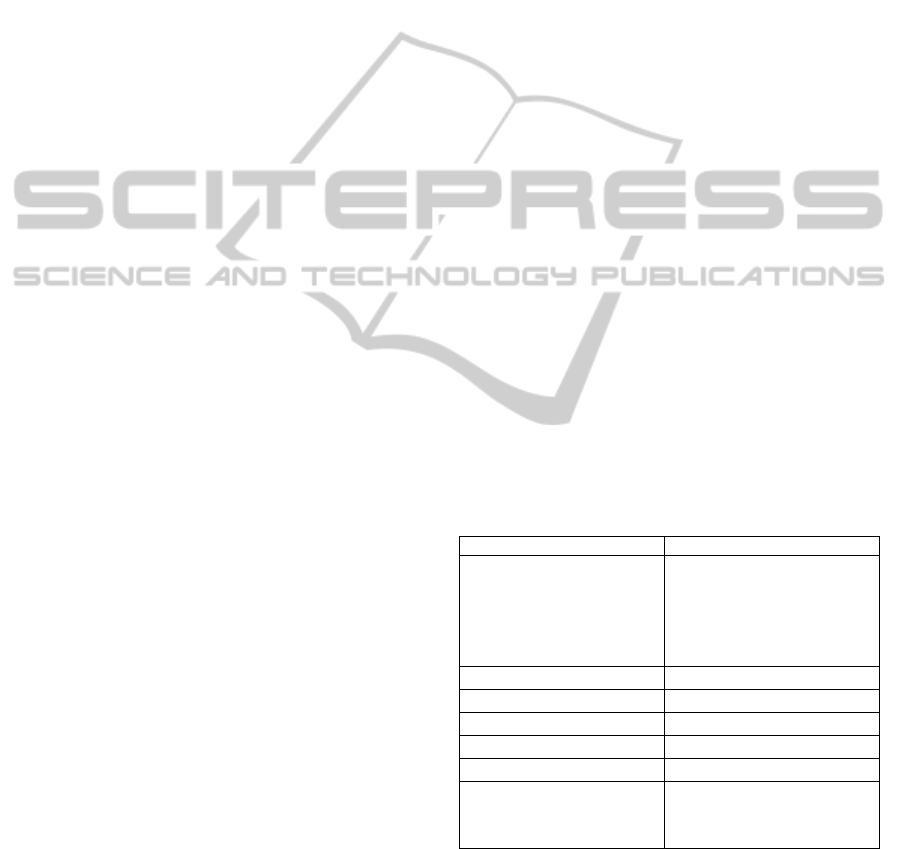
Hence, the present research was designed to
study healthy subjects engaged into two reasoning
tasks, valid syllogisms versus paradoxes, adjusted to
induce working memory (WM).
Contemporary neuropsychological views define
WM as the capacity of the human subject to keep
information ‘on-line’ necessary for an ongoing task
(Baddeley, 1998); (Collette and Van der Linden,
2002). Accordingly, WM is not for ‘memorizing’
per se; it is rather in the service of complex
cognitive activities, such as reasoning, monitoring,
problem solving, decision making, planning and
searching/shifting the initiation or inhibition
response (Miyake and Shah, 1999); (Glassman,
2000). Thus, WM incorporates, among others, a
central executive system. Therefore, the present
study, dealing with a sample of healthy adults, aims
at determining if different patterns of electro-
physiological activity exist, as reflected by event
related potentials (ERPs). Each experimental
condition and setting is adjusted, so as to induce
working memory operation.
Event-related potential (ERP) techniques are
known to be useful tools in the investigation of
information processing and seem to be sensitive to
subtle neuropsychological changes (Kotchoubey,
2006); (Kotchoubey et al., 2002); (Papageorgiou and
Rabavilas, 2003); (Papageorgiou et al. 2004);
(Beratis et al. 2009). The main goal of the present
work is to provide direct evidence of association
and/or dissociation of Aristotelian syllogistic
reasoning and reasoning induced during the
exposition to paradoxes. A comparative study of
these activation patterns in Aristotelian and paradox-
related reasoning could reveal critical aspects of
reasoning processing, associated with perception,
attention and cognitive behaviour. We note that
these aspects are unobservable with behavioural
methods alone.
2 METHODS
2.1 Participants
This study was approved by the Ethics committee of
University Mental Health Research Institute
(UMHRI). Thirty-one healthy subjects (aged 33.6
years on average, standard deviation: 9.1; 17 males)
participated in the experiment. All participants gave
written consent, after being extensively informed
about the procedure. They all had normal vision and
no one had neurological or psychiatric history.
2.2 Behavioural Procedures and the
Four Different Classes of Questions
The participants were seated comfortably 1m away
from a computer monitor in an electromagnetically
shielded room. First, proper instructions were given
to the participants together with a training test. The
participants entered the formal experimental session,
once they had fully comprehended the experimental
task. The experiment was designed to validate two
mental functions, one associated with “valid”
syllogisms and another with “paradox reasoning”.
Two indicative examples follow:
A) Concerning the class “valid”, the following
statements were shown to each participant: “All men
are animals. All animals are mortal. Hence, all men
are mortal.”
B) Concerning the class “paradox”, the following
statements were shown to each participant: “A
moving arrow occupies a certain space at each
instant. But, when an object occupies a specific
space, it is motionless. Therefore, the arrow cannot
simultaneously move and be motionless.” (The
revised Oxford Translation of Aristotle, 1995).
Every such sequence of statements, forming a
reasoning, appeared on the computer monitor
accompanied by the question “true or false”. The
duration of the presented sentence was directly
proportional to the letters involved in each sentence
as described in Table 1.
Table 1: Units for magnetic properties.
Sequence of actions Duration of actions
Valid or paradox sentence
(visual presentation)
Duration according to the
numbers of the letters in the
sentences e.g. a sentence
involving 92 letters presented
11,04sec
EEG recording 1000ms
Warning stimulus 100ms
ERP recording 1sec
Warning stimulus repetition 100ms
Response onset Within 5sec
Period between response
completion and onset of
next sentence presentation
4-9sec
Then, the monitor screen went blank for
1000ms. Next, a sound warning stimulus of 65dB,
500Hz and 100ms duration was given, followed by
the same warning stimulus after 900ms. Participants,
after the second warning stimulus, were asked to
judge each reasoning as either correct or incorrect.
In addition, his/hers estimated degree of confidence
CLASSIFYING EVENT RELATED POTENTIALS FOR VALID AND PARADOX REASONING
219