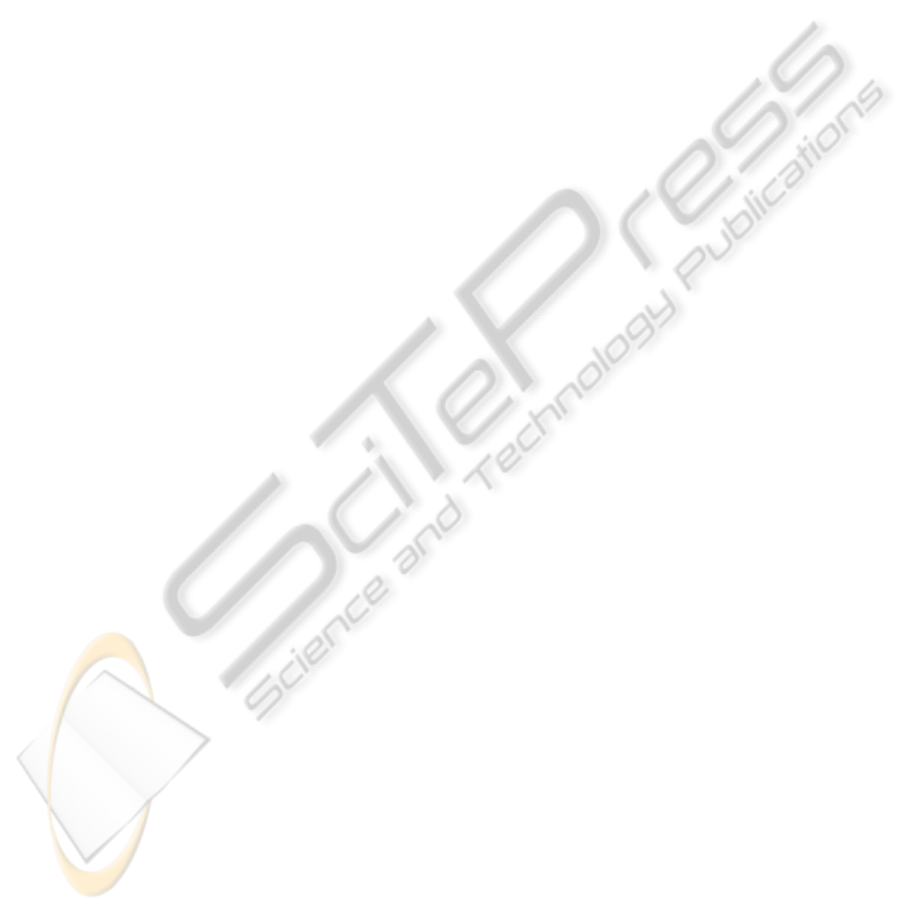
Vince’s performance of the “come” prototype lacks
the orientation of his hand palm. Even though the
plain hand wrist trajectory matches the subject’s hand
wrist trajectory, the incorrectly oriented palm might
make it difficult for a human user to identify the in-
tended gesture. Presumably, a more detailed body
model, as e.g. in (Bergmann and Kopp, 2009), would
improve the prototype representation. In contrast,
other gesture classes are sufficiently represented only
by hand wrist trajectories, e.g., Vinces performances
of all waving related classes are easy to comprehend.
Figure 4 illustrates the dependency between the
hyperparameters (K, σ) and the leave-one-out ac-
curacy in terms of AUC rates. This figure clearly
demonstrate that the accuracy remains stable and is
almost independent of the number of states K and the
value of emission distribution parameter σ. Only for
values of σ < 0.2 or K = 10 the AUC accuracy sub-
stantially decreases.
Figure 5(a) shows the performance results of our
evaluation in terms of classification accuracy on the
test set depending on the number of training exam-
ples per class for different classifiers. These in-
clude OMMs with a high number of states according
to maximum classification accuracy (K = 210) and
OMMs with a reduced number of states (K = 10, 50).
In general, all classifiers are able to recognize un-
seen gesture trajectories with high accuracy, although
OMM classifier with 10 model states reach substan-
tially lower accuracy. Using all training examples,
NN
DTW
as well OMMs classify gestures with high ac-
curacy of ≈ 0.94, although the performance for OMM
classifiers with only K = 10 is noticeably lower. The
plot also shows that a reduction of the number of
training examples does not substantially reduce the
classification accuracy. Only for OMMs with a low
number of states, a degradation can be observed be-
low three examples.
The slightly higher recognition performance of
NN
DTW
classifiers comes at the cost of substantially
increased computational demands. In the scenario
with all available training data, OMMs classifiers pro-
vide an average speed-up factor of at least ≈ 3. For
decreasing number of models states the speed-up fac-
tor increases once more. OMM classifier with K = 50
respond in ≈ 0.14 seconds, with K = 10 OMM clas-
sify an unseen gesture in 0.04 seconds. In comparison
to the average classification times of NN
DTW
this is an
acceleration between 3 and 44 times. This allows low-
latency recognition of gesture performances which is
a requirement for interaction with humans.
6 CONCLUSIONS
We applied ordered means models (OMMs) to recog-
nize and reproduce natural gesture trajectories. The
results from our classification experiment show that
OMMs are able to learn gestures from multivariate
times series even if only few observations are avail-
able. Furthermore, our run time measurements indi-
cate, that OMMs are well suited for low latency ges-
ture recognition. Even though more complex mod-
els and methods might further increase the recogni-
tion performance, in particular in human computer
interaction scenarios the response time is crucial.
Here, OMMs are able to provide a suitable trade-
off between accuracy and computational demands.
We showed that OMMs with few model states can
still reach competitive accuracy indices while consid-
erably decreasing computational demands to ensure
low latency capability. The combination of abstract-
ing and reproducing prototypical gesture trajectories,
the achievable response times, and the high recogni-
tion accuracy even for small training data sets makes
OMMs an ideal method for human computer interac-
tion.
In our ongoing research we focus on the auto-
matic optimization of classification in online use on
continuous interaction streams. Additionally, we are
working on a porting the gesture tracking system
to Microsofts Kinect
TM
. To further improve dis-
crimination performance in supervised setups, future
work in this context will include the use of Fisher
kernels (Jaakkola et al., 1999), which are straight-
forward to derive from OMMs.
ACKNOWLEDGEMENTS
This work is supported by the German Research
Foundation (DFG) in the Center of Excellence for
Cognitive Interaction Technology (CITEC). Thomas
Lingner has been funded by the PostDoc program of
the German Academic Exchange Service (DAAD).
REFERENCES
Amit, R. and Mataric, M. (2002). Learning movement se-
quences from demonstration. In ICDL ’02: Proceed-
ings of the 2nd International Conference on Develop-
ment and Learning, pages 203–208, Cambridge, Mas-
sachusetts. MIT Press.
Bergmann, K. and Kopp, S. (2009). Gnetic – using bayesian
decision networks for iconic gesture generation. In
Proceedings of the 9th Conference on Intelligent Vir-
tual Agents, pages 76–89. Springer.
ICPRAM 2012 - International Conference on Pattern Recognition Applications and Methods
160