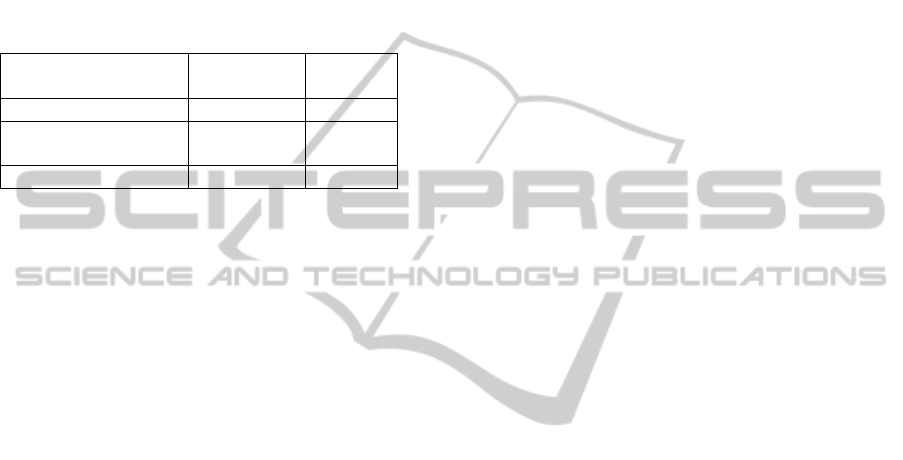
tive when compared with the literature. For Met-
enkephalin e 1CRN, MODE-P proved to be a good
optimizer considering the potential energy values. Its
values are smaller than those in the literature, asso-
ciate with good RMSD values. In particular case
of Met-enkephalin, for instance, potential energy and
RMSD
all−atoms
values obtained by MODE-P are bet-
ter than the comparison approach. For 1ZDD the val-
ues in terms of energy and RMSD were competitive
to others approaches.
Table 4: Results for 1ZDD protein.
Algorithm Energy (kcal
mol
−1
)
RMSD
C
α
(
˚
A)
MODE-P -1050.85 3.846
I-PAES (Cutello et al.,
2006)
-1052.09 2.27
GA (Dorn et al., 2011) -983.27 3.92
5 CONCLUSIONS AND FUTURE
WORKS
This paper has presented a multi-objective evolution-
ary algorithm for PSP problem with ab initio ap-
proach. The evaluation of the conformation of a pro-
tein is estimated using energy values of local and non-
local interactions in order to compose the potential
energy.
The results obtained suggest that MODE-P can
predict small proteins structures with competitive val-
ues compared with other works in literature. The in-
novative way for choosing the best individual in a
multi-objective differential evolution proved to be a
good option to be used during the evolutionary pro-
cess.
As future work we intend to expand MODE-P to
deal with medium size proteins and investigate alter-
native methods for decision maker.
ACKNOWLEDGEMENTS
The authors acknowledge the CNPq grant 307735/
2008-7 and Fundac¸˜ao Arauc´aria project 400/0910705
for the partial financial support.
REFERENCES
Becerra, D., Sandoval, A., Restrepo-Montoya, D., and
Ni˜no, L. F. (2010). A parallel multi-objective ab initio
approach for protein structure prediction. In Park, T.,
Tsui, S. K.-W., Chen, L., Ng, M. K., Wong, L., and Hu,
X., editors, BIBM, pages 137–141. IEEE Computer So-
ciety.
Coello, C. C., Lamont, G., and van Veldhuizen, D.
(2007). Evolutionary Algorithms for Solving Multi-
Objective Problems. Genetic and Evolutionary Compu-
tation. Springer, Berlin, Heidelberg, 2nd edition.
Cohen, F. E. and Kelly, J. W. (2003). Therapeutic
approaches to protein-misfolding diseases. Nature,
426:905–909.
Cutello, V., Narzisi, G., and Nicosia, G. (2006). A multi-
objective evolutionary approach to the protein structure
prediction problem. J R Soc Interface, 3(6):139–51.
Dandekar, T. and Argos, P. (1996). Identifying the tertiary
fold of small proteins with different topologies from se-
quence and secondary structure using the genetic algo-
rithm and extended criteria specific for strand regions.
Journal of Molecular Biology, 256(3):645–660.
Dorn, M., Buriol, L. S., and Lamb, L. C. (2011). A hybrid
genetic algorithm for the 3-d protein structure prediction
problem using a path-relinking strategy. In Evolution-
ary Computation (CEC), 2011 IEEE Congress on, pages
2709 –2716.
Pollastri, G., Przybylski, D., Rost, B., and Baldi, P. (2002).
Improving the prediction of protein secondary structure
in three and eight classes using recurrent neural networks
and profiles. Proteins: Structure, Function, and Bioin-
formatics, 47:228–235.
Storn, R. and Price, K. (1997). Differential evolution
– a simple and efficient heuristic for global optimiza-
tion over continuous spaces. J. of Global Optimization,
11(4):341–359.
Sun, Z. R., Rao, X. Q., Peng, L. W., and Xu, D. (1997). Pre-
diction of protein supersecondary structures based on the
artificial neural network method. Protein Engineering,
10(7).
Tramontano, A. (2006). Protein Structure Prediction: Con-
cepts and Applications. John Wiley and Sons.
Tudela, J. C. C. and Lopera, J. O. (2009). Parallel protein
structure prediction by multiobjective optimization. In
Proceedings of the 2009 17th Euromicro International
Conference on Parallel, Distributed and Network-based
Processing, pages 268–275, Washington, DC, USA.
IEEE Computer Society.
BIOINFORMATICS 2012 - International Conference on Bioinformatics Models, Methods and Algorithms
298