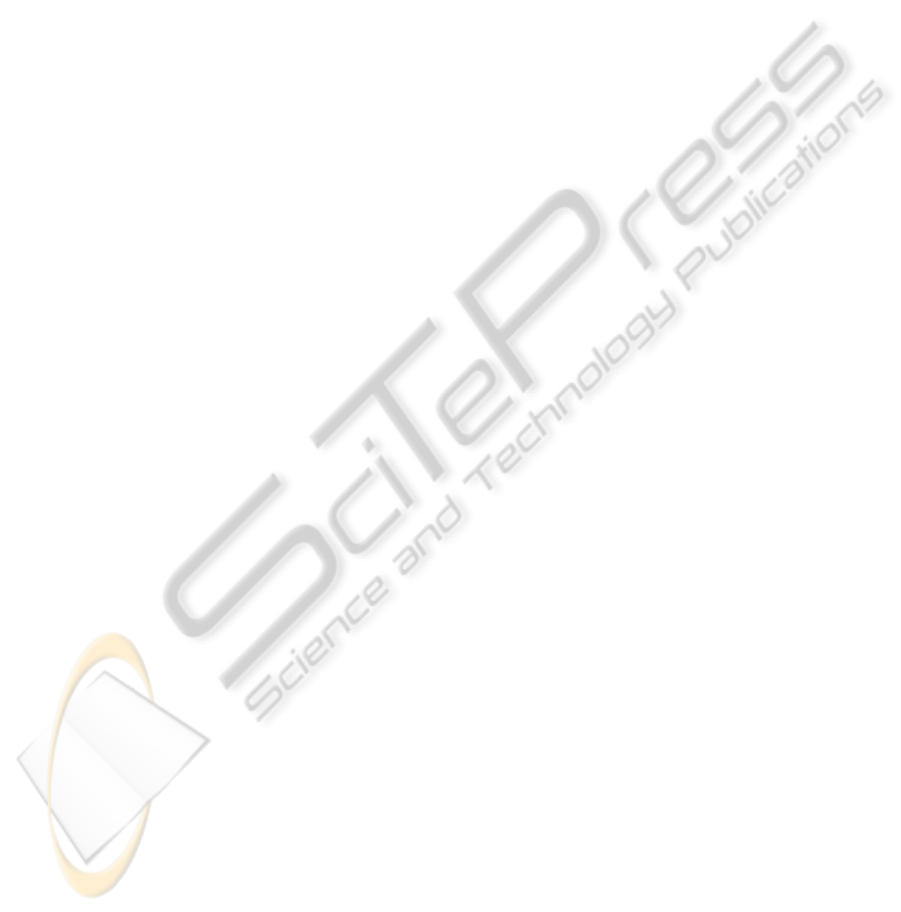
used, and the second value – when pure outputs of
SVC were used. In the SLP row the first value
means results where no parameter α was used in (3)
and the second value – the result with selected
optimal α. The best result in the PWFT column is
written in italic, while the best one within all the
methods for the data is marked in bold. The last
column in Table 2 shows the inaccuracy of the best
method.
Despite the differences between “the best” and
other methods in Table 2 being statistically
insignificant, it may be concluded that in some cases
the newly presented method may successfully
compete with others.
5 CONCLUSIONS AND
DISCUSSIONS
It was shown that due to the inclination of SLP to
obtain a set of classical statistical classifiers during
its training evolution, the PWFT+SLP classifier is an
effective method when the data classes are scattered
in such a way that the classifiers of diverse
complexities are needed for different pairs.
When using SVC as a pair-wise classifier, the
modification with sigmoid function (3) for outputs
has to be done. Experimental results showed that
both, the SVC and SLP based pair-wise classifiers
may be used as proper pair-wise classifiers in PWFT
fusion method. Therefore there still remains room
for investigation of PWFT method suitability
according to the number of classes, its
dimensionalities and other statistical characteristics.
A new parameter α was proposed for
PWFT+SLP error rate improvement. Preliminary
results showed that in situations unfavourable for the
PWFT+SLP method, the use of proper scaling
parameter α could allow it to perform at least with
the same error rate as voting methods. Employment
of some particular procedure of selecting optimal α
has to be investigated more deeply, because
improper α selection may make results worse, and
of course, selection of a proper value may
considerably improve overall classification results.
ACKNOWLEDGEMENTS
This paper is part of research financed by Research
Council of Lithuania (contract number MIP-
043/2011).
REFERENCES
Bishop, C. M., 1995. Neural Networks for Pattern
Recognition. Oxford Univ. Press.
Boser, B., Guyon, I., Vapnik, V., 1992. A Training
Algorithm for Optimal Margin Classifiers.
Proceedings of the Fith Annual Workshop on
Computational Learning Theory, pages 144-152.
ACM Press.
Chang, C.-C., Lin, C.-J., 2001. LIBSVM: a library for
support vector machines; http://www.csie.ntu.edu.tw/
~cjlin/libsvm.
Frank, A., Asuncion, A., 2010. UCI Machine Learning
Repository. Irvine, CA: University of California,
School of Information and Computer Science
http://www.ics.uci.edu/~mlearn/MLRepository.html.
Haghigi, M. S., Vahedian, A., Yazdi, H. S., 2011.
Extended Decision Template Presentation for
Combining Classifiers. Expert Systems with
Applications 38, pages. 8414-8418.
Hastie, T. and Tibshirani, R., 1998. Classification by
pairwise coupling. The Annals of Statistics, 26(1):
451-471.
Krzysko, M., Wolynski, W., 2009. New variants of
pairwise classification. European Journal of
Operational Research (EOR) 199(2):512-519.
Kuncheva, l., Bezdek, J. C., Sutton, M. A., 1998. On
Combining Multiple Classifiers by Fuzzy Templates,
Proceedings of the 1998 Annual Meeting of the North
American Fuzzy Information Processing Society,
193 – 197.
Platt J. C., Cristianini N., and Shawe-Taylor J., 2000.
Large margin DAG’s for multi-class classification.
Advances in Neural Information Processing Systems.
Cambridge, MA: MIT Press, vol. 12 pp. 547-553.
Raudys, S., 1998. Evolution and generalization of a single
neuron. I. SLP as seven statistical classifiers. Neural
Networks 11: 283−96.
Raudys, S., 2001. Statistical and Neural Classifiers: An
integrated approach to design. Springer-Verlag, NY.
Raudys., S, Kybartas R., Zavadskas, E. K., 2010.
Multicategory Nets of Single-layer perceptrons:
Complexity and Sample Size Issues, IEEE
Transactions on Neural Networks, vol. 2, no. 5,
784 – 795.
Skurichina, M., Raudys, S., Duin, R. P. W, 2000. K-NN
directed noise injection in multilayer perceptron
training, IEEE Trans. on Neural Networks, 11(2):
504−511.
FUZZY TEMPLATES FOR PAIR-WISE MULTI-CLASS CLASSIFICATION
567