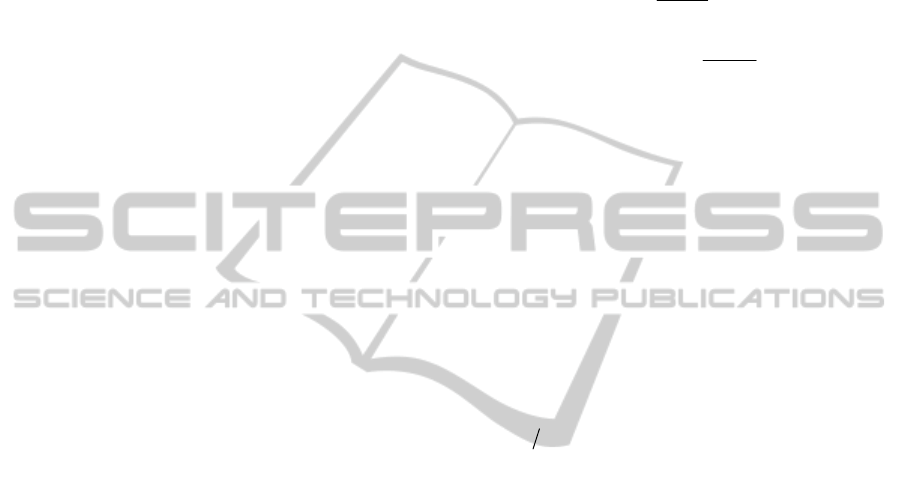
about epidemic mathematical models
(Mukhopadhyay and Bhattacharyya, 2007). Other
important aim is that relative to the design of control
strategies in order to eradicate the persistence of the
infection in the host population (De la Sen and
Alonso-Quesada, 2010); (De la Sen et al., 2011);
(Makinde, 2007); (Safi and Gumel, 2011). In this
context, an explicit vaccination function of many
different kinds may be considered, namely: constant,
continuous-time, impulsive, mixed
constant/impulsive, mixed continuous-
time/impulsive, discrete-time and so on.
In this paper, a SEIR epidemic model is
considered. The dynamics of susceptible (S) and
immune (R) populations are directly affected by a
vaccination function
V(t) , which also has indirectly
influence in the time evolution of infected or
exposed (E) and infectious (I) populations. In fact,
such a vaccination function has to be suitably
designed in order to eradicate the infection from the
population. This model has been already studied in
(De la Sen and Alonso-Quesada, 2010) from the
viewpoint of equilibrium points in the controlled and
free-vaccination cases. A vaccination auxiliary
control law being proportional to the susceptible
population was proposed in order to achieve the
whole population be asymptotically immune. Such
an approach assumed that the SEIR model was of
the aforementioned true-mass action type, its
parameters were known and the illness transmission
was not critical. Moreover, some important issues of
positivity, stability and tracking of the SEIR model
were discussed. The present paper proposes an
alternative method to obtain the vaccination control
law to asymptotically eradicate the epidemic
disease. Namely, the vaccination function is
synthesized by means of an input-output exact
feedback linearization technique. Such a
linearization control strategy constitutes the main
contribution of the paper. Moreover, mathematical
proofs about the epidemics eradication based on
such a controlled SEIR while maintaining the non-
negativity of all the partial populations for all time
are issued. The exact feedback linearization can be
implemented by using a proper nonlinear coordinate
transformation and a static-state feedback control.
The use of such a linearization strategy is motivated
by three main facts, namely: (i) it is a power tool for
controlling nonlinear systems which is based on
well-established technical principles (Isidori, 1995),
(ii) the given SEIR model is highly nonlinear and
(iii) such a control strategy has not been yet applied
in epidemic models.
2 SEIR EPIDEMIC MODEL
Let S(t) , E(t) , I(t) and R(t) be, respectively, the
susceptible, infected (or exposed), infectious and
removed-by-immunity populations at time
t .
Consider a time-invariant true-mass action type
SEIR epidemic model given by:
S(t)I(t)
S(t) S(t) R(t) N 1 V(t)
N
(1)
S(t)I(t)
E(t) ( )E(t)
N
(2)
I(t) ( )I(t) E(t)
(3)
R(t) ( )R(t) I(t) NV(t)
(4)
subject to initial conditions
S(0) 0 , E(0) 0 ,
I(0) 0 and R(0) 0 under a vaccination function
00
V:
, with
0
0,
. In the above
SEIR model,
0 is the total population at any
time instant
0
t
, 0
is the rate of deaths and
births from causes unrelated to the infection,
0
is the rate of losing immunity,
0 is the
transmission constant (with the total number of
infections per unity of time at time
t being
S(t)I(t) N
) and,
1
0
and
1
0
are,
respectively, the average durations of the latent and
infective periods. The total population dynamics can
be obtained by summing-up (1)-(4) yielding:
N(t) S(t) E(t) I(t) R(t) 0
(5)
so that the total population
(t)N(0)N
is
constant
0
t
. Then, this model is suitable for
epidemic diseases with very small mortality
incidence caused by infection and for populations
with equal birth and death rates so that the total
population may be considered constant for all time.
3 VACCINATION STRATEGY
An ideal control objective is that the removed-by-
immunity population asymptotically tracks the whole
population
. In this way, the joint infected plus
infectious population asymptotically tends to zero as
t , so the infection is eradicated from the
population. A vaccination control law based on a
static-state feedback linearization strategy is
developed for achieving such a control objective.
This technique requires a nonlinear coordinate
A VACCINATION CONTROL LAW BASED ON FEEDBACK LINEARIZATION TECHNIQUES FOR SEIR
EPIDEMIC MODELS
77