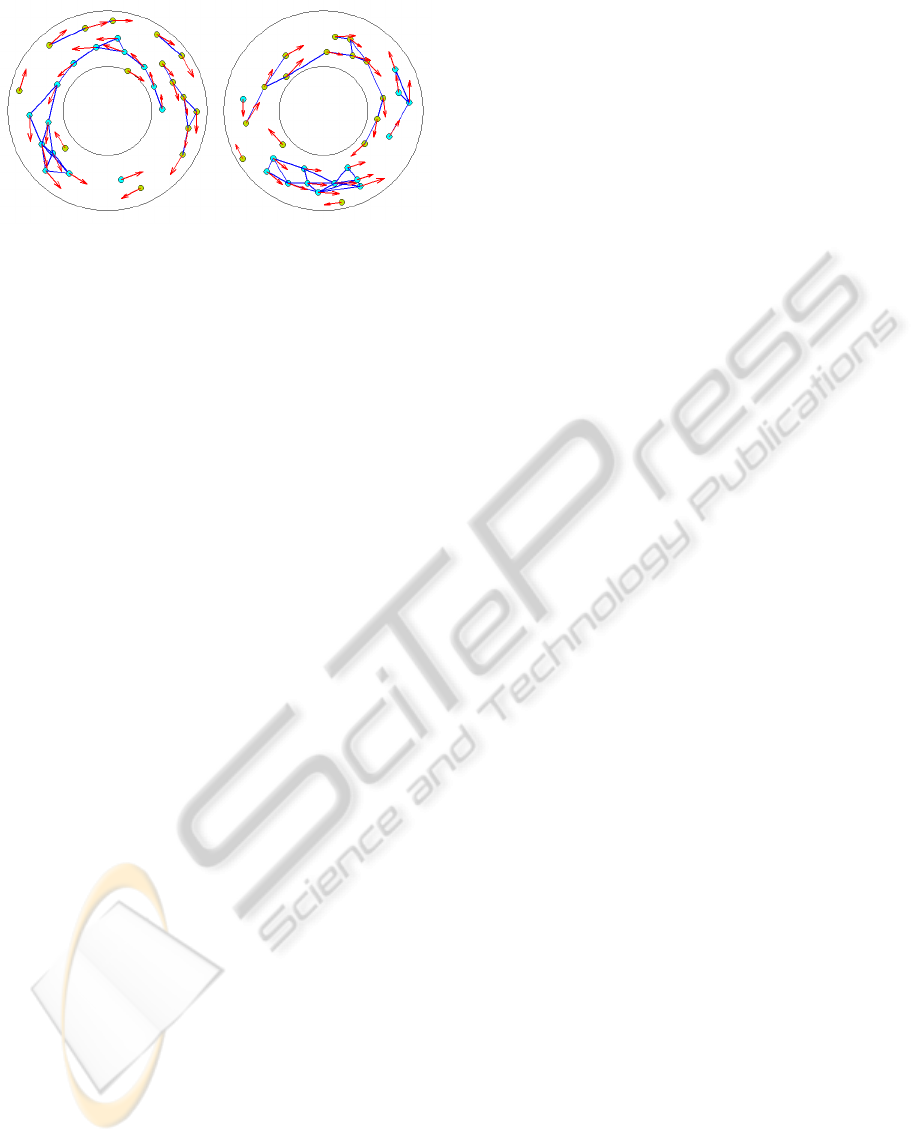
Figure 5: Comparison between two situations occurring
within the same experimental session (N = 30 pedestrians).
The first situation (left graph) is clearly asymmetric in its or-
derliness: counter-clockwise pedestrians are all in the same
lane but one, whereas clockwise pedestrians form several
small lanes. At this moment β
c
= 0.38 and β
cc
= 0.91. The
second situation (right graph) is more balanced: β
c
= 0.73
and β
cc
= 0.70.
and a time scale. Indeed, its originality lies in tak-
ing time into account to detect the formation and the
break-up of lanes. Moreover, sensitivity studies show
that the method is robust to parameter variations as
long as their values are high enough.
In addition, we designed a universal order index
which can prove to be very useful in both experimen-
tal and numerical data of complex systems. Being
based on the concept of statistical entropy, it ensures
a measure of order in a very general sense, and is eas-
ily transferable to different contexts and studies in the
complex systems field.
Future work will include the application of these
tools to systems with a large number of agents,
namely trail formation in simulated and experimental
ant colonies.
ACKNOWLEDGEMENTS
This study was supported by a research grant from the
PEDIGREE project funded by the French National
Research Agency (Grant No ANR-08-SYSC-015).
REFERENCES
Casellas, E., Gautrais, J., Fournier, R., Blanco, S., Combe,
M., Fourcassi, V., Theraulaz, G., and Jost, C. (2008).
From individual to collective displacements in het-
erogenous environments. Journal of Theoretical Bi-
ology, 250: 424-434.
Couzin, I. and Franks, N. (2003). Self-organized lane for-
mation and optimized traffic flow in army ants. Pro-
ceedings of the Royal Society B: Biological Sciences
270(1511):139-146.
Couzin, I. and Krause, J. (2003). Self-organization and col-
lective behavior in vertebrates. Advances in the Study
of Behavior 32:1-75.
Helbing, D., Buzna, L., Johansson, A., and Werner, T.
(2005). Self-organized pedestrian crowd dynam-
ics: Experiments, simulations, and design solutions.
Transportation Science 39(1):1-24.
Helbing, D. and Molnar, P. (1995). Social force model for
pedestrian dynamics. Physical Review E. 51(5):4282-
4286.
Helbing, D., Molnar, P., Farkas, I., and Bolay, K. (2001).
Self-organizing pedestrian movement. Environment
and Planning B: Planning and Design 28:361-383.
Kretz, T., Gr¨unebohm, A., Kaufman, M., Mazur, F., and
Schreckenberg, M. (2006). Experimental study of
pedestrian counterflow in a corridor. Journal of Sta-
tistical Mechanics: Theory and Experiments P10001.
Moussa¨ıd, M., Helbing, D., and Theraulaz, G. (2011). How
simple rules determine pedestrian behavior and crowd
disasters. Proceedings of the National Academy of
Sciences 108(17):6884-6888.
Older, S. (1968). Movement of pedestrians on footways
in shopping streets. Traffic Engineering and Control
10:160-163.
Rex, M. and Lwen, H. (2007). Lane formation in oppositely
charged colloids driven by an electric field: Chaining
and two-dimensional crystallization. Physical Review
E (Statistical, Nonlinear, and Soft Matter Physics) 75.
Sumpter, D. (2010). Collective animal behavior. Princeton
University Press.
Wolfram, S. (1984). Universality and complexity in cellular
automata. Physica 10D, 1-35.
Yamori, K. (1998). Going with the flow: micro-macro dy-
namics in the macrobehavioral patterns of pedestrian
crowds. Psychological Review, Vol. 105, No.3, 530-
557.
LANE DETECTION IN PEDESTRIAN MOTION AND ENTROPY-BASED ORDER INDEX
197