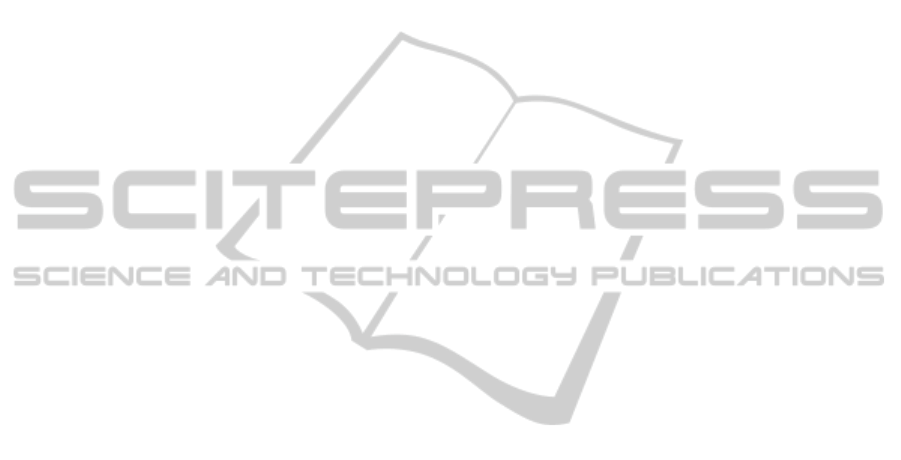
number of membership functions is not yet ideal and
should be explored further.
To evaluate the influence of the data tu-
ple/parameter ratio, we trained ANFIS 1 with the
data of 11 out of the 14 experiments, which equates
to a data/parameter ratio of 47,5 (see table 2). The
resulting mean average percentage error amounts to
Err
ap
= 81, 9%, which is significantly larger than for
the first training. Increasing the number of mem-
bership functions by one for one input would result
in a data/parameter ratio of 41,9. The improvement
gained by a higher input space resolution would be
negated by the low data/parameter ratio, therefore in-
creasing the number of membership functions was
postponed until further data is available.
To further improve the simulation results and to
examine the information content of the chosen acti-
vation variables, ANFIS 2 was trained. Its structure
remained the same as ANFIS 1, except for the input
space, where the parameter time was omitted.
This modification improved the net performance
significantly, leading to an average percentage error
of only Err
ap
= 1, 5% after three-fold cross valida-
tion. Evaluating the resulting linear functions re-
vealed however that this is achieved by modelling the
output as constant, with the superposition of all linear
functions taking approximately the form y = u
1
.
8 CONCLUSIONS
We designed and implemented a biofeedback system
as a game-like computer program to guide the user
into a predefined affective state. As a first step to-
wards the development of a model identification adap-
tive controller which will optimise the biofeedback
system with respect to time, we conducted a series
of experiments.
Our goal was the approximation of the system as
linear time-invariant models, allowing for the appli-
cation of well defined and efficient optimising tools
when designing the control algorithm and the adap-
tion laws. While being able to generate models with
average percentage errors below 2%, the examined
model structure did not yet reveal a correlation be-
tween the skin conductance and the controllable vari-
ables of the biofeedback game.
However, since the data/parameter ratio showed
to have a high influence on the net’s performance, we
conclude that for an exhaustive analysis of the influ-
ence of the input partitioning, more data is necessary.
With the higher granularity of the input space, the
generalisation requirement of the linear models is re-
duced. This could result in more accurate models bet-
ter suited to depict the correlation between skin con-
ductance and game parameters.
Further research is suggested to determine
whether an ANFIS can be trained to approximate the
system’s behaviour and to find a suitable foundation
to construct a MIAC in which the system is identified
online as a linear time-invariant model.
REFERENCES
Bosch, S., Marin-Perianu, M., Marin-Perianu, R., Havinga,
P., and Hermens, H. (2009). Keep on moving! activ-
ity monitoring and stimulation using wireless sensor
networks. In EuroSSC’09 Proceedings of the 4th Eu-
ropean conference on Smart sensing and context.
Chiari, L., Farella, E., Rocchi, L., and Benini, L. (2009). A
biofeedback based portable device to support elderly
mobility in the home environment. In World Congress
on Medical Physics and Biomedical Engineering.
Fairclough, S. H. (2009). Fundamentals of physiological
computing. Interacting with Computers, 21:133–145.
Isermann, R. (1987). Digitale Regelsysteme. Springer
Berlin Heidelberg.
Jang, J.-S., Sun, C.-T., and Mizutani, E. (1997). Neuro-
Fuzzy and Soft Computing - A Computational
Approach to Learning and Machine Intelligence.
Prentice-Hall.
Karray, F. O. and Silva, C. W. D. (2004). Soft computing
and intelligent systems design: theory, tools, and ap-
plications. Pearson Education.
Morris, M. (2006). Biofeedback revisited: Dynamic dis-
plays to improve health trajectories. In PERSUASIVE
2006.
Nacke, L. (2009). Affective Ludology: Scientific Measure-
ment of User Experience in Interactive Entertainment.
PhD thesis, Blekinge Institute of Technology, School
of Computing.
Oswald, W. D. (2007). Pr
¨
avention der demenz – das sima-
projekt. Public Health Forum, 15:28.
Pl
¨
otzsch, O. (2009). Bev
¨
olkerung Deutschlands bis 2060
- 12. koordinierte Bev
¨
olkerungsvorausberechnung.
Statistisches Bundesamt, Wiesbaden.
Rachuy, C., Budde, S., and Schill, K. (2011). Unobtrusive
data retrieval for providing individual assistance in aal
environments. In International Conference on Health
Informatics 2011.
Rief, W. and Birbaumer, N., editors (2006). Biofeedback
- Grundlagen, Indikatoren, Kommunikation, praktis-
ches Vorgehen in der Therapie. Schattauer GmbH.
Schr
¨
oder, D. (2010). Intelligente Verfahren: Identifikation
und Regelung nichtlinearer Systeme. Springer Berlin
Heidelberg.
SomaticVision, I. (2009). Somaticvision. abgerufen am
11.11.10.
Stern, R. M., Ray, W. J., and Quigley, K. S. (2001). Psy-
chophysiological Recording. Oxford University Press,
Inc.
WildDivine (2010). Wilddivine. abgerufen am 10.11.10.
HEALTHINF 2012 - International Conference on Health Informatics
212