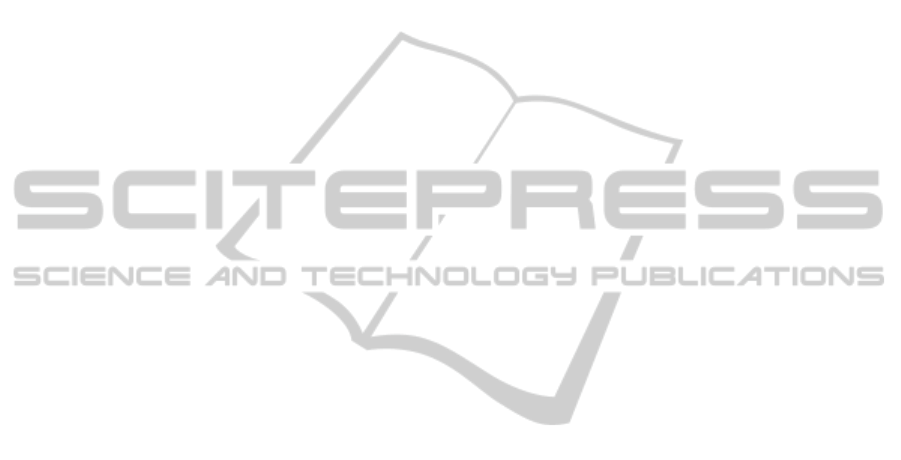
are more detailed food textures under it as shown in
Figure 3, from the knowledge of the labeled plot, the
food texture elasticity is mostly inside the soft part
and food texture crunchiness is mostly inside the hard
part.
5 CONCLUSIONS
Common ways for food texture measurement is to use
equipments that cannot be used for continuous eating
habits monitoring. However, it is very important to
monitoring eating habits by observing the food tex-
ture of foods people eat in daily life.
In this paper, the possibility of estimating food
texture in daily life monitoring is explored, and a
model of detailed food texture estimation using sound
for wearable eating habits monitoring is developed.
The proposed model’s efficiency has been validated,
showing an high accuracy and a good stability for dif-
ferent individuals and different food types with vari-
ety of food textures.
ACKNOWLEDGEMENTS
This research was supported by Japan Science and
Technology agency (JST)’s strategic sector for cre-
ation of advanced integrated sensing technologies for
realizing safe and secure societies: research project
on ”Development of a Physiological and Environmen-
tal Information Processing Platform and its Applica-
tion to the Metabolic Syndrome Measures”.
REFERENCES
Abraham, S., Collins, G., and Nordsieck, M. (1971). Re-
lationship of childhood weight status to morbidity in
adults. HSMHA Health Reports, Vol. 86, No. 3, pages
273–284.
Amft, O., Stger, M., Lukowicz, P., and Trster, G. (2005).
Analysis of chewing sounds for dietary monitoring. In
Proceedings of 7th International Conference on Ubiq-
uitous Computing. Springer.
Hoffmans, A. F., Kromhout, D., and de Lezenne Coulander,
C. (1989). Body mass index at the age of 18 and its ef-
fects on 32-year-mortality from coronary heart disease
and cancer. a nested case-control study among the en-
tire 1932 dutch male birth cohort. Journal of Clinical
Epidemiology, Vol. 6, Issue 6, pages 513–520.
Hung, H., Willet, W., Ascherio, A., Rosner, B. A., Rimm,
E., and Joshipura, K. J. (2003). Tooth loss and dietary
intake. Journal of the American Dental Association,
Vol. 134, No. 9, pages 1185–1192.
Kohonen, T. (1997). Self-Organizing Maps. Springer, Ger-
many, 2nd edition.
Kohyama, K., Mioche, L., and Bourdiol, P. (2003). In-
fluence of age and dental status on chewing behavior
studied by emg recordings during consumption of var-
ious food samples. Gerontology, Vol. 20, No. 1, pages
15–23.
Morlet, J. (1984). Decomposition of hardy functions into
square integrable wavelets of constant shape. SIAM.
Math. Anal., pages 723–736.
Nishimura, J. and Kuroda, T. (2008). Eating habits moni-
toring using wireless wearable in-ear microphone. In
International Symposium on Wireless Pervasive Com-
munications (ISWPC08). IEEE.
Oka, K., Sakuarae, A., Fujise, T., Yoshimatsu, H., Sakata,
T., and Nakata, M. (2003). Food texture differences
affect energy metabolism in rats. Journal of Dental
Research, vol. 82 No. 6, pages 491–494.
Peng, H., Long, F., and Ding, C. (2005). Feature selec-
tion based on mutual information: Criteria of max-
dependency, max-relevance, and min-redundancy.
IEEE Transactions on Pattern Analysis and Machine
Intelligence Vol. 27, No. 8, pages 1226–1238.
Shuzo, M., Komori, S., Takashima, T., Lopez, G., Tatsuta,
S., Yanagimoto, S., Warisawa, S., Delaunay, J.-J., and
Yamada, I. (2010). Wearable eating habit sensing sys-
tem using internal body sound. Journal of Advanced
Mechanical Design, Systems, and Manufacturing Vol.
4, Issue 1, pages 158–166.
Unler, A., Murat, A., and Chinnam, R. B. (2010). mr2pso:
A maximum relevance minimum redundancy feature
selection method based on swarm intelligence for sup-
port vector machine classification. Information Sci-
ences Vol. 181, Issue 20, pages 4625–4641.
Yoshikawa, S. and Okabe, M. (1978). Texture profile pat-
tern of foods by profile terms and texturometer (in
japanese). Report of National Food Research Insti-
tute, Vol. 33, pages 123–129.
Zhu, F. and Guan, S. (2004). Feature selection for modular
ga-based classification. Applied Soft Computing Vol.
4, Issue 4, pages 381–393.
HEALTHINF 2012 - International Conference on Health Informatics
218