Trans. Biom. Eng., 54(5),
847-853.
Itakura, N., Kinbara, Y., Fuwa, T. and Sakamoto, K.
(1996). Discrimination of Forearm’s Motions by
Surface EMG Signals using Neural Network. Applied
Human Science – J. Physiological Anthropology, 15(6),
287-294.
Oskoei, M.A. and Hu, H. (2006). GA-based Feature Subset
Selection for Myoelectric Classification. Proc. IEEE
Int. Conf. Robotics and Biomimetics, China, 1465-1470.
Parker, P., Englehart, K., and Hudgins, B. (2006).
Myoelectric signal processing for control of powered
limb prostheses. J. Electromyog and Kinesiol., 16, 541-
548.
BIOSIGNALS 2012 - International Conference on Bio-inspired Systems and Signal Processing
354