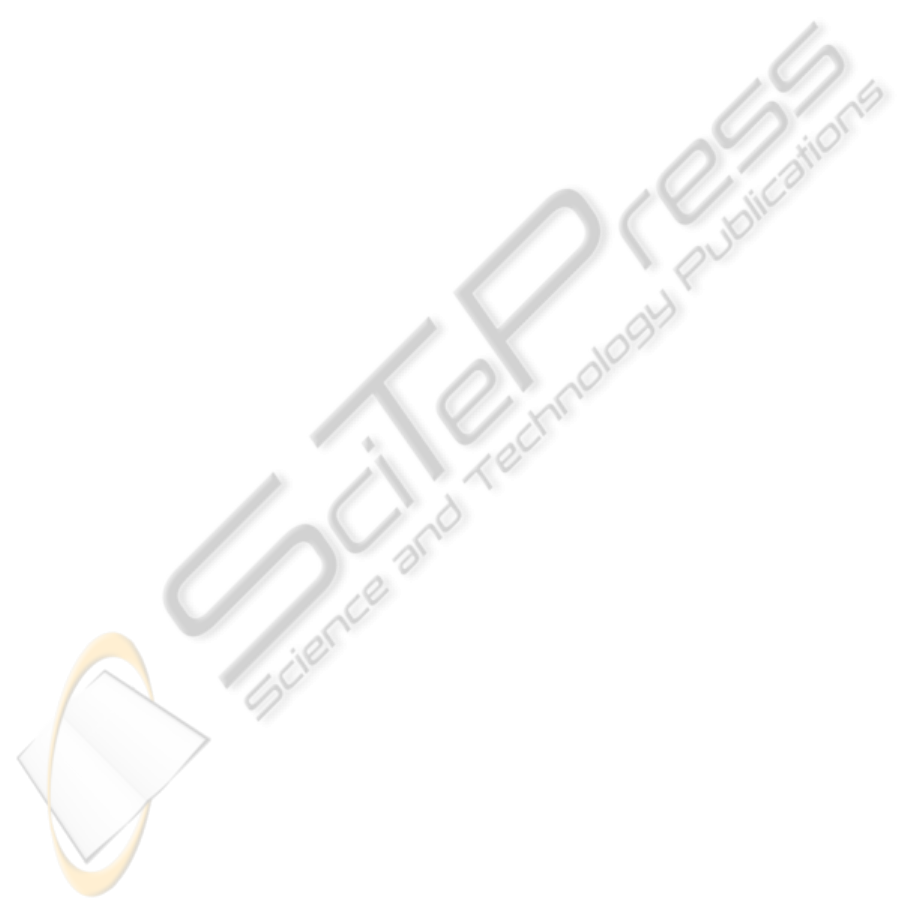
methods are unable to improve on the regression re-
sults of the regressor used as the basis for the study; a
weighted nearest neighbour regressor.
SNDA, one of the new methods proposed in the
article, has a performance comparable to WPCA for
low dimensions, and it shown to perform better at
higher dimensions. It is also worth noting that WPCA
performs better than LDAr, which contradicts the re-
sults of (Kwak and Lee, 2010), in which LDAr out-
performed WPCA.
Possible future work could determine whether the
conclusions obtained here might extend to cases in
which other regressors are used, as well as consid-
ering the effect of the parameters of the methods. An-
other interesting line of work would be to use these
methods as inductors of diversity in the algorithms
for building ensemble of regressors. This would be
motivated by the results obtained for Rotation For-
est using PCA (Rodríguez et al., 2006), or Nonlinear
Boosting Projection using NDA (García-Pedrajas and
García-Osorio, 2011). It is tempting to think that the
substitution of PCA and NDA in regression problems
for some of the proposed methods in this article could
improve the results.
REFERENCES
Demšar, J. (2006). Statistical comparisons of classifiers
over multiple data sets. The Journal of Machine
Learning Research, 7:1–30.
Fisher, R. et al. (1936). The use of multiple measurements
in taxonomic problems. Annals of eugenics, 7(2):179–
188.
Frank, A. and Asuncion, A. (2010). UCI machine learning
repository. Stable URL: http://archive.ics.uci.edu/ml/.
Fukunaga, K. and Mantock, J. (1983). Nonparametric
discriminant analysis. IEEE Transaction on Pattern
Analysis and Machine Intelligence, 6(5):671–678.
García-Pedrajas, N. and García-Osorio, C. (2011). Con-
structing ensembles of classifiers using supervised
projection methods based on misclassified instances.
Expert Systems with Applications, 38(1):343–359.
DOI: 10.1016/j.eswa.2010.06.072.
Guyon, I. and Elisseeff, A. (2003). An introduction to vari-
able and feature selection. Journal of Machine Learn-
ing Research, 3:1157–1182.
Jolliffe, I. (1986). Principal Component Analysis. Springer-
Verlag.
Kwak, N. and Lee, J.-W. (2010). Feature extraction based
on subspace methods for regression problems. Neuro-
computing, 73(10-12):1740–1751.
Lee, J. A. and Verleysen, M. (2007). Nonlinear Dimension-
ality Reduction. Springer.
Li, K.-C. (1991). Sliced inverse regression for dimension
reduction. Journal of the American Statistical Associ-
ation, 86(414):316–327.
Li, K.-C. (1992). On principal hessian directions for data
visualization and dimension reduction: Another appli-
cation of stein’s lemma. Journal of the American Sta-
tistical Association, 84(420):1025–1039. Stable URL:
http://www.jstor.org/stable/229064.
Li, K. C. (2000). High dimensional data analy-
sis via the SIR/PHD approach. Available at
http://www.stat.ucla.edu/∼kcli/sir-PHD.pdf.
Liu, H. and Yu, L. (2005). Toward integrating feature selec-
tion algorithms for classification and clustering. IEEE
Transanction on Knowledge and Data Engineering,
17:491–502.
Rodríguez, J. J., Kuncheva, L. I., and Alonso, C. J. (2006).
Rotation forest: A new classifier ensemble method.
IEEE Transactions on Pattern Analysis and Machine
Intelligence, 28(10):1619–1630.
Roweis, S. T. and Saul, L. K. (2000). Nonlinear Dimension-
ality Reduction by Locally Linear Embedding. Sci-
ence, 290(5500):2323–2326.
Tenenbaum, J. B., de Silva, V., and Langford, J. C. (2000).
A Global Geometric Framework for Nonlinear Di-
mensionality Reduction. Science, 290(5500):2319–
2323.
Tian, Q., Yu, J., and Huang, T. S. (2005). Boosting multiple
classifiers constructed by hybrid discriminant analy-
sis. In Oza, N. C., Polikar, R., Kittler, J., and Roli,
F., editors, Multiple Classifier Systems, volume 3541
of Lecture Notes in Computer Science, pages 42–52,
Seaside, CA, USA. Springer.
Wu, Q., Mukherjee, S., and Liang, F. (2008). Localized
sliced inverse regression. In Koller, D., Schuurmans,
D., Bengio, Y., and Bottou, L., editors, NIPS, pages
1785–1792. MIT Press.
ICPRAM 2012 - International Conference on Pattern Recognition Applications and Methods
204