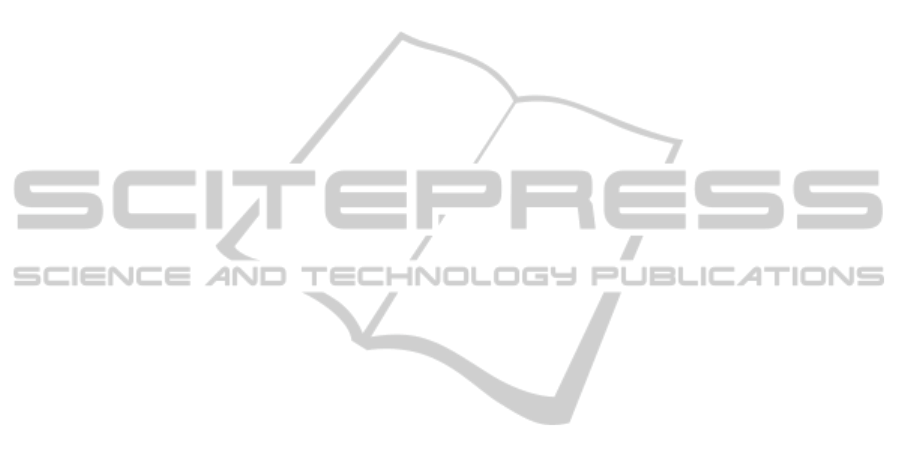
The signal-features are the first and second Auto-
Regressive coefficients, the Zero Crossing, the Num-
ber of Turns, the Wilson Amplitude and the frequency
histogram coefficients of the 225-500 Hz band (di-
vided in 5 segments).
We believe that the proposed methodology for
channel and feature selection makes a significant im-
provement with respect to the current ones. This
novel methodology may not only be appropriate for
the particular application presented in this paper (i.e.
channel and feature selection for hand gesture detec-
tion based on sEMG signals) but also in other case
scenarios, such as gene selection for classification of
phenotypes based on microarray data.
ACKNOWLEDGEMENTS
The authors would like to thank the department of Ed-
ucation of the Basque Government for their support.
REFERENCES
Bellman, R. (1961). Adaptive Control Processes: A Guided
Tour, volume 19 of A Rand Corporation Research
Study Series. Princeton University Press.
Boostani, R. and Moradi, M. H. (2003). Evaluation of the
forearm EMG signal features for the control of a pros-
thetic hand. Physiological measurement, 24(2).
Chang, C.-c. and Lin, C.-j. (2011). LIBSVM : a Library for
Support Vector Machines.
Cover, T. T. (1974). The Best Two Independent Measure-
ments are not the Two Best. Systems, Man and Cyber-
netics, IEEE Transactions, 1(JANUARY):116–117.
Ding, C. and Peng, H. (2005). Minimum redundancy fea-
ture selection from microarray gene expression data.
Journal of bioinformatics and computational biology.
Englehart, K., Hudgins, B., and Member, S. (2003). A Ro-
bust , Real-Time Control Scheme for Multifunction
Myoelectric Control. Engineering In Medicine And
Biology, 50(7):848–854.
Fuglsang-Frederiksen, A. and Pugdahl, K. (2010). Current
status on electrodiagnostic standards and guidelines
in neuromuscular disorders. Clinical neurophysiol-
ogy : official journal of the International Federation
of Clinical Neurophysiology, 122(3):440–455.
Hargrove, L. J., Englehart, K., and Hudgins, B. (2007).
A comparison of surface and intramuscular myoelec-
tric signal classification. IEEE transactions on bio-
medical engineering, 54(5):847–53.
Hudgins, B., Parker, P., and Scott, R. N. (1993). A new
strategy for multifunction myoelectric control. IEEE
transactions on bio-medical engineering, 40.
Hughes, G. (1968). On the mean accuracy of statistical pat-
tern recognizers. IEEE Transactions on Information
Theory, 14(1):55–63.
Jain, A. and Duin, R. (2000). Statistical pattern recognition:
a review. IEEE Transactions on Pattern Analysis and
Machine Intelligence, 22(1):4–37.
Kang, W. J., Shiu, J. R., Cheng, C. K., Lai, J. S., Tsao,
H. W., and Kuo, T. S. (1995). The application of cep-
stral coefficients and maximum likelihood method in
EMG pattern recognition. IEEE transactions on bio-
medical engineering, 42(8):777–85.
Khokhar, Z. O., Xiao, Z. G., and Menon, C. (2010). Sur-
face EMG pattern recognition for real-time control of
a wrist exoskeleton. Biomedical engineering online.
Kuiken, T. a., Miller, L. a., Lipschutz, R. D., Lock, B. a.,
Stubblefield, K., Marasco, P. D., Zhou, P., and Du-
manian, G. a. (2007). Targeted reinnervation for en-
hanced prosthetic arm function in a woman with a
proximal amputation: a case study. Lancet.
Merletti, R. and Parker, P. (2004). Electromyography: Phys-
iology, engineering, and noninvasive applications. En-
gineering In Medicine And Biology.
Palmes, P., Ang, W. T., Widjaja, F., Tan, L. C. S., and Au,
W. L. (2010). Pattern mining of multichannel sEMG
for tremor classification. IEEE transactions on bio-
medical engineering, 57(12):2795–805.
Peng, H., Long, F., and Ding, C. (2005). Feature selec-
tion based on mutual information: criteria of max-
dependency, max-relevance, and min-redundancy.
IEEE transactions on pattern analysis and machine
intelligence, 27(8):1226–38.
Philipson, L, Larsson, P, G. (1988). The electromyographic
signal as a measure of muscular force: a compari-
son of detection and quantification techniques. Elec-
tromyography and clinical neurophysiology.
Tenore, F., Ramos, A., Fahmy, A., Acharya, S., Etienne-
Cummings, R., and Thakor, N. V. (2007). Towards the
control of individual fingers of a prosthetic hand using
surface EMG signals. Annual International Confer-
ence of the IEEE Engineering in Medicine and Biol-
ogy Society.
Tenore, F. V. G., Ramos, A., Fahmy, A., Acharya, S.,
Etienne-Cummings, R., and Thakor, N. V. (2009). De-
coding of individuated finger movements using sur-
face electromyography. IEEE transactions on bio-
medical engineering, 56(5):1427–34.
Tkach, D., Huang, H., and Kuiken, T. a. (2010). Study
of stability of time-domain features for electromyo-
graphic pattern recognition. Journal of neuroengi-
neering and rehabilitation, 7:21.
Zardoshti-Kermani, M., Wheeler, B., Badie, K., and
Hashemi, R. (1995). EMG feature evaluation for
movement control of upper extremity prostheses.
IEEE Transactions on Rehabilitation Engineering.
BIOSIGNALS 2012 - International Conference on Bio-inspired Systems and Signal Processing
48