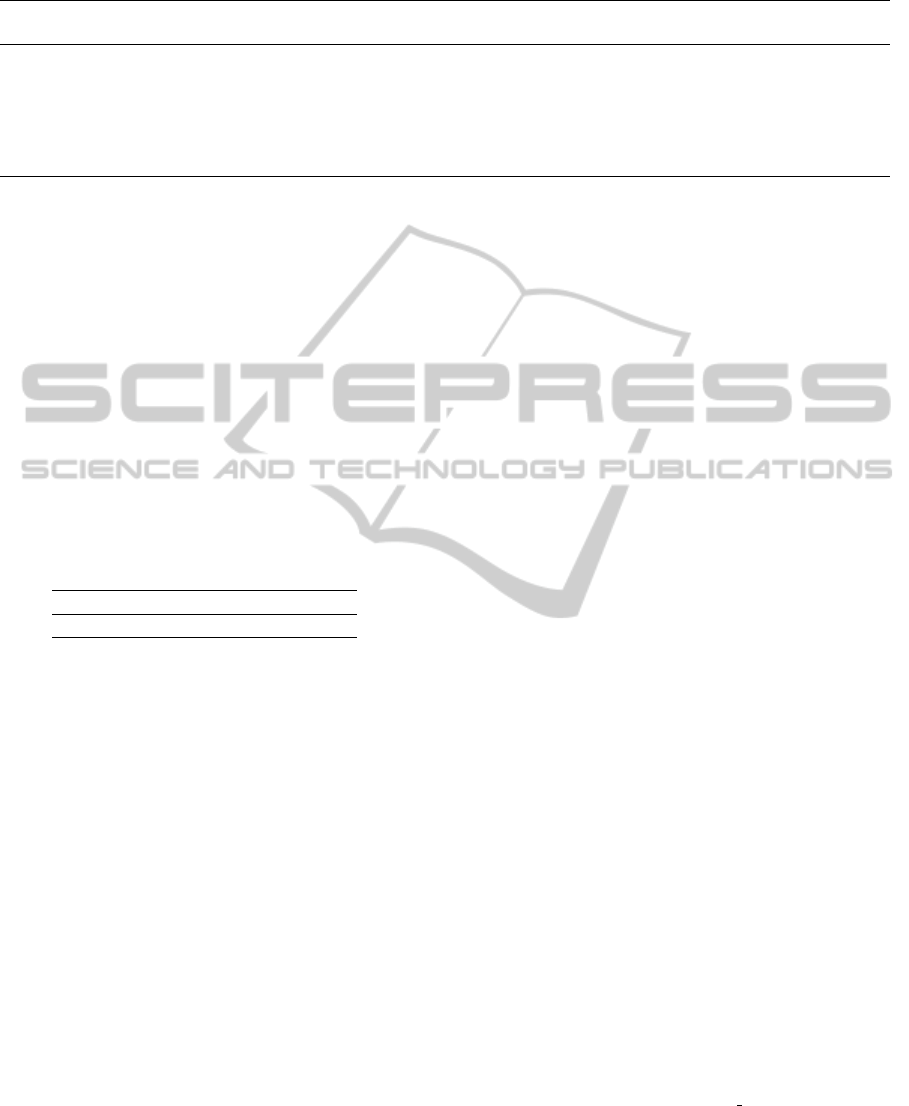
Table 1: Results of comparisons using 5 parameters evaluation.
Comparison Overall Lowest error rate Highest error rate Lowest error rate Highest error rate
situations No. error rate(%) for individual(%) for individual(%) for food type(%) for food type(%)
LE + LPF 35 7 57 16 (Salad) 61 (Fruit jelly)
ZCT + LPF 41 8 61 31 (Salad) 62 (Fruit jelly)
ADA + LPF 30 7 55 13 (Salad) 60 (Fruit jelly)
LE + LPF + rule 8 2 17 4 (Salad) 12 (Banana)
ZCT + LPF + rule 11 3 20 8 (Fruit jelly) 43 (Banana)
ADA + LPF + rule 7 3 14 2 (Salad) 11 (Marshmallow)
of 9 frames crosses zero with a negative value slope
and the local peak of low pass filter output is larger
than the sensitivity threshold.
6.2 Comparison Results
The overall accuracy of the mastication counting
models were compared (Table 2). The low pass fil-
ter based model with fixed cut-off frequency of 1.5
Hz was named as M1, the low pass filter based model
with adaptive cut-offfrequencywas named as M2, the
log energy based model was named as M3, and the
model proposed in section 5 was named as M.
Table 2: Comparison of overall accuracy for 4 models.
Methods M M1 M2 M3
Accuracy(%) 93 55 85 77
7 CONCLUSIONS
In this paper, we proposed a data analysis model for
realizing automatic mastication counting for any indi-
vidual and food type with high accuracy (93%). The
proposed model reduces the influence of individual
chewing ways differences (speed, strength, etc.) to a
large extent, and is little influenced by chewing sound
of different food types (soft, hard, crispy, etc.).
ACKNOWLEDGEMENTS
This research was supported by Japan Science and
Technology agency (JST)’s strategic sector for cre-
ation of advanced integrated sensing technologies for
realizing safe and secure societies: research project
on ”Development of a Physiological and Environmen-
tal Information Processing Platform and its Applica-
tion to the Metabolic Syndrome Measures”.
REFERENCES
Abraham, S., Collins, G., and Nordsieck, M. (1971). Re-
lationship of childhood weight status to morbidity in
adults. In HSMHA Health Reports. United States.
Health Services and Mental Health Administration.
Amft, O., Stger, M., and Trster, G. (2005). Analysis of
chewing sounds for dietary monitoring. In Proceed-
ings of 7th International Conference on Ubiquitous
Computing. Springer.
Hoffmans, A. F., Kromhout, D., and de Lezenne Coulande,
C. (1989). Body mass index at the age of 18 and its
effects on 32-year-mortality from coronary heart dis-
ease and cancer. a nnested case-control study among
the entire 1932 dutch male birth cohort. Journal of
Clinical Epidemiology Vol. 42, Issue 6, pages 513–
520.
Nicklas, T., Baranowski, T., Cullen, K., and Berenson, G.
(2001). Eating patterns, dietary quality and obesity.
Journal of the American College of Nutrition Vol. 20,
Issue 6, pages 599–608.
Nishimura, J. and Kuroda, T. (2008). Eating habits moni-
toring using wireless wearable in-ear microphone. In
Proceedings of International Symposium on Wireless
Pervasive Communications (ISWPC08). IEEE.
Shuzo, M., Komori, S., Takashima, T., Lopez, G., Tatsuta,
S., Yanagimoto, S., Warisawa, S., Delaunay, J.-J., and
Yamada, I. (2010). Wearable eating habit sensing sys-
tem using internal body sound. Journal of Advanced
Mechanical Design, Systems, and Manufacturing Vol.
4, Issue 1, pages 158–166.
Yasutomi, K. and Masuda, Y. (2008). Example of food
education for young japanese obese by wearing the
’kamikami sensor’ while eating. Journal of Japanese
Society for Masticatory Science and Health Promo-
tion Vol. 18, Issue 2, pages 149–150.
Notes
1
http://www.mando.se/en/start-page/start-page-/1.aspx
2
http://en.wikipedia.org/wiki/Zero crossing
3
http://www.mathworks.com/help/toolbox/signal/
findpeaks.html
MASTICATION COUNTING METHOD ROBUST TO FOOD TYPE AND INDIVIDUAL
377