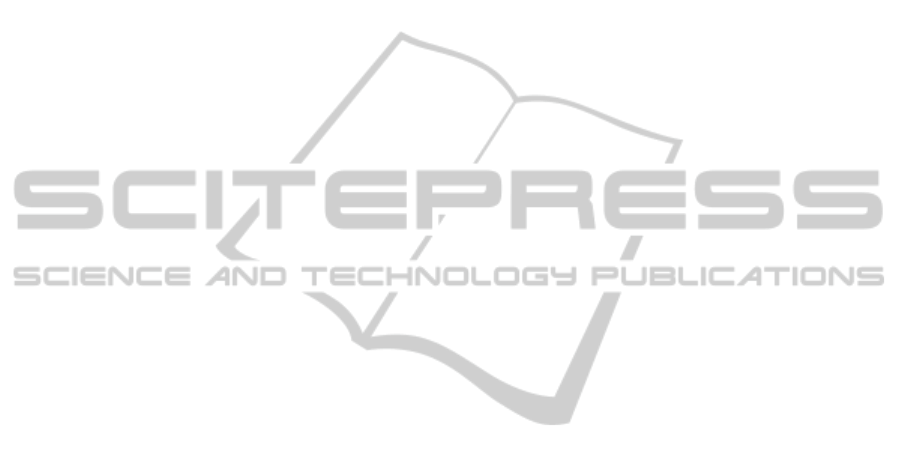
group of genes rather than metaGenes.
4 CONCLUSIONS
By systematically filtering complex microarray
datasets, we identified the minimal gene sets able to
discriminate disease states. This is important as any
diagnostic test needs to be cost effective, and testing
small numbers of genes in disease biopsies is much
more cost-effective compared to performing, for
example, genome-wide analyses. While PCA may
be useful in reducing array dimensionality, methods
that isolate identifiable genes are preferred.
Moreover, the identity of critical genes yields insight
into mechanisms of disease pathogenesis. A further
increase in accuracy may be provided by the
inclusion of currently unannotated transcripts, or by
increasing pathway definitions, but at the present
time this is algorithmically complex. Ultimately,
diagnostic gene expression fingerprints must be
rigorously evaluated in prospective analyses, and we
are currently refining our methods to facilitate
discrimination of ever more complex disease types.
REFERENCES
Ashburner, M., Ball, C. A., Blake, J. A., Botstein, D.,
Butler, H., Cherry, J. M., Davis, A. P., Dolinski, K.,
Dwight, S. S., Eppig, J. T. and others. (2000). Gene
Ontology: tool for the unification of biology. Nature
genetics, 25, 25-29.
Asyali, M. H., Colak, D., Demirkaya, O. and Inan, M. S.
(2006). Gene Expression Profile Classification: A
Review. Current Bioinformatics, 1, 55-73.
Bonome, T., Levine, D. A., Shih, J., Randonovich, M.,
Pise-Masison, C. A., Bogomolniy, F., Ozbun, L.,
Brady, J., Barrett, J. C., Boyd, J. and others. (2008). A
gene signature predicting for survival in suboptimally
debulked patients with ovarian cancer. Cancer
Research, 68, 5478.
Cheadle, C., Vawter, M. P., Freed, W. J. and Becker, K.
G. (2003). Analysis of Microarray Data Using Z Score
Transformation. Journal Of Molecular Diagnostics, 5,
73-81.
Chen, X. and Wang, L. (2009). Integrating Biological
Knowledge with Gene Expression Profiles for
Survival Prediction of Cancer. Journal of
Computational Biology, 16, 265–278.
Chuang, H. Y., Lee, E., Liu, Y. T., Lee, D. and Ideker, T.
(2007). Network-based classification of breast cancer
metastasis. Molecular systems biology, 3, 140.
Curtis, R. K., Oresic, M. and Vidal-Puig, A. (2005).
Pathways to the analysis of microarray data. TRENDS
In Biotechnology, 23, 429-435.
Dahlquist, K. D., Salomonis, N., Vranizan, K., Lawlor, S.
C. and Conklin, B. R. (2002). GenMAPP, a new tool
for viewing and analyzing microarray data on
biological pathways. Nature Genetics, 31, 19-93.
Gene Expression Omnibus database. (n.d.). Retrieved June
2011, from http://www.ncbi.nlm.nih.gov/geo/
GeneSifter® Analysis Edition. (n.d.). Retrieved January
2011, from http://www.genesifter.net
Guo, Z., Zhang, T., Li, X., Wang, Q., Xu, J., Yu, H., Zhu,
J., Wang, H., Wang, C., Topol, E., Wang, Q. and Rao,
S. (2005). Towards precise classification of cancers
based on robust gene functional expression profiles.
BMC Bioinformatics, 6, 58.
Hwang, T. and Park, T. (2009). Identification of
differentially expressed subnetworks based on
multivariate ANOVA. BMC bioinformatics, 10, 128.
Ibrahim, M. A. H., Jassim, S., Cawthorne, M. A. and
Langlands, K. (2011a). A Topology-based Score for
Pathway Enrichment. In Press, Journal of
Computational Biology .
Ibrahim, M. A. H., Jassim, S., Cawthorne, M. A. and
Langlands, K. (2011b). Pathway-based Gene Selection
for Disease Classification. International Conference
on Information Society (pp. 360-365). London: IEEE.
Jain, A. and Zongker, D. (1997). Feature Selection:
Evaluation, Application, and Small Sample
Performance. IEEE Transactions On Pattern Analysis
And Machine Intelligence PAMI, 19, 153-157.
Jain, A. K., Duin, R. P. and Mao, J. (2000). Statistical
Pattern Recognition: A Review. IEEE Transactions
On Pattern Analysis And Machine Intelligence PAMI,
22, 4-37.
Kanehisa, M. and Goto, S. (2000). KEGG: Kyoto
encyclopedia of genes and genomes. Nucleic Acids
Research, 28, 27.
Kim, J. M., Sohn, H. Y., Yoon, S. Y., Oh, J. H., Yang, J.
O., Kim, J. H., Song, K. S., Rho, S. M., Yoo, H. S.,
Kim, Y. S. and others. (2005). Identification of Gastric
Cancer–Related Genes Using a cDNA Microarray
Containing Novel Expressed Sequence Tags
Expressed in Gastric Cancer Cells. Clinical Cancer
Research, 5, 473.
Kim, S. J., Nakayama, S., Miyoshi, Y., Taguchi, T.,
Tamaki, Y., Matsushima, T., Torikoshi, Y., Tanaka,
S., Yoshida, T., Ishihara, H. and others. (2007).
Determination of the specific activity of CDK1 and
CDK2 as a novel prognostic indicator for early breast
cancer. Annals of Oncology, 48, 68.
Lawrence, J. A., Merino, M. J., Simpson, J. F., Manrow,
R. E., Page, D. L. and Steeg, P. S. (1998). A high-risk
lesion for invasive breast cancer, ductal carcinoma in
situ, exhibits frequent overexpression of retinoid X
receptor. Cancer Epidemiology Biomarkers &
Prevention, 7, 29.
Lo, Y. L., Yu, J. C., Chen, S. T., Hsu, G. C., Mau, Y. C.,
Yang, S. L., Wu, P. E. and Shen, C. Y. (2007). Breast
cancer risk associated with genotypic polymorphism
of the mitotic checkpoint genes: a multigenic study on
cancer susceptibility. Carcinogenesi , 28, 1079.
Nacu, S., Critchley-Thorne, R., Lee, P. and Holmes, S.
BIOINFORMATICS 2012 - International Conference on Bioinformatics Models, Methods and Algorithms
162