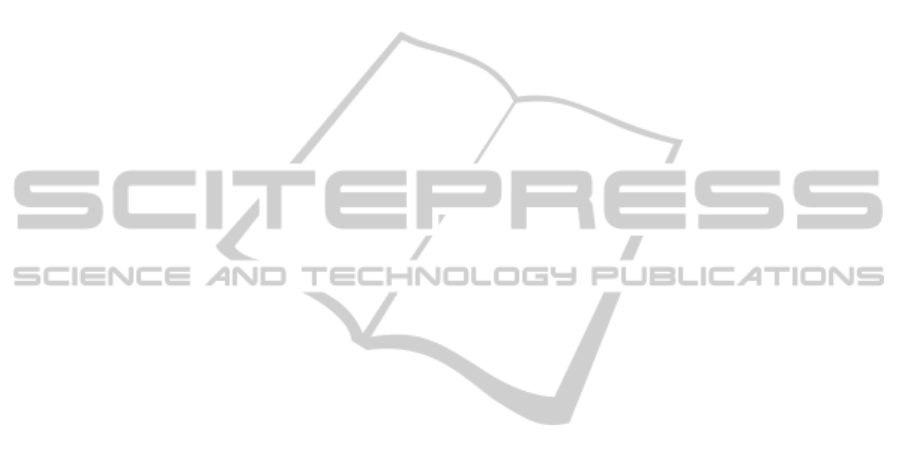
with NN that eventuated to recommendation of F7,
C3, P3 and O1 channels for identification analysis.
Touyama and Hirose (2008) by assaying Cz, Pz,
CPz which contain P300 occurrence that outcome
from retrieval of image in single-trial mode and 5,
10 and 20 times averaging with LDA as a classifier,
respectively . The performed method in Touyama
investigation has some advantages, such as easy
operation for retrieval protocols on different persons
and using only 3 electrodes which can be useful for
reducing the stress of subject.
Biometry system in verification mode evaluates
the accuracy of identity detection by verifying
claimed individual with comparing the individual
with his/her own template(s) , whiles biometry in
identification mode processes and compares the
claimed person with the whole recorded data set
(Woodward, Nicholas, Orlans and Higgins, 2003).
In the following investigation, the performance of
biometry system has been improved moreover the
conceivability of using verification mode by
evaluating EEG signal based on P300 occurrence.
The SVM (Support Vector Machine) and MLP
(multilayer perceptron) NN have been used for
identification and verification. In verification mode
the signals were only processed in single-trial way.
In addition of single-trial, the 5, 10 and 20 times
averaging were analysed in identification mode,
which had a significant improvement in single-trial
in comparison with the pervious study (Touyama &
Hirose, 2008) and also in maximum state, 100%
accuracy has obtained with only 5 times averaging.
This article is organized as follows. In Section 2
the way EEG signals were recorded has been
presented. Afterwards the process will be explained
including a brief description about the PCA
algorithm, which data dimensions were reduced by
it, utilized NN and proposed SVM. Results and
discussion are discussed specifically in the third and
fourth sections.
2 METHODES AND MATERIALS
2.1 Dataset
The EEG’s data in fourteenth reference has been
used in this study. These datasets have been
recorded by Touyama’s team in Tokyo University.
The EEG was recorded according to the extended
10/10 system. Only Cz, Pz, CPz channels have been
used for processing the datasets. EEG analog signals
have been recorded by a multi-channel bio-signal
amplifier named MEG-6116, which its band-width
was regulated from 0.5 and 30 Hz with a band pass
filter. Then data sets were sampled with 128 Hz
frequency by using a standard A/D converter and the
digitized EEG data was stored in a personal
computer. Five healthy subjects with normal vision
abilities were considered. All subjects were male
with 23, 25, 36, 24 and 21 years old, respectively.
During recording, each of the subjects was placed in
front of a monitor on a comfy chair, and with about
11.4 degrees of visual angle. 9 different images have
been shown to each person randomly, that these
pictures were shown to him and he selected one or
more images (oddball-task). Time of displaying for
each photo was 0.5 seconds and before showing the
next photo, 2 seconds had been given for eye-
fixation. Thus each period of this experiment took
6.5 seconds. In each session for each person, this
experiment was repeated 20 times. Then, EEG signal
was recorded in each session in total for each
subject, during 130 seconds (20 × 6.5 = 130). The
process had been repeated for each subject in 5
sessions
2.2 Processing
The only EEG data sets, which are referred to target
picture retrieval and have P300 characteristic, have
been processed. The first and fifth subject had been
chosen 3 pictures, the second and the fourth, 1
picture and the third had been selected 2 pictures,
among 9 shown pictures. Hence the number of
EEG’s datasets on single-trial mode, are 300, 100,
200, 100, 300 samples, alternatively. Therefore the
entire dataset comprises 1000 samples. One of the
potential’s indicator methods depends on time
domain averaging. In this state 5, 10, 20 times
averaging among related EEG signals of each person
were performed due to P300 occurrence employing
as a unique feature. Toward this, the entire data sets
were divided in to N parts, which N equals 5, 10 and
20, alternatively for 5, 10 and 20 times averaging.
Therefore the dataset contains 50, 100 and 200
samples per 5, 10 and 20 times averaging,
respectively. According to three channels, recording
time and sampling frequency, for apiece signal, 192
dimensions (3*50*128=192) in time domain
attained, which were chosen as a feature vector.
Confirmation or ignoring the identity of a person
is the target of biometry in verification mode that
reduces the data set’s volume and consequently
speeds up the process. Therefore 10 percent of each
person’s signal was allocated as a main part of
dataset in verification mode, and some dissimilar
brain signals contain P300 were added to
BIOMETRY BASED ON EEG SIGNALS USING NEURAL NETWORK AND SUPPORT VECTOR MACHINE
375