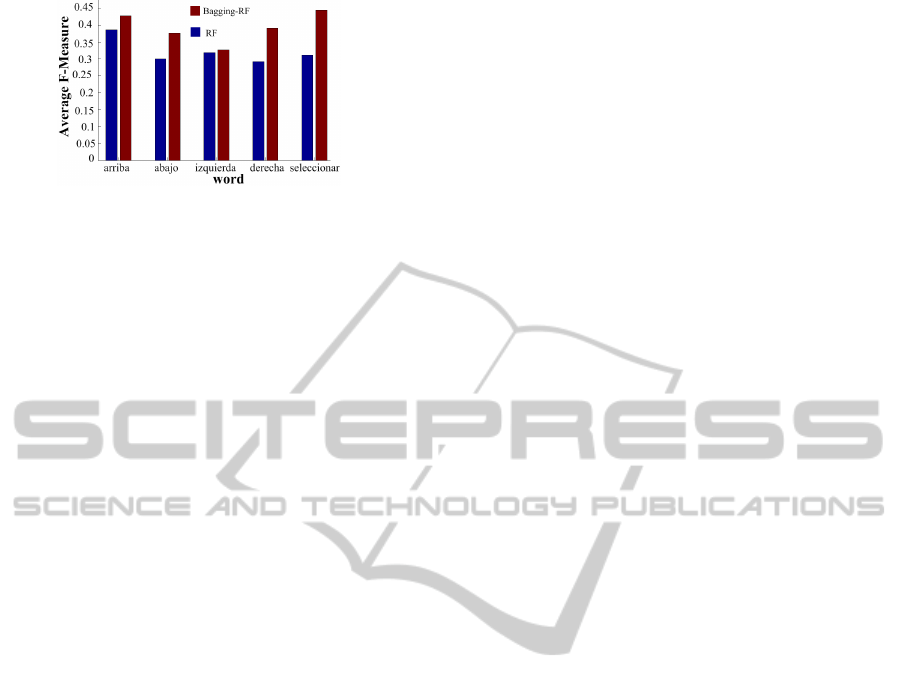
Figure 3: Graph of average f-measure for each word ob-
tained for RF and Bagging RF using 10-folds cross valida-
tion on the subject’s data.
It is important to note that, the words “selec-
cionar” and “arriba” classified by Bagging-RF have a
F-measure above 0.4, which is twice bigger than ran-
dom.
Last, it is worth to mention that in the presented
work the results obtained are relatively comparable to
state of the art similar works, like those reported in
(Porbadnigk, 2008) where the classification was eval-
uated only based in accuracy, reporting 45.95% for
five words. This comparison is mentioned consider-
ing the differences described in section 1.
4 CONCLUSIONS AND FUTURE
WORK
The acoustic speech signal and the EEG signals have
different features, which makes them naturally dis-
similar. In consequence, we explored an alternative
processing and classification approach to treat the
EEG signals, in particular those related to unspoken
speech. Indeed, the problem of interpreting unspo-
ken speech is still far to be solved. However, from
our experiments we obtained evidence to affirm that
the EEG signals actually carry useful information to
allow the classification of unspoken words. We con-
clude this based on the percentages of accuracy in
the classification for the four classifiers, which, are
above chance for five classes (see figure 2). Our re-
sults and experimental procedures are consistent with
those reported in the state of the art, because: we per-
formed experiments with more than one classifier, we
explored a language different to English, we used a
reduced vocabulary with more semantic meaning, and
we worked with features obtained by a feature selec-
tion approach instead a dimensionality reduction ap-
proach. The average f-measure was below the per-
centages due to chance for five classes.
To improve the reported results we propose to ex-
plore how to utilize and compare all windows regard-
less their size. We propose to apply independent com-
ponent analysis (ICA) and assess each independent
component using the Hurst’s coefficient to eliminate
some artifacts as blinks and heartbeats. To select an-
other wavelet family also could help. We also plan to
test another EEG signal representation and combine
them with the DWT coefficients. Finally, it is still
possible to use hybrid intelligent systems, and others
ensemble schemes to improve classification results.
ACKNOWLEDGEMENTS
This work was done under partial support of CONA-
CyT (scholarship # 234705), and INAOE.
REFERENCES
Brigham, K. and Kumar, B. (2010). Imagined Speech
Classification with EEG Signals for Silent Commu-
nication: A Preliminary Investigation into Synthetic
Telepathy. In Bioinformatics and Biomedical Engi-
neering (iCBBE), 2010 4th International Conference
on, pages 1–4. IEEE.
DaSalla, C. S., Kambara, H., Koike, Y., and Sato, M.
(2009). Spatial filtering and single-trial classification
of EEG during vowel speech imagery. In i-CREATe
’09: Proceedings of the 3rd International Convention
on Rehabilitation Engineering & Assistive Technol-
ogy, pages 1–4, New York, NY, USA. ACM.
Denby, B., Schultz, T., Honda, K., Hueber, T., Gilbert, J.,
and Brumberg, J. (2010). Silent speech interfaces.
Speech Communication, 52(4):270–287.
Dietterich, T. (2000). Ensemble methods in machine learn-
ing. Multiple classifier systems, pages 1–15.
D’Zmura, M., Deng, S., Lappas, T., Thorpe, S., and Srini-
vasan, R. (2009). Toward EEG sensing of imagined
speech. Human-Computer Interaction. New Trends,
pages 40–48.
Geschwind, N. (1972). Language and the brain. Scientific
American.
Lotte, F., Congedo, M., Lécuyer, A., Lamarche, F., and Ar-
nald, B. (2007). A review of classication algorithms
for EEG-based brain-computer interfaces. Journal of
Neural Engineering, 4:r1–r13.
Porbadnigk, A. (2008). EEG-based Speech Recognition:
Impact of Experimental Design on Performance. Mas-
ter’s thesis, Institut für Theoretische Informatik Uni-
versität Karlsruhe (TH), Karlsruhe, Germany.
Suppes, P., Lu, Z., and Han, B. (1997). Brain wave
recognition of words. Proceedings of the National
Academy of Sciences of the United States of America,
94(26):14965.
Wester, M. (2006). Unspoken Speech - Speech Recognition
Based On Electroencephalography. Master’s thesis,
Institut für Theoretische Informatik Universität Karl-
sruhe (TH), Karlsruhe, Germany.
TOWARD A SILENT SPEECH INTERFACE BASED ON UNSPOKEN SPEECH
373