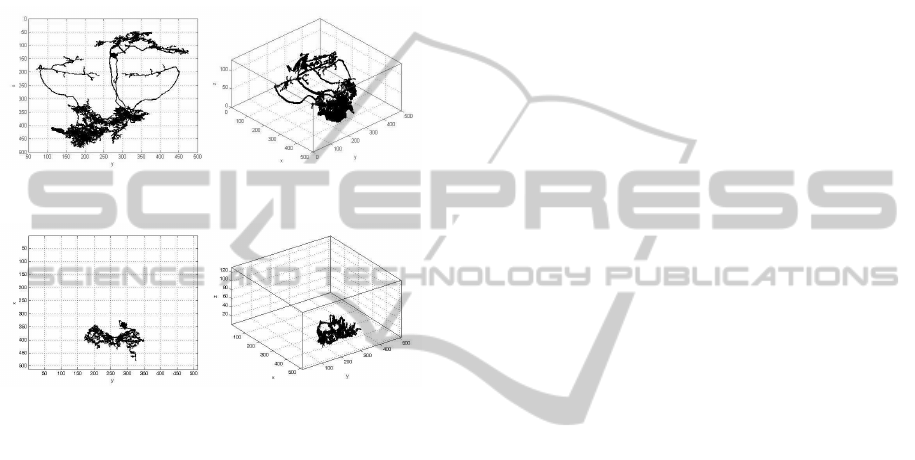
cessfully. Fig.7 represents that even if the neurons,
which are spatially close and randomly assigned to
similar colors, cannot be clearly separated, the pro-
posed algorithm can at least provide a reference to as-
sist biologists identifying the neural circuits in a cell-
to-cell level. In short, the experimental results show
that the proposed scheme can segment the Flybow im-
agery well, even though there are still some improve-
ments needed to be carried out.
(a) (b)
(c) (d)
Figure 7: Neurons that cannot be separated from each other.
Though terminals of the neuron fibers of at least 2 neuron
cells are interlaced, the segmentation result can also provide
a reference to biologists for identifying different neurons.
5 CONCLUSIONS
We proposed a prototype scheme based on grayscale
morphological operations for segmenting Fly-
bow/Brainbow imagery. It is time-consuming to label
the neural circuits from Flybow/Brainbowimagery by
hand and also difficult to trace them by using existing
algorithms designed for tracing a single neuron. The
proposed method can provide segmentation results
semi-automatically, and consequently it would be
useful for biologists to identify the neuro-circuits.
Besides, in order to develop a sound and robust
algorithm for this kind of data, it is inevitable to es-
tablish a ground truth first. Thus, our segmentation
results need to be verified by biologists repeatedly un-
till a well-accepted ground truth is constructed. We
will start this task by first segmenting some neurons
well-known in biological literatures and then extend
the algorithm to other neurons. Moreover, there is at
least one another reachable future improvement for
this prototype scheme. That is, design a distance met-
ric which can integrate color information into exist-
ing tracing algorithms or clustering methods so that
it is able to seperate neighboring neurons assigned
to similar colors. By completing the possible im-
provements, we are looking forward to establishing a
more robust segmentation/tracing scheme for Brain-
bow/Flybow imagery in the future.
ACKNOWLEDGEMENTS
This research work is supported by Academia Sinica,
Taiwan. The authors also want to thank Prof. Ann-
Shyn Chiang, the Program Director of the Brain Re-
search Center at National Tsing Hua University, and
his team for providing the experiment image sets and
their enthusiastic support.
REFERENCES
Bas, E. and Erdogmus, D. (2010). Piecewise linear cylinder
models for 3-dimensional axon segmentation in brain-
bow imagery. In Proceedings of ISBI, 1297–1300.
Bier, E. (2005). Drosophila, the golden bug, emerges as a
tool for human genetics. Nature Rev. Genet., 6:9–23.
Gonzalez, R. C. and Woods, R. E. (2007). Digital image
processing. Prentice Hall, 3rd edition.
Hadjieconomou, D., Rotkopf, S., Alexandre, C., Bell, D.,
Dickson, B., and Salecker, I. (2011). Flybow: genetic
multicolor cell labeling for neural circuit analysis in
drosophila melanogaster. Nature Methods, 8:260–
266.
Lee, P. C., Chang, H. M., Lin, C. Y., Chiang, A. S., and
Ching, Y. T. (2009). Constructing neuronal structure
from 3D confocal microscopic images. Journal of
Medical and Biological Engineering, 29:1–6.
Livet, J., Weissman, T. A., Kang, H., Draft, R. W., Lu,
J., Bennis, R. A., Sanes, J. R. , and Lichtman, J. W.
(2007). Transgenic strategies for combinatorial ex-
pression of fluorescent proteins in the nervous system.
Nature, 450:56–62.
Mathwork. http://www.mathworks.com/help/toolbox/
images/f18-16264.html
Peng, H., Long, F., and Myers, G. (2011). Automatic 3D
neuron tracing using all-path pruning. In Proceedings
of Bioinformatics [ISMB/ECCB], 239–247.
Rodriguez, A., Ehlenberger, D., Hof, P., and Wearne,
S. (2011). Three-dimensional neuron tracing by
voxel scooping. Journal of Neuroscience Methods,
184:169–175.
FLYBOW IMAGE SEGMENTATION - For Tracing Neuron Circuits in Drosophila Brain
369